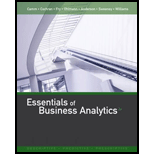
(a)
Draw the time-series plot for the given data.
Identify the pattern.
(a)

Explanation of Solution
Step-by-step procedure to construct time-series plot is given below.
- Enter the data in columns A and B. Select the data.
- Click on Insert tab and then click on line.
- Select line with markers
The output is given below:
From the above time-series plot, it is clear that plot shows upward trend. Also, there exists seasonal pattern.
(b)
Find a multiple regression equation that represents seasonal effect using dummy variables for the given data.
(b)

Answer to Problem 25P
The regression equation is,
Explanation of Solution
Dummy variables are defined as given below:
Also, all the dummy variables are 0 when the reading time corresponds to 5:00 p.m. to 6:00 p.m.
The given data is entered as given below:
Hourly Dummy Variables | |||||||||||||
Date | Hour | yt | 1 | 2 | 3 | 4 | 5 | 6 | 7 | 8 | 9 | 10 | 11 |
July 15 | 6:00 a.m. - 7:00 a.m. | 25 | 1 | 0 | 0 | 0 | 0 | 0 | 0 | 0 | 0 | 0 | 0 |
July 15 | 7:00 a.m. - 8:00 a.m. | 28 | 0 | 1 | 0 | 0 | 0 | 0 | 0 | 0 | 0 | 0 | 0 |
July 15 | 8:00 a.m. - 9:00 a.m. | 35 | 0 | 0 | 1 | 0 | 0 | 0 | 0 | 0 | 0 | 0 | 0 |
July 15 | 9:00 a.m. - 10:00 a.m. | 50 | 0 | 0 | 0 | 1 | 0 | 0 | 0 | 0 | 0 | 0 | 0 |
July 15 | 10:00 a.m. - 11:00 a.m. | 60 | 0 | 0 | 0 | 0 | 1 | 0 | 0 | 0 | 0 | 0 | 0 |
July 15 | 11:00 a.m. - 12:00 p.m. | 60 | 0 | 0 | 0 | 0 | 0 | 1 | 0 | 0 | 0 | 0 | 0 |
July 15 | 12:00 p.m. - 1:00 p.m. | 40 | 0 | 0 | 0 | 0 | 0 | 0 | 1 | 0 | 0 | 0 | 0 |
July 15 | 1:00 p.m. - 2:00 p.m. | 35 | 0 | 0 | 0 | 0 | 0 | 0 | 0 | 1 | 0 | 0 | 0 |
July 15 | 2:00 p.m. - 3:00 p.m. | 30 | 0 | 0 | 0 | 0 | 0 | 0 | 0 | 0 | 1 | 0 | 0 |
July 15 | 3:00 p.m. - 4:00 p.m. | 25 | 0 | 0 | 0 | 0 | 0 | 0 | 0 | 0 | 0 | 1 | 0 |
July 15 | 4:00 p.m. - 5:00 p.m. | 25 | 0 | 0 | 0 | 0 | 0 | 0 | 0 | 0 | 0 | 0 | 1 |
July 15 | 5:00 p.m. - 6:00 p.m. | 20 | 0 | 0 | 0 | 0 | 0 | 0 | 0 | 0 | 0 | 0 | 0 |
July 16 | 6:00 a.m. - 7:00 a.m. | 28 | 1 | 0 | 0 | 0 | 0 | 0 | 0 | 0 | 0 | 0 | 0 |
July 16 | 7:00 a.m. - 8:00 a.m. | 30 | 0 | 1 | 0 | 0 | 0 | 0 | 0 | 0 | 0 | 0 | 0 |
July 16 | 8:00 a.m. - 9:00 a.m. | 35 | 0 | 0 | 1 | 0 | 0 | 0 | 0 | 0 | 0 | 0 | 0 |
July 16 | 9:00 a.m. - 10:00 a.m. | 48 | 0 | 0 | 0 | 1 | 0 | 0 | 0 | 0 | 0 | 0 | 0 |
July 16 | 10:00 a.m. - 11:00 a.m. | 60 | 0 | 0 | 0 | 0 | 1 | 0 | 0 | 0 | 0 | 0 | 0 |
July 16 | 11:00 a.m. - 12:00 p.m. | 65 | 0 | 0 | 0 | 0 | 0 | 1 | 0 | 0 | 0 | 0 | 0 |
July 16 | 12:00 p.m. - 1:00 p.m. | 50 | 0 | 0 | 0 | 0 | 0 | 0 | 1 | 0 | 0 | 0 | 0 |
July 16 | 1:00 p.m. - 2:00 p.m. | 40 | 0 | 0 | 0 | 0 | 0 | 0 | 0 | 1 | 0 | 0 | 0 |
July 16 | 2:00 p.m. - 3:00 p.m. | 35 | 0 | 0 | 0 | 0 | 0 | 0 | 0 | 0 | 1 | 0 | 0 |
July 16 | 3:00 p.m. - 4:00 p.m. | 25 | 0 | 0 | 0 | 0 | 0 | 0 | 0 | 0 | 0 | 1 | 0 |
July 16 | 4:00 p.m. - 5:00 p.m. | 20 | 0 | 0 | 0 | 0 | 0 | 0 | 0 | 0 | 0 | 0 | 1 |
July 16 | 5:00 p.m. - 6:00 p.m. | 20 | 0 | 0 | 0 | 0 | 0 | 0 | 0 | 0 | 0 | 0 | 0 |
July 17 | 6:00 a.m. - 7:00 a.m. | 35 | 1 | 0 | 0 | 0 | 0 | 0 | 0 | 0 | 0 | 0 | 0 |
July 17 | 7:00 a.m. - 8:00 a.m. | 42 | 0 | 1 | 0 | 0 | 0 | 0 | 0 | 0 | 0 | 0 | 0 |
July 17 | 8:00 a.m. - 9:00 a.m. | 45 | 0 | 0 | 1 | 0 | 0 | 0 | 0 | 0 | 0 | 0 | 0 |
July 17 | 9:00 a.m. - 10:00 a.m. | 70 | 0 | 0 | 0 | 1 | 0 | 0 | 0 | 0 | 0 | 0 | 0 |
July 17 | 10:00 a.m. - 11:00 a.m. | 72 | 0 | 0 | 0 | 0 | 1 | 0 | 0 | 0 | 0 | 0 | 0 |
July 17 | 11:00 a.m. - 12:00 p.m. | 75 | 0 | 0 | 0 | 0 | 0 | 1 | 0 | 0 | 0 | 0 | 0 |
July 17 | 12:00 p.m. - 1:00 p.m. | 60 | 0 | 0 | 0 | 0 | 0 | 0 | 1 | 0 | 0 | 0 | 0 |
July 17 | 1:00 p.m. - 2:00 p.m. | 45 | 0 | 0 | 0 | 0 | 0 | 0 | 0 | 1 | 0 | 0 | 0 |
July 17 | 2:00 p.m. - 3:00 p.m. | 40 | 0 | 0 | 0 | 0 | 0 | 0 | 0 | 0 | 1 | 0 | 0 |
July 17 | 3:00 p.m. - 4:00 p.m. | 25 | 0 | 0 | 0 | 0 | 0 | 0 | 0 | 0 | 0 | 1 | 0 |
July 17 | 4:00 p.m. - 5:00 p.m. | 25 | 0 | 0 | 0 | 0 | 0 | 0 | 0 | 0 | 0 | 0 | 1 |
July 17 | 5:00 p.m. - 6:00 p.m. | 25 | 0 | 0 | 0 | 0 | 0 | 0 | 0 | 0 | 0 | 0 | 0 |
Step-by-step procedure to obtain multiple linear regression line is given below.
- Enter the data in columns A to M.
- Click on Data tab and then Data Analysis.
- Select Regression and click ok.
- In Input Y
Range select, $B$2:$B$37 and Input X Range select $C$2:$M$37 - Click Ok.
The output is given below:
From the output the regression equation is,
Here, X Variable 1 represents Hour1, X Variable 2 represents Hour2, … X variable 11 represents Hour11.
(c)
Find the estimates of the levels of nitrogen for July 18 using the model developed in part (b).
(c)

Explanation of Solution
From part (b), the regression equation is,
Forecast for July 18 is obtained as given below:
Hourly forecast | Calculation | |
Hour1 | 29.34 | |
Hour2 | 33.34 | |
Hour3 | 38.34 | |
Hour4 | 56 | |
Hour5 | 64 | |
Hour6 | 66.67 | |
Hour7 | 50 | |
Hour8 | 40 | |
Hour9 | 35 | |
Hour10 | 25 | |
Hour11 | 23.34 | |
Hour12 | 21.67 | 21.67 |
(d)
Construct a multiple regression equation that represents seasonal effect using dummy variables and a t variable for the given data.
(d)

Answer to Problem 25P
The regression equation is,
Explanation of Solution
Create a variable t such that t = 1 for hour 1 on July 15, t = 2 for hour 2 on July 2, …, t = 36 for hour 12 on July 18.
The given data is entered as given below:
Hourly Dummy Variables | ||||||||||||||
Date | Hour | yt | 1 | 2 | 3 | 4 | 5 | 6 | 7 | 8 | 9 | 10 | 11 | t |
July 15 | 6:00 a.m. - 7:00 a.m. | 25 | 1 | 0 | 0 | 0 | 0 | 0 | 0 | 0 | 0 | 0 | 0 | 1 |
July 15 | 7:00 a.m. - 8:00 a.m. | 28 | 0 | 1 | 0 | 0 | 0 | 0 | 0 | 0 | 0 | 0 | 0 | 2 |
July 15 | 8:00 a.m. - 9:00 a.m. | 35 | 0 | 0 | 1 | 0 | 0 | 0 | 0 | 0 | 0 | 0 | 0 | 3 |
July 15 | 9:00 a.m. - 10:00 a.m. | 50 | 0 | 0 | 0 | 1 | 0 | 0 | 0 | 0 | 0 | 0 | 0 | 4 |
July 15 | 10:00 a.m. - 11:00 a.m. | 60 | 0 | 0 | 0 | 0 | 1 | 0 | 0 | 0 | 0 | 0 | 0 | 5 |
July 15 | 11:00 a.m. - 12:00 p.m. | 60 | 0 | 0 | 0 | 0 | 0 | 1 | 0 | 0 | 0 | 0 | 0 | 6 |
July 15 | 12:00 p.m. - 1:00 p.m. | 40 | 0 | 0 | 0 | 0 | 0 | 0 | 1 | 0 | 0 | 0 | 0 | 7 |
July 15 | 1:00 p.m. - 2:00 p.m. | 35 | 0 | 0 | 0 | 0 | 0 | 0 | 0 | 1 | 0 | 0 | 0 | 8 |
July 15 | 2:00 p.m. - 3:00 p.m. | 30 | 0 | 0 | 0 | 0 | 0 | 0 | 0 | 0 | 1 | 0 | 0 | 9 |
July 15 | 3:00 p.m. - 4:00 p.m. | 25 | 0 | 0 | 0 | 0 | 0 | 0 | 0 | 0 | 0 | 1 | 0 | 10 |
July 15 | 4:00 p.m. - 5:00 p.m. | 25 | 0 | 0 | 0 | 0 | 0 | 0 | 0 | 0 | 0 | 0 | 1 | 11 |
July 15 | 5:00 p.m. - 6:00 p.m. | 20 | 0 | 0 | 0 | 0 | 0 | 0 | 0 | 0 | 0 | 0 | 0 | 12 |
July 16 | 6:00 a.m. - 7:00 a.m. | 28 | 1 | 0 | 0 | 0 | 0 | 0 | 0 | 0 | 0 | 0 | 0 | 13 |
July 16 | 7:00 a.m. - 8:00 a.m. | 30 | 0 | 1 | 0 | 0 | 0 | 0 | 0 | 0 | 0 | 0 | 0 | 14 |
July 16 | 8:00 a.m. - 9:00 a.m. | 35 | 0 | 0 | 1 | 0 | 0 | 0 | 0 | 0 | 0 | 0 | 0 | 15 |
July 16 | 9:00 a.m. - 10:00 a.m. | 48 | 0 | 0 | 0 | 1 | 0 | 0 | 0 | 0 | 0 | 0 | 0 | 16 |
July 16 | 10:00 a.m. - 11:00 a.m. | 60 | 0 | 0 | 0 | 0 | 1 | 0 | 0 | 0 | 0 | 0 | 0 | 17 |
July 16 | 11:00 a.m. - 12:00 p.m. | 65 | 0 | 0 | 0 | 0 | 0 | 1 | 0 | 0 | 0 | 0 | 0 | 18 |
July 16 | 12:00 p.m. - 1:00 p.m. | 50 | 0 | 0 | 0 | 0 | 0 | 0 | 1 | 0 | 0 | 0 | 0 | 19 |
July 16 | 1:00 p.m. - 2:00 p.m. | 40 | 0 | 0 | 0 | 0 | 0 | 0 | 0 | 1 | 0 | 0 | 0 | 20 |
July 16 | 2:00 p.m. - 3:00 p.m. | 35 | 0 | 0 | 0 | 0 | 0 | 0 | 0 | 0 | 1 | 0 | 0 | 21 |
July 16 | 3:00 p.m. - 4:00 p.m. | 25 | 0 | 0 | 0 | 0 | 0 | 0 | 0 | 0 | 0 | 1 | 0 | 22 |
July 16 | 4:00 p.m. - 5:00 p.m. | 20 | 0 | 0 | 0 | 0 | 0 | 0 | 0 | 0 | 0 | 0 | 1 | 23 |
July 16 | 5:00 p.m. - 6:00 p.m. | 20 | 0 | 0 | 0 | 0 | 0 | 0 | 0 | 0 | 0 | 0 | 0 | 24 |
July 17 | 6:00 a.m. - 7:00 a.m. | 35 | 1 | 0 | 0 | 0 | 0 | 0 | 0 | 0 | 0 | 0 | 0 | 25 |
July 17 | 7:00 a.m. - 8:00 a.m. | 42 | 0 | 1 | 0 | 0 | 0 | 0 | 0 | 0 | 0 | 0 | 0 | 26 |
July 17 | 8:00 a.m. - 9:00 a.m. | 45 | 0 | 0 | 1 | 0 | 0 | 0 | 0 | 0 | 0 | 0 | 0 | 27 |
July 17 | 9:00 a.m. - 10:00 a.m. | 70 | 0 | 0 | 0 | 1 | 0 | 0 | 0 | 0 | 0 | 0 | 0 | 28 |
July 17 | 10:00 a.m. - 11:00 a.m. | 72 | 0 | 0 | 0 | 0 | 1 | 0 | 0 | 0 | 0 | 0 | 0 | 29 |
July 17 | 11:00 a.m. - 12:00 p.m. | 75 | 0 | 0 | 0 | 0 | 0 | 1 | 0 | 0 | 0 | 0 | 0 | 30 |
July 17 | 12:00 p.m. - 1:00 p.m. | 60 | 0 | 0 | 0 | 0 | 0 | 0 | 1 | 0 | 0 | 0 | 0 | 31 |
July 17 | 1:00 p.m. - 2:00 p.m. | 45 | 0 | 0 | 0 | 0 | 0 | 0 | 0 | 1 | 0 | 0 | 0 | 32 |
July 17 | 2:00 p.m. - 3:00 p.m. | 40 | 0 | 0 | 0 | 0 | 0 | 0 | 0 | 0 | 1 | 0 | 0 | 33 |
July 17 | 3:00 p.m. - 4:00 p.m. | 25 | 0 | 0 | 0 | 0 | 0 | 0 | 0 | 0 | 0 | 1 | 0 | 34 |
July 17 | 4:00 p.m. - 5:00 p.m. | 25 | 0 | 0 | 0 | 0 | 0 | 0 | 0 | 0 | 0 | 0 | 1 | 35 |
July 17 | 5:00 p.m. - 6:00 p.m. | 25 | 0 | 0 | 0 | 0 | 0 | 0 | 0 | 0 | 0 | 0 | 0 | 36 |
Step-by-step procedure to obtain multiple linear regression line is given below.
- Enter the data in columns A to N.
- Click on Data tab and then Data Analysis.
- Select Regression and click ok.
- In Input Y Range select, $B$2:$B$37 and Input X Range select $C$2:$N$37
- Click Ok.
The output is given below:
From the output the regression equation is,
Here, X Variable 1 represents Hour1, X Variable 2 represents Hour2,… X variable 11 represents Hour11 and X variable 12 represents t.
(e)
Calculate the estimates of the levels of nitrogen for July 18 using the model developed in part (d).
(e)

Explanation of Solution
From part (d), the regression equation is,
Forecast for July 18 is given below:
Hourly forecast | T | Calculation | |
1 | 37 | 39.93 | |
2 | 38 | 43.93 | |
3 | 39 | 48.93 | |
4 | 40 | 66.6 | |
5 | 41 | 74.71 | |
6 | 42 | 77.28 | |
7 | 43 | 60.61 | |
8 | 44 | 50.61 | |
9 | 45 | 45.62 | |
10 | 46 | 35.62 | |
11 | 47 | 33.95 | |
12 | 48 | 32.29 |
(f)
Justify which of the models (b) or (d) is effective.
(f)

Answer to Problem 25P
Model (d) is preferred.
Explanation of Solution
For the multiple regression equation developed in part (b), MSE is obtained as given below:
Date | Hour | yt | Forecast | Forecast Error | Squared Forecast Error |
15-Jul | 6:00 a.m. - 7:00 a.m. | 25 | 29.34 | -4.34 | 18.8356 |
15-Jul | 7:00 a.m. - 8:00 a.m. | 28 | 33.34 | -5.34 | 28.5156 |
15-Jul | 8:00 a.m. - 9:00 a.m. | 35 | 38.34 | -3.34 | 11.1556 |
15-Jul | 9:00 a.m. - 10:00 a.m. | 50 | 56 | -6 | 36 |
15-Jul | 10:00 a.m. - 11:00 a.m. | 60 | 64 | -4 | 16 |
15-Jul | 11:00 a.m. - 12:00 p.m. | 60 | 66.67 | -6.67 | 44.4889 |
15-Jul | 12:00 p.m. - 1:00 p.m. | 40 | 50 | -10 | 100 |
15-Jul | 1:00 p.m. - 2:00 p.m. | 35 | 40 | -5 | 25 |
15-Jul | 2:00 p.m. - 3:00 p.m. | 30 | 35 | -5 | 25 |
15-Jul | 3:00 p.m. - 4:00 p.m. | 25 | 25 | 0 | 0 |
15-Jul | 4:00 p.m. - 5:00 p.m. | 25 | 23.34 | 1.66 | 2.7556 |
15-Jul | 5:00 p.m. - 6:00 p.m. | 20 | 21.67 | -1.67 | 2.7889 |
16-Jul | 6:00 a.m. - 7:00 a.m. | 28 | 29.34 | -1.34 | 1.7956 |
16-Jul | 7:00 a.m. - 8:00 a.m. | 30 | 33.34 | -3.34 | 11.1556 |
16-Jul | 8:00 a.m. - 9:00 a.m. | 35 | 38.34 | -3.34 | 11.1556 |
16-Jul | 9:00 a.m. - 10:00 a.m. | 48 | 56 | -8 | 64 |
16-Jul | 10:00 a.m. - 11:00 a.m. | 60 | 64 | -4 | 16 |
16-Jul | 11:00 a.m. - 12:00 p.m. | 65 | 66.67 | -1.67 | 2.7889 |
16-Jul | 12:00 p.m. - 1:00 p.m. | 50 | 50 | 0 | 0 |
16-Jul | 1:00 p.m. - 2:00 p.m. | 40 | 40 | 0 | 0 |
16-Jul | 2:00 p.m. - 3:00 p.m. | 35 | 35 | 0 | 0 |
16-Jul | 3:00 p.m. - 4:00 p.m. | 25 | 25 | 0 | 0 |
16-Jul | 4:00 p.m. - 5:00 p.m. | 20 | 23.34 | -3.34 | 11.1556 |
16-Jul | 5:00 p.m. - 6:00 p.m. | 20 | 21.67 | -1.67 | 2.7889 |
17-Jul | 6:00 a.m. - 7:00 a.m. | 35 | 29.34 | 5.66 | 32.0356 |
17-Jul | 7:00 a.m. - 8:00 a.m. | 42 | 33.34 | 8.66 | 74.9956 |
17-Jul | 8:00 a.m. - 9:00 a.m. | 45 | 38.34 | 6.66 | 44.3556 |
17-Jul | 9:00 a.m. - 10:00 a.m. | 70 | 56 | 14 | 196 |
17-Jul | 10:00 a.m. - 11:00 a.m. | 72 | 64 | 8 | 64 |
17-Jul | 11:00 a.m. - 12:00 p.m. | 75 | 66.67 | 8.33 | 69.3889 |
17-Jul | 12:00 p.m. - 1:00 p.m. | 60 | 50 | 10 | 100 |
17-Jul | 1:00 p.m. - 2:00 p.m. | 45 | 40 | 5 | 25 |
17-Jul | 2:00 p.m. - 3:00 p.m. | 40 | 35 | 5 | 25 |
17-Jul | 3:00 p.m. - 4:00 p.m. | 25 | 25 | 0 | 0 |
17-Jul | 4:00 p.m. - 5:00 p.m. | 25 | 23.34 | 1.66 | 2.7556 |
17-Jul | 5:00 p.m. - 6:00 p.m. | 25 | 21.67 | 3.33 | 11.0889 |
1076.001 |
For the multiple regression equation developed in part (d), MSE is obtained as given below:
Date | Hour | t | yt | Forecast | Forecast Error | Squared Forecast Error |
15-Jul | 6:00 a.m. - 7:00 a.m. | 1 | 25 | 24.09 | 0.91 | 0.8281 |
15-Jul | 7:00 a.m. - 8:00 a.m. | 2 | 28 | 28.09 | -0.09 | 0.0081 |
15-Jul | 8:00 a.m. - 9:00 a.m. | 3 | 35 | 33.09 | 1.91 | 3.6481 |
15-Jul | 9:00 a.m. - 10:00 a.m. | 4 | 50 | 50.76 | -0.76 | 0.5776 |
15-Jul | 10:00 a.m. - 11:00 a.m. | 5 | 60 | 58.87 | 1.13 | 1.2769 |
15-Jul | 11:00 a.m. - 12:00 p.m. | 6 | 60 | 61.44 | -1.44 | 2.0736 |
15-Jul | 12:00 p.m. - 1:00 p.m. | 7 | 40 | 44.77 | -4.77 | 22.7529 |
15-Jul | 1:00 p.m. - 2:00 p.m. | 8 | 35 | 34.77 | 0.23 | 0.0529 |
15-Jul | 2:00 p.m. - 3:00 p.m. | 9 | 30 | 29.78 | 0.22 | 0.0484 |
15-Jul | 3:00 p.m. - 4:00 p.m. | 10 | 25 | 19.78 | 5.22 | 27.2484 |
15-Jul | 4:00 p.m. - 5:00 p.m. | 11 | 25 | 18.11 | 6.89 | 47.4721 |
15-Jul | 5:00 p.m. - 6:00 p.m. | 12 | 20 | 16.45 | 3.55 | 12.6025 |
16-Jul | 6:00 a.m. - 7:00 a.m. | 13 | 28 | 29.37 | -1.37 | 1.8769 |
16-Jul | 7:00 a.m. - 8:00 a.m. | 14 | 30 | 33.37 | -3.37 | 11.3569 |
16-Jul | 8:00 a.m. - 9:00 a.m. | 15 | 35 | 38.37 | -3.37 | 11.3569 |
16-Jul | 9:00 a.m. - 10:00 a.m. | 16 | 48 | 56.04 | -8.04 | 64.6416 |
16-Jul | 10:00 a.m. - 11:00 a.m. | 17 | 60 | 64.15 | -4.15 | 17.2225 |
16-Jul | 11:00 a.m. - 12:00 p.m. | 18 | 65 | 66.72 | -1.72 | 2.9584 |
16-Jul | 12:00 p.m. - 1:00 p.m. | 19 | 50 | 50.05 | -0.05 | 0.0025 |
16-Jul | 1:00 p.m. - 2:00 p.m. | 20 | 40 | 40.05 | -0.05 | 0.0025 |
16-Jul | 2:00 p.m. - 3:00 p.m. | 21 | 35 | 35.06 | -0.06 | 0.0036 |
16-Jul | 3:00 p.m. - 4:00 p.m. | 22 | 25 | 25.06 | -0.06 | 0.0036 |
16-Jul | 4:00 p.m. - 5:00 p.m. | 23 | 20 | 23.39 | -3.39 | 11.4921 |
16-Jul | 5:00 p.m. - 6:00 p.m. | 24 | 20 | 21.73 | -1.73 | 2.9929 |
17-Jul | 6:00 a.m. - 7:00 a.m. | 25 | 35 | 34.65 | 0.35 | 0.1225 |
17-Jul | 7:00 a.m. - 8:00 a.m. | 26 | 42 | 38.65 | 3.35 | 11.2225 |
17-Jul | 8:00 a.m. - 9:00 a.m. | 27 | 45 | 43.65 | 1.35 | 1.8225 |
17-Jul | 9:00 a.m. - 10:00 a.m. | 28 | 70 | 61.32 | 8.68 | 75.3424 |
17-Jul | 10:00 a.m. - 11:00 a.m. | 29 | 72 | 69.43 | 2.57 | 6.6049 |
17-Jul | 11:00 a.m. - 12:00 p.m. | 30 | 75 | 72 | 3 | 9 |
17-Jul | 12:00 p.m. - 1:00 p.m. | 31 | 60 | 55.33 | 4.67 | 21.8089 |
17-Jul | 1:00 p.m. - 2:00 p.m. | 32 | 45 | 45.33 | -0.33 | 0.1089 |
17-Jul | 2:00 p.m. - 3:00 p.m. | 33 | 40 | 40.34 | -0.34 | 0.1156 |
17-Jul | 3:00 p.m. - 4:00 p.m. | 34 | 25 | 30.34 | -5.34 | 28.5156 |
17-Jul | 4:00 p.m. - 5:00 p.m. | 35 | 25 | 28.67 | -3.67 | 13.4689 |
17-Jul | 5:00 p.m. - 6:00 p.m. | 36 | 25 | 27.01 | -2.01 | 4.0401 |
414.6728 |
MSE for model in (d) is smaller than MSE for the model in (b). Thus, model (d) is preferred.
Want to see more full solutions like this?
Chapter 8 Solutions
Essentials of Business Analytics (MindTap Course List)
- explain the importance of the Hypothesis test in a business setting, and give an example of a situation where it is helpful in business decision making.arrow_forwardA college wants to estimate what students typically spend on textbooks. A report fromthe college bookstore observes that textbooks range in price from $22 to $186. Toobtain a 95% confidence level for a confidence interval estimate to plus or minus $10,how many students should the college survey? (We may estimate the populationstandard deviation as (range) ÷ 4.)arrow_forwardIn a study of how students give directions, forty volunteers were given the task ofexplaining to another person how to reach a destination. Researchers measured thefollowing five aspects of the subjects’ direction-giving behavior:• whether a map was available or if directions were given from memory without a map,• the gender of the direction-giver,• the distances given as part of the directions,• the number of times directions such as “north” or “left” were used,• the frequency of errors in directions. Identify each of the variables in this study, and whether each is quantitative orqualitative. For each quantitative variable, state whether it is discrete or continuous. Was this an observational study or an experimental study? Explain your answer.arrow_forward
- explain the difference between the confident interval and the confident level. provide an example to show how to correctly interpret a confidence interval.arrow_forwardSketch to scale the orbit of Earth about the sun. Graph Icarus’ orbit on the same set of axesWhile the sun is the center of Earth’s orbit, it is a focus of Icarus’ orbit. There aretwo points of intersection on the graph. Based on the graph, what is the approximate distance between the two points of intersection (in AU)?arrow_forwardThe diameters of ball bearings are distributed normally. The mean diameter is 67 millimeters and the standard deviation is 3 millimeters. Find the probability that the diameter of a selected bearing is greater than 63 millimeters. Round to four decimal places.arrow_forward
- Suppose you like to keep a jar of change on your desk. Currently, the jar contains the following: 22 Pennies 27 Dimes 9 Nickels 30 Quarters What is the probability that you reach into the jar and randomly grab a penny and then, without replacement, a dime? Express as a fraction or a decimal number rounded to four decimal places.arrow_forwardA box contains 14 large marbles and 10 small marbles. Each marble is either green or white. 9 of the large marbles are green, and 4 of the small marbles are white. If a marble is randomly selected from the box, what is the probability that it is small or white? Express as a fraction or a decimal number rounded to four decimal places.arrow_forwardCan I get help with this step please? At a shooting range, instructors can determine if a shooter is consistently missing the target because of the gun sight or because of the shooter's ability. If a gun's sight is off, the variance of the distances between the shots and the center of the shot pattern will be small (even if the shots are not in the center of the target). A student claims that it is the sight that is off, not his aim, and wants the instructor to confirm his claim. If a skilled shooter fires a gun at a target multiple times, the distances between the shots and the center of the shot pattern, measured in centimeters (cm), will have a variance of less than 0.33. After the student shoots 28 shots at the target, the instructor calculates that the distances between his shots and the center of the shot pattern, measured in cm, have a variance of 0.25. Does this evidence support the student's claim that the gun's sight is off? Use a 0.025 level of significance. Assume that the…arrow_forward
- The National Academy of Science reported that 38% of research in mathematics is published by US authors. The mathematics chairperson of a prestigious university wishes to test the claim that this percentage is no longer 38%. He has no indication of whether the percentage has increased or decreased since that time. He surveys a simple random sample of 279 recent articles published by reputable mathematics research journals and finds that 123 of these articles have US authors. Does this evidence support the mathematics chairperson's claim that the percentage is no longer 38 % ? Use a 0.02 level of significance. Compute the value of the test statistic. Round to two decimal places.arrow_forwardA marketing research company desires to know the mean consumption of milk per week among males over age 32. They believe that the milk consumption has a mean of 4 liters, and want to construct a 98% confidence interval with a maximum error of 0.07 liters. Assuming a variance of 0.64 liters, what is the minimum number of males over age 32 they must include in their sample? Round up to the next integer.arrow_forwardSuppose GRE Verbal scores are normally distributed with a mean of 461 and a standard deviation of 118. A university plans to recruit students whose scores are in the top 4 % . What is the minimum score required for recruitment? Round to the nearest whole number, if necessaryarrow_forward
- Glencoe Algebra 1, Student Edition, 9780079039897...AlgebraISBN:9780079039897Author:CarterPublisher:McGraw HillHolt Mcdougal Larson Pre-algebra: Student Edition...AlgebraISBN:9780547587776Author:HOLT MCDOUGALPublisher:HOLT MCDOUGALTrigonometry (MindTap Course List)TrigonometryISBN:9781305652224Author:Charles P. McKeague, Mark D. TurnerPublisher:Cengage Learning
- Intermediate AlgebraAlgebraISBN:9781285195728Author:Jerome E. Kaufmann, Karen L. SchwittersPublisher:Cengage LearningBig Ideas Math A Bridge To Success Algebra 1: Stu...AlgebraISBN:9781680331141Author:HOUGHTON MIFFLIN HARCOURTPublisher:Houghton Mifflin HarcourtAlgebra for College StudentsAlgebraISBN:9781285195780Author:Jerome E. Kaufmann, Karen L. SchwittersPublisher:Cengage Learning
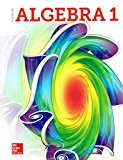
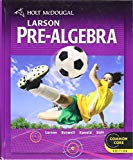
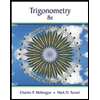
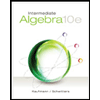
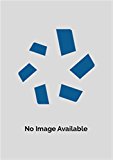
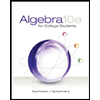