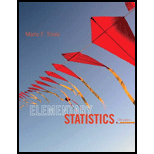
Concept explainers
Confidence Intervals for β0 and β1 Confidence intervals for the y-intercept β0 and slope β1, for a regression line (y = β0 + β1x) can be found by evaluating the limits in the intervals below.
where
where
The y-intercept b0 and the slope b1 are found from the sample data and tα/2 is found from Table A-3 by using n − 2 degrees of freedom. Using the 40 pairs of shoe print lengths (x) and heights (y) from Data Set 2 in Appendix B, find the 95% confidence

Want to see the full answer?
Check out a sample textbook solution
Chapter 10 Solutions
Elementary Statistics
- Olympic Pole Vault The graph in Figure 7 indicates that in recent years the winning Olympic men’s pole vault height has fallen below the value predicted by the regression line in Example 2. This might have occurred because when the pole vault was a new event there was much room for improvement in vaulters’ performances, whereas now even the best training can produce only incremental advances. Let’s see whether concentrating on more recent results gives a better predictor of future records. (a) Use the data in Table 2 (page 176) to complete the table of winning pole vault heights shown in the margin. (Note that we are using x=0 to correspond to the year 1972, where this restricted data set begins.) (b) Find the regression line for the data in part ‚(a). (c) Plot the data and the regression line on the same axes. Does the regression line seem to provide a good model for the data? (d) What does the regression line predict as the winning pole vault height for the 2012 Olympics? Compare this predicted value to the actual 2012 winning height of 5.97 m, as described on page 177. Has this new regression line provided a better prediction than the line in Example 2?arrow_forwardFind the equation of the regression line for the following data set. x 1 2 3 y 0 3 4arrow_forwardTable 6 shows the population, in thousands, of harbor seals in the Wadden Sea over the years 1997 to 2012. a. Let x represent time in years starting with x=0 for the year 1997. Let y represent the number of seals in thousands. Use logistic regression to fit a model to these data. b. Use the model to predict the seal population for the year 2020. c. To the nearest whole number, what is the limiting value of this model?arrow_forward
- bThe average rate of change of the linear function f(x)=3x+5 between any two points is ________.arrow_forwardfix or correct my answers if there is any error and give me the correct using the formatarrow_forwardSuppose there is 1 dependent variable (dissolved oxygen, Y) and 3 independent variables (water temp X1, depth X2, and hardness of water X3). Below is the result of the multiple linear regression. Coefficients Standard Error t Stat P-value Intercept 24.84 4.36 5.69 0.00 Water Temperature (C) -1.17 0.37 -3.20 0.02 Depth (feet) -0.15 0.24 -0.61 0.56 Hardness as mg/L CaCO3 -0.04 0.04 -0.95 0.37 Which of the three independent variable(s) is (are) significant predictor(s) of dissolved oxygen? Use .05 level of significance.arrow_forward
- The least-squares regression equation is y 833.6x + 11,122 where y is the median income and x is the percentage of 25 years and older with at least a bachelor's degree in the region. The scatter diagram indicates a linear relation between the two variables with a correlation coefficient of 0.7897. Complete parts (a) through (d). 55000 20000- 1s 20 25 30 35 40 4s 50 55 60 Bachelor's % (a) Predict the median income of a region in which 30% of adults 25 years and older have at least a bachelor's degree. |(Round to the nearest dollar as needed.) Median Incomearrow_forwardA different linear regression model predicts a student's GPA from the number of classes missed, weekly hours spent on studying and their age. The table below gives partial output from SPSS. Unstandardized Std Standardized Coefficients t sig. Coefficients error Constant 0.745 Number of classes missed -0.297 -0.907 Average weekly hours 0.298 0.71 spent studying Student age 0.019 0.03 If a student misses 3 classes, studies 7 hours per week and is 19 years old, what will the model predict as this student's GPA? Give your answer to 3 decimal places. (Do not be alarmed if you get a negative GPA. The question is asking what the model predicts. This would mean that the model is not that good. )arrow_forward10) A regression was run to determine if there is a relationship between hours of TV watched per day (x) and number of situps a person can do (y).The results of the regression were:y=ax+b a=-0.767 b=31.009 r2=0.609961 r=-0.781 Use this to predict the number of situps a person who watches 7.5 hours of TV can do (to one decimal place)arrow_forward
- A statistics student wanted to determine if the distance to a destination was related to the price an airline would charge to fly to that destination. The student did a regression of airfare, y, on distance, x, and obtained the printout shown below from a statistical software package. Predictor Coef Constant 83.02 Distance 0.117 Oŷ= 0.117+83.02x Oy=83.02 +0.117x Oy= 49.29 + 83.02x Oŷ= 0.028 + 0.117x Oy=83.02 + 25.25x 1 SE Coef 49.29 0.028 S = 25.25 R-Sq = 63.2 % Which of the following is the correct equation of the least-squares line? Mark this and return t-ratio 1.689 4.179 R-Sq (Adj) = 64.7% Save and Exit р 0.046 0.000 di I tv Next AM Submit Aaarrow_forwardA regression was run to determine if there is a relationship between hours of TV watched per day (xx) and number of situps a person can do (yy).The results of the regression were:y=ax+b a=-0.853 b=34.041 r2=0.389376 r=-0.624 Use this to predict the number of situps a person who watches 7 hour(s) of TV can do, and please round your answer to a whole number.arrow_forwardLet edu denote years of education, exper years of experience, wage denote wages measured in dollars per hour, and assume that the following model is correct: wage = B1 + B2edu + e where E[eledu, exper] = 0 and the error e is homoskedastic, so that Var(e|edu, exper) = Consider two regressions: (I) A first regression estimates the given model (denote those estimates by b1, b2); (I) A second regression also adds exper as a regressor -- i.e. estimates wage = ß1 + Bzedu + ßzexper + e (denote those estimates by b1, b2, b3). Which of the following statements is true? O a. The estimator b2 may be biased (i.e. we may have E[b2] # B2). O b. If wage and exper are positively correlated, then E[b3] > 0. O c. Var(b2) depends on the correlation between exper and edu (Hint: this is not asking the variance of b2.) O d. If Var(b2) = Var(b2), then the correlation between exper and edu must be zero. Oe. The correlation between wage and exper must be equal to zero. Clear my choicearrow_forward
- Functions and Change: A Modeling Approach to Coll...AlgebraISBN:9781337111348Author:Bruce Crauder, Benny Evans, Alan NoellPublisher:Cengage LearningGlencoe Algebra 1, Student Edition, 9780079039897...AlgebraISBN:9780079039897Author:CarterPublisher:McGraw Hill
- Algebra & Trigonometry with Analytic GeometryAlgebraISBN:9781133382119Author:SwokowskiPublisher:CengageBig Ideas Math A Bridge To Success Algebra 1: Stu...AlgebraISBN:9781680331141Author:HOUGHTON MIFFLIN HARCOURTPublisher:Houghton Mifflin HarcourtAlgebra and Trigonometry (MindTap Course List)AlgebraISBN:9781305071742Author:James Stewart, Lothar Redlin, Saleem WatsonPublisher:Cengage Learning
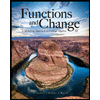
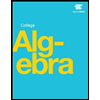
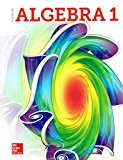
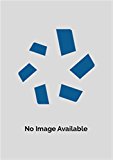
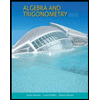