Question 1. How can gender bias in historical data be addressed? Choices Make the bias explicit and rectify it. Only use data from the last year. Only hire male employees. Ignore it. Question 2 What did the COMPAS data reveal about black and white defendants? Question 2 choices: Both groups had equal risk scores. White defendants were more likely to be labeled as risky. Black defendants were more likely to be falsely labeled as risky. Both groups had the same rate of recidivism. Question 3. In what capacity are algorithms changing employees' lives? Question 3 choices: Predicting retirement ages. Supporting hiring, promotion, training, and compensation decisions. Dictating daily schedules. They ensure higher salaries for everyone. Question 4 .Why was a gender-neutral STEM ad shown to more men than women on a social media platform? Question 4 options: The ad was mislabeled. Men were more interested in STEM. It was designed that way. Young women were more expensive to advertise to. Question 5. What does the People Analytics field employ to help managers? Question 5 options: Automated machine learning algorithms. Manual calculations. Traditional HR techniques. Interviews only. Question 6. What approach does the adjusted model NOT represent? Question 6 options: Bias-correction. Using demographic information. Estimation of promotion potential. Quota-based approach. Question 7. In the adjusted model, what percentage of women are identified as promotion candidates? Question 7 options: 9.3%. 11%. 5%. 7%. Question 8. What was the issue with Amazon's resume screening tool? Question 8 options: It had a preference for older applicants. It only selected resumes with advanced degrees. It favored male applicants for technical roles. It favored resumes with a lot of experience. Question 9. What problem did LinkedIn face with its auto-complete feature? Question 9 options: It was too slow. It suggested male names over female ones. It could only handle English names. It didn't recognize common names. Question 10. What does Figure 5 provide? Question 10 options: An illustration of the VA's promotion system. A map of the VA IT department. A checklist for key analytical questions and vendor considerations. The demographics of VA's employees. Question 11. Why should the analytical process not be applied blindly? Question 11 options: It's recommended by every expert. It saves time. It's easier to manage. To avoid potential biases in data and ensure fairness. Question 12. What is the potential problem of predictive law enforcement? Question 12 options: Fewer arrests in certain communities. Less training data for future models. Reduced crime rates. Focusing policing on areas already heavily policed. Question 13. Which domain is not mentioned as being drawn upon for HR decisions? Question 13 options: Natural language processing. Applied psychology. Statistical modeling. Quantum physics. Question 14. What percentage of the VA IT department is male-dominated? Question 14 options: 85%. 50%. 75%. 60%. Question 15. Why might a model still carry bias even if it does not directly use demographic variables? Question 15 options: All models inherently carry bias. Bias can't be carried without direct variables. Machine learning algorithms always correct for bias. Other variables can be correlated with demographic characteristics
Question 1. How can gender bias in historical data be addressed?
Choices
Make the bias explicit and rectify it.
Only use data from the last year.
Only hire male employees.
Ignore it.
Question 2 What did the COMPAS data reveal about black and white defendants?
Question 2 choices:
Both groups had equal risk scores.
White defendants were more likely to be labeled as risky.
Black defendants were more likely to be falsely labeled as risky.
Both groups had the same rate of recidivism.
Question 3. In what capacity are algorithms changing employees' lives?
Question 3 choices:
Predicting retirement ages.
Supporting hiring, promotion, training, and compensation decisions.
Dictating daily schedules.
They ensure higher salaries for everyone.
Question 4 .Why was a gender-neutral STEM ad shown to more men than women on a social media platform?
Question 4 options:
The ad was mislabeled.
Men were more interested in STEM.
It was designed that way.
Young women were more expensive to advertise to.
Question 5. What does the People Analytics field employ to help managers?
Question 5 options:
Automated machine learning algorithms.
Manual calculations.
Traditional HR techniques.
Interviews only.
Question 6. What approach does the adjusted model NOT represent?
Question 6 options:
Bias-correction.
Using demographic information.
Estimation of promotion potential.
Quota-based approach.
Question 7. In the adjusted model, what percentage of women are identified as promotion candidates?
Question 7 options:
9.3%.
11%.
5%.
7%.
Question 8. What was the issue with Amazon's resume screening tool?
Question 8 options:
It had a preference for older applicants.
It only selected resumes with advanced degrees.
It favored male applicants for technical roles.
It favored resumes with a lot of experience.
Question 9. What problem did LinkedIn face with its auto-complete feature?
Question 9 options:
It was too slow.
It suggested male names over female ones.
It could only handle English names.
It didn't recognize common names.
Question 10. What does Figure 5 provide?
Question 10 options:
An illustration of the VA's promotion system.
A map of the VA IT department.
A checklist for key analytical questions and vendor considerations.
The demographics of VA's employees.
Question 11. Why should the analytical process not be applied blindly?
Question 11 options:
It's recommended by every expert.
It saves time.
It's easier to manage.
To avoid potential biases in data and ensure fairness.
Question 12. What is the potential problem of predictive law enforcement?
Question 12 options:
Fewer arrests in certain communities.
Less training data for future models.
Reduced crime rates.
Focusing policing on areas already heavily policed.
Question 13. Which domain is not mentioned as being drawn upon for HR decisions?
Question 13 options:
Natural language processing.
Applied psychology.
Statistical modeling.
Quantum physics.
Question 14. What percentage of the VA IT department is male-dominated?
Question 14 options:
85%.
50%.
75%.
60%.
Question 15. Why might a model still carry bias even if it does not directly use demographic variables?
Question 15 options:
All models inherently carry bias.
Bias can't be carried without direct variables.
Machine learning algorithms always correct for bias.
Other variables can be correlated with demographic characteristics

Step by step
Solved in 5 steps

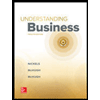
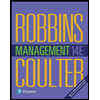
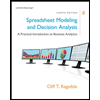
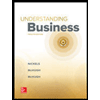
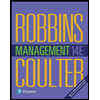
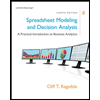
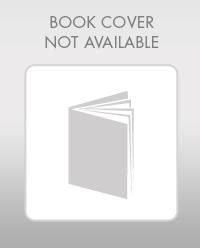
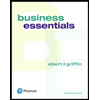
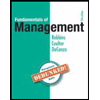