3.1. Cross-validation_ evaluating estimator performance — scikit-learn 1.4.1 documentation
pdf
keyboard_arrow_up
School
Purdue University *
*We aren’t endorsed by this school
Course
106
Subject
Statistics
Date
Apr 3, 2024
Type
Pages
12
Uploaded by ColonelField7454
3/25/24, 9:05 PM
3.1. Cross-validation: evaluating estimator performance — scikit-learn 1.4.1 documentation
https://scikit-learn.org/stable/modules/cross_validation.html
1/12
X_test, y_test
train_test_split
C
>>> import
numpy
as
np
>>> from
sklearn.model_selection
import
train_test_split
>>> from
sklearn
import
datasets
>>> from
sklearn
import
svm
>>> X, y = datasets
.
load_iris(return_X_y
=
True
)
>>> X
.
shape, y
.
shape
((150, 4), (150,))
>>> X_train, X_test, y_train, y_test = train_test_split(
... X, y, test_size
=
0.4
, random_state
=
0
)
>>> X_train
.
shape, y_train
.
shape
((90, 4), (90,))
>>> X_test
.
shape, y_test
.
shape
((60, 4), (60,))
>>> clf = svm
.
SVC(kernel
=
'linear'
, C
=
1
)
.
fit(X_train, y_train)
>>> clf
.
score(X_test, y_test)
0.96...
3/25/24, 9:05 PM
3.1. Cross-validation: evaluating estimator performance — scikit-learn 1.4.1 documentation
https://scikit-learn.org/stable/modules/cross_validation.html
2/12
cross_val_score
score
cv
cross_val_score
KFold
StratifiedKFold
ClassifierMixin
>>> from
sklearn.model_selection
import
cross_val_score
>>> clf = svm
.
SVC(kernel
=
'linear'
, C
=
1
, random_state
=
42
)
>>> scores = cross_val_score(clf, X, y, cv
=
5
)
>>> scores
array([0.96..., 1. , 0.96..., 0.96..., 1. ])
>>> print
(
"
%0.2f
accuracy with a standard deviation of %0.2f
" % (scores
.
mean(), scores
.
std()))
0.98 accuracy with a standard deviation of 0.02
>>> from
sklearn
import
metrics
>>> scores = cross_val_score(
... clf, X, y, cv
=
5
, scoring
=
'f1_macro'
)
>>> scores
array([0.96..., 1. ..., 0.96..., 0.96..., 1. ])
>>> from
sklearn.model_selection
import
ShuffleSplit
>>> n_samples = X
.
shape[
0
]
>>> cv = ShuffleSplit(n_splits
=
5
, test_size
=
0.3
, random_state
=
0
)
>>> cross_val_score(clf, X, y, cv
=
cv)
array([0.977..., 0.977..., 1. ..., 0.955..., 1. ])
3/25/24, 9:05 PM
3.1. Cross-validation: evaluating estimator performance — scikit-learn 1.4.1 documentation
https://scikit-learn.org/stable/modules/cross_validation.html
3/12
Pipeline
cross_validate
cross_val_score
['test_score', 'fit_time',
'score_time']
['test_<scorer1_name>', 'test_<scorer2_name>',
'test_<scorer...>', 'fit_time', 'score_time']
return_train_score
False
True
return_estimator=True
re‐
turn_indices=True
>>> def
custom_cv_2folds
(X):
... n = X
.
shape[
0
]
... i = 1
... while
i <= 2
:
... idx = np
.
arange(n * (i - 1
) / 2
, n * i / 2
, dtype
=
int
)
... yield
idx, idx
... i += 1
...
>>> custom_cv = custom_cv_2folds(X)
>>> cross_val_score(clf, X, y, cv
=
custom_cv)
array([1. , 0.973...])
>>> from
sklearn
import
preprocessing
>>> X_train, X_test, y_train, y_test = train_test_split(
... X, y, test_size
=
0.4
, random_state
=
0
)
>>> scaler = preprocessing
.
StandardScaler()
.
fit(X_train)
>>> X_train_transformed = scaler
.
transform(X_train)
>>> clf = svm
.
SVC(C
=
1
)
.
fit(X_train_transformed, y_train)
>>> X_test_transformed = scaler
.
transform(X_test)
>>> clf
.
score(X_test_transformed, y_test)
0.9333...
>>> from
sklearn.pipeline
import
make_pipeline
>>> clf = make_pipeline(preprocessing
.
StandardScaler(), svm
.
SVC(C
=
1
))
>>> cross_val_score(clf, X, y, cv
=
cv)
array([0.977..., 0.933..., 0.955..., 0.933..., 0.977...])
>>> from
sklearn.model_selection
import
cross_validate
>>> from
sklearn.metrics
import
recall_score
>>> scoring = [
'precision_macro'
, 'recall_macro'
]
>>> clf = svm
.
SVC(kernel
=
'linear'
, C
=
1
, random_state
=
0
)
>>> scores = cross_validate(clf, X, y, scoring
=
scoring)
>>> sorted
(scores
.
keys())
['fit_time', 'score_time', 'test_precision_macro', 'test_recall_macro']
>>> scores[
'test_recall_macro'
]
array([0.96..., 1. ..., 0.96..., 0.96..., 1. ])
Your preview ends here
Eager to read complete document? Join bartleby learn and gain access to the full version
- Access to all documents
- Unlimited textbook solutions
- 24/7 expert homework help
3/25/24, 9:05 PM
3.1. Cross-validation: evaluating estimator performance — scikit-learn 1.4.1 documentation
https://scikit-learn.org/stable/modules/cross_validation.html
4/12
cross_validate
cross_val_predict
cross_val_score
cross_val_predict
cross_val_score
cross_val_score
cross_val_predict
cross_val_predict
cross_val_predict
KFold
>>> from
sklearn.metrics
import
make_scorer
>>> scoring = {
'prec_macro'
: 'precision_macro'
,
... 'rec_macro'
: make_scorer(recall_score, average
=
'macro'
)}
>>> scores = cross_validate(clf, X, y, scoring
=
scoring,
... cv
=
5
, return_train_score
=
True
)
>>> sorted
(scores
.
keys())
['fit_time', 'score_time', 'test_prec_macro', 'test_rec_macro',
'train_prec_macro', 'train_rec_macro']
>>> scores[
'train_rec_macro'
]
array([0.97..., 0.97..., 0.99..., 0.98..., 0.98...])
>>> scores = cross_validate(clf, X, y,
... scoring
=
'precision_macro'
, cv
=
5
,
... return_estimator
=
True
)
>>> sorted
(scores
.
keys())
['estimator', 'fit_time', 'score_time', 'test_score']
3/25/24, 9:05 PM
3.1. Cross-validation: evaluating estimator performance — scikit-learn 1.4.1 documentation
https://scikit-learn.org/stable/modules/cross_validation.html
5/12
KFold
RepeatedKFold
KFold
RepeatedStratifiedKFold
LeaveOneOut
>>> import
numpy
as
np
>>> from
sklearn.model_selection
import
KFold
>>> X = [
"a"
, "b"
, "c"
, "d"
]
>>> kf = KFold(n_splits
=
2
)
>>> for
train, test in
kf
.
split(X):
... print
(
"
%s
%s
" % (train, test))
[2 3] [0 1]
[0 1] [2 3]
>>> X = np
.
array([[
0.
, 0.
], [
1.
, 1.
], [
-
1.
, -
1.
], [
2.
, 2.
]])
>>> y = np
.
array([
0
, 1
, 0
, 1
])
>>> X_train, X_test, y_train, y_test = X[train], X[test], y[train], y[test]
>>> import
numpy
as
np
>>> from
sklearn.model_selection
import
RepeatedKFold
>>> X = np
.
array([[
1
, 2
], [
3
, 4
], [
1
, 2
], [
3
, 4
]])
>>> random_state = 12883823
>>> rkf = RepeatedKFold(n_splits
=
2
, n_repeats
=
2
, random_state
=
random_state)
>>> for
train, test in
rkf
.
split(X):
... print
(
"
%s
%s
" % (train, test))
...
[2 3] [0 1]
[0 1] [2 3]
[0 2] [1 3]
[1 3] [0 2]
>>> from
sklearn.model_selection
import
LeaveOneOut
>>> X = [
1
, 2
, 3
, 4
]
>>> loo = LeaveOneOut()
>>> for
train, test in
loo
.
split(X):
... print
(
"
%s
%s
" % (train, test))
[1 2 3] [0]
[0 2 3] [1]
[0 1 3] [2]
[0 1 2] [3]
3/25/24, 9:05 PM
3.1. Cross-validation: evaluating estimator performance — scikit-learn 1.4.1 documentation
https://scikit-learn.org/stable/modules/cross_validation.html
6/12
LeavePOut
LeaveOneOut
LeaveOneOut
KFold
ShuffleSplit
random_state
ShuffleSplit
ShuffleSplit
KFold
>>> from
sklearn.model_selection
import
LeavePOut
>>> X = np
.
ones(
4
)
>>> lpo = LeavePOut(p
=
2
)
>>> for
train, test in
lpo
.
split(X):
... print
(
"
%s
%s
" % (train, test))
[2 3] [0 1]
[1 3] [0 2]
[1 2] [0 3]
[0 3] [1 2]
[0 2] [1 3]
[0 1] [2 3]
>>> from
sklearn.model_selection
import
ShuffleSplit
>>> X = np
.
arange(
10
)
>>> ss = ShuffleSplit(n_splits
=
5
, test_size
=
0.25
, random_state
=
0
)
>>> for
train_index, test_index in
ss
.
split(X):
... print
(
"
%s
%s
" % (train_index, test_index))
[9 1 6 7 3 0 5] [2 8 4]
[2 9 8 0 6 7 4] [3 5 1]
[4 5 1 0 6 9 7] [2 3 8]
[2 7 5 8 0 3 4] [6 1 9]
[4 1 0 6 8 9 3] [5 2 7]
Your preview ends here
Eager to read complete document? Join bartleby learn and gain access to the full version
- Access to all documents
- Unlimited textbook solutions
- 24/7 expert homework help
3/25/24, 9:05 PM
3.1. Cross-validation: evaluating estimator performance — scikit-learn 1.4.1 documentation
https://scikit-learn.org/stable/modules/cross_validation.html
7/12
StratifiedKFold
StratifiedShuffleSplit
StratifiedKFold
KFold
StratifiedKFold
RepeatedStratifiedKFold
StratifiedShuffleSplit
>>> from
sklearn.model_selection
import
StratifiedKFold, KFold
>>> import
numpy
as
np
>>> X, y = np
.
ones((
50
, 1
)), np
.
hstack(([
0
] * 45
, [
1
] * 5
))
>>> skf = StratifiedKFold(n_splits
=
3
)
>>> for
train, test in
skf
.
split(X, y):
... print
(
'train - {}
| test - {}
'
.
format(
... np
.
bincount(y[train]), np
.
bincount(y[test])))
train - [30 3] | test - [15 2]
train - [30 3] | test - [15 2]
train - [30 4] | test - [15 1]
>>> kf = KFold(n_splits
=
3
)
>>> for
train, test in
kf
.
split(X, y):
... print
(
'train - {}
| test - {}
'
.
format(
... np
.
bincount(y[train]), np
.
bincount(y[test])))
train - [28 5] | test - [17]
train - [28 5] | test - [17]
train - [34] | test - [11 5]
3/25/24, 9:05 PM
3.1. Cross-validation: evaluating estimator performance — scikit-learn 1.4.1 documentation
https://scikit-learn.org/stable/modules/cross_validation.html
8/12
groups
GroupKFold
GroupKFold
StratifiedGroupKFold
KFold
GroupKFold
KFold
GroupKFold
KFold
shuffle=True
StratifiedGroupKFold
StratifiedKFold
GroupKFold
GroupKFold
>>> from
sklearn.model_selection
import
GroupKFold
>>> X = [
0.1
, 0.2
, 2.2
, 2.4
, 2.3
, 4.55
, 5.8
, 8.8
, 9
, 10
]
>>> y = [
"a"
, "b"
, "b"
, "b"
, "c"
, "c"
, "c"
, "d"
, "d"
, "d"
]
>>> groups = [
1
, 1
, 1
, 2
, 2
, 2
, 3
, 3
, 3
, 3
]
>>> gkf = GroupKFold(n_splits
=
3
)
>>> for
train, test in
gkf
.
split(X, y, groups
=
groups):
... print
(
"
%s
%s
" % (train, test))
[0 1 2 3 4 5] [6 7 8 9]
[0 1 2 6 7 8 9] [3 4 5]
[3 4 5 6 7 8 9] [0 1 2]
>>> from
sklearn.model_selection
import
StratifiedGroupKFold
>>> X = list
(
range
(
18
))
>>> y = [
1
] * 6 + [
0
] * 12
>>> groups = [
1
, 2
, 3
, 3
, 4
, 4
, 1
, 1
, 2
, 2
, 3
, 4
, 5
, 5
, 5
, 6
, 6
, 6
]
>>> sgkf = StratifiedGroupKFold(n_splits
=
3
)
>>> for
train, test in
sgkf
.
split(X, y, groups
=
groups):
... print
(
"
%s
%s
" % (train, test))
[ 0 2 3 4 5 6 7 10 11 15 16 17] [ 1 8 9 12 13 14]
[ 0 1 4 5 6 7 8 9 11 12 13 14] [ 2 3 10 15 16 17]
[ 1 2 3 8 9 10 12 13 14 15 16 17] [ 0 4 5 6 7 11]
3/25/24, 9:05 PM
3.1. Cross-validation: evaluating estimator performance — scikit-learn 1.4.1 documentation
https://scikit-learn.org/stable/modules/cross_validation.html
9/12
GroupKFold
LeaveOneGroupOut
LeavePGroupsOut
n_groups=1
GroupKFold
n_splits
groups
LeaveOneGroupOut
LeavePGroupsOut
LeaveOneGroupOut
>>> from
sklearn.model_selection
import
LeaveOneGroupOut
>>> X = [
1
, 5
, 10
, 50
, 60
, 70
, 80
]
>>> y = [
0
, 1
, 1
, 2
, 2
, 2
, 2
]
>>> groups = [
1
, 1
, 2
, 2
, 3
, 3
, 3
]
>>> logo = LeaveOneGroupOut()
>>> for
train, test in
logo
.
split(X, y, groups
=
groups):
... print
(
"
%s
%s
" % (train, test))
[2 3 4 5 6] [0 1]
[0 1 4 5 6] [2 3]
[0 1 2 3] [4 5 6]
>>> from
sklearn.model_selection
import
LeavePGroupsOut
>>> X = np
.
arange(
6
)
>>> y = [
1
, 1
, 1
, 2
, 2
, 2
]
>>> groups = [
1
, 1
, 2
, 2
, 3
, 3
]
>>> lpgo = LeavePGroupsOut(n_groups
=
2
)
>>> for
train, test in
lpgo
.
split(X, y, groups
=
groups):
... print
(
"
%s
%s
" % (train, test))
[4 5] [0 1 2 3]
[2 3] [0 1 4 5]
[0 1] [2 3 4 5]
Your preview ends here
Eager to read complete document? Join bartleby learn and gain access to the full version
- Access to all documents
- Unlimited textbook solutions
- 24/7 expert homework help
3/25/24, 9:05 PM
3.1. Cross-validation: evaluating estimator performance — scikit-learn 1.4.1 documentation
https://scikit-learn.org/stable/modules/cross_validation.html
10/12
GroupShuffleSplit
ShuffleSplit
LeavePGroupsOut
LeavePGroupsOut
GroupShuffleSplit
LeavePGroupsOut
PredefinedSplit
test_fold
train_test_split
ShuffleSplit
split()
>>> from
sklearn.model_selection
import
GroupShuffleSplit
>>> X = [
0.1
, 0.2
, 2.2
, 2.4
, 2.3
, 4.55
, 5.8
, 0.001
]
>>> y = [
"a"
, "b"
, "b"
, "b"
, "c"
, "c"
, "c"
, "a"
]
>>> groups = [
1
, 1
, 2
, 2
, 3
, 3
, 4
, 4
]
>>> gss = GroupShuffleSplit(n_splits
=
4
, test_size
=
0.5
, random_state
=
0
)
>>> for
train, test in
gss
.
split(X, y, groups
=
groups):
... print
(
"
%s
%s
" % (train, test))
...
[0 1 2 3] [4 5 6 7]
[2 3 6 7] [0 1 4 5]
[2 3 4 5] [0 1 6 7]
[4 5 6 7] [0 1 2 3]
>>> import
numpy
as
np
>>> from
sklearn.model_selection
import
GroupShuffleSplit
>>> X = np
.
array([
0.1
, 0.2
, 2.2
, 2.4
, 2.3
, 4.55
, 5.8
, 0.001
])
>>> y = np
.
array([
"a"
, "b"
, "b"
, "b"
, "c"
, "c"
, "c"
, "a"
])
>>> groups = np
.
array([
1
, 1
, 2
, 2
, 3
, 3
, 4
, 4
])
>>> train_indx, test_indx = next
(
... GroupShuffleSplit(random_state
=
7
)
.
split(X, y, groups)
... )
>>> X_train, X_test, y_train, y_test = \
... X[train_indx], X[test_indx], y[train_indx], y[test_indx]
>>> X_train
.
shape, X_test
.
shape
((6,), (2,))
>>> np
.
unique(groups[train_indx]), np
.
unique(groups[test_indx])
(array([1, 2, 4]), array([3]))
3/25/24, 9:05 PM
3.1. Cross-validation: evaluating estimator performance — scikit-learn 1.4.1 documentation
https://scikit-learn.org/stable/modules/cross_validation.html
11/12
KFold
ShuffleSplit
TimeSeriesSplit
TimeSeriesSplit
KFold
cv=some_integer
cross_val_score
train_test_split
random_state
None
KFold(..., shuffle=True)
GridSearchCV
fit
random_state
>>> from
sklearn.model_selection
import
TimeSeriesSplit
>>> X = np
.
array([[
1
, 2
], [
3
, 4
], [
1
, 2
], [
3
, 4
], [
1
, 2
], [
3
, 4
]])
>>> y = np
.
array([
1
, 2
, 3
, 4
, 5
, 6
])
>>> tscv = TimeSeriesSplit(n_splits
=
3
)
>>> print
(tscv)
TimeSeriesSplit(gap=0, max_train_size=None, n_splits=3, test_size=None)
>>> for
train, test in
tscv
.
split(X):
... print
(
"
%s
%s
" % (train, test))
[0 1 2] [3]
[0 1 2 3] [4]
[0 1 2 3 4] [5]
3/25/24, 9:05 PM
3.1. Cross-validation: evaluating estimator performance — scikit-learn 1.4.1 documentation
https://scikit-learn.org/stable/modules/cross_validation.html
12/12
permutation_test_score
permutation_test_score
n_permutations
n_permutations
cv
permutation_test_score
permutation_test_score
(n_permutations + 1) * n_cv
Your preview ends here
Eager to read complete document? Join bartleby learn and gain access to the full version
- Access to all documents
- Unlimited textbook solutions
- 24/7 expert homework help
Related Questions
6/p = 5/11
the cross products property way?? idk
arrow_forward
Define Least Squares Regression Unbiased Estimators α^, β^, σ^²?
arrow_forward
The least-squares regression line for a set of (Age, Skill_Score) data is yn = 5.0x + 0.7. The data point for age 6has residual -1.4. What is the skill score for age 6?A) -4.6 B) 4.6 C) 29.3D) 30.7 E) 32.1
arrow_forward
A new bandage has been developed that is thought to aid in the healing of small cuts. The bandage has special air circulation holes as well as antiseptic within the bandage pad. Controlled one centimeter cuts in volunteers were completely healed in an average of 11 days utilizing the old bandage. Below are the healing times for similar cuts utilizing the new bandage. Did the new bandage significantly reduce healing times? Analyze both parametrically and nonparametrically (with both the sign test and Wilcoxon signed-rank test).
9.5, 9.7,10.5, 10.2, 10.8, 10.9, 10.1, 11.3, 9.9, 9.6, 11.2, 11.0, 10.0, 10.4, 11.4, 9.8
arrow_forward
T/275T: Statistic X zy Section 5.5 - QNT/275T: Statistics X
T_56598163/chapter/5/section/5
qx3zay7
cs for Decision Making home >
erence between two population proportions
5.5. 1. HYPOTHESIS TEST FOR THe difference between two population proportions.
Jump to level 1
Test statistic = Ex: 0.12
A political campaign is interested in whether a geographic difference existed in support
for raising the minimum wage in a certain state. Polls were conducted in the two largest
cities in the state about raising the minimum wage. In city 1, a poll of 800 randomly
selected voters found that 510 supported raising the minimum wage. In city 2, a poll of
1000 randomly selected voters found that 602 supported raising the minimum wage.
What type of hypothesis test should be performed? Two-tailed z-test v
℗1 = 0.637
P2
p = 0.617
p-value = Ex: 0.123
Check
+
Does sufficient evidence exist to support the claim that the level of support differs
Select v
between the two cities at the a = 0.1 significance level?…
arrow_forward
Interpret the generated results at 5% level of significance.
arrow_forward
Interpreting technology: The following display from the TI-84 Plus calculator presents the least-squares regression line for predicting the price of a certain stock y from the prime interest rate in percent x
LinReg
y=+abx
a=2.29525776
b=0.38970413
r^2=0.4319044662
r=0.65719439
Regression line equation: 2.295225776+.38970413x
What is the correlation between the interest rate and the yield of the stock?
arrow_forward
Calendar
08.07 WebAssign: Section 8.2: Te X
W Section 8.2: Testing Mean with K X
i webassign.net/web/Student/Assignment-Responses/submit?dep=23798486&tags=autosave#question3878843_3
E student.fz.k12.mo.us bookmarks
I Future Plans Tyler Student
É AP Students - Coll.
A Launch Dashboard
O Canvas Dashboard
Calendar
a0 Duolingo
* MackinVIA ap stats book
A ap stats assignmen.
E ap us history textbo.
CENGAGE WEBASSIGN
O EN
folkertsemma25@student.fz.k12.mo.us (sign out)
Home
My Assignments
Grades
Communication
Calendar
E My eBooks
E MASTER - AP Statistics 20-21, section APStats (Sem 2) SP21,
* INSTRUCTOR
Section 8.2: Testing Mean with Known SD (Homework)
Justin Thomas
Springfield Public Schools.MO
A Print Assignment
Current Score
Due Date
QUESTION
5
SAT, MAY 29, 2021
1
3
4
TOTAL SCORE
11:59 PM CDT
POINTS
1/1
1/1
1/1
6/8
4/4
13/15
86.7%
+ Request Extension
Assignment Submission & Scoring
Assignment Submission
For this assignment, you submit answers by question parts. The number of submissions…
arrow_forward
Interpreting technology: The following display from the TI-84 Plus calculator presents the least-squares regression line for predicting the price of a certain stock y from the prime interest rate in percent x
LinReg
y=+a+bx
a=2.29525776
b=0.38970413
r^2=0.4319044662
r=0.65719439
Predict the price when the prime interest rate is 6%. Round the answer to at least four decimal places.
arrow_forward
I ONLY NEED PART C,D, and E answered please thanks
A regression was run to determine if there is a relationship between the happiness index (y) and life expectancy in years of a given country (x).The results of the regression were:
ˆyy^=a+bxa=-1.102b=0.074
(a) Write the equation of the Least Squares Regression line of the formˆyy^= + x(b) Which is a possible value for the correlation coefficient, rr?
-0.858
-1.07
1.07
0.858
(c) If a country increases its life expectancy, the happiness index will
decrease
increase
(d) If the life expectancy is increased by 2.5 years in a certain country, how much will the happiness index change? Round to two decimal places._____(e) Use the regression line to predict the happiness index of a country with a life expectancy of 63 years. Round to two decimal places._______Use the space below to type your answer AND/OR to upload a picture of your work for all the questions in this problem.
arrow_forward
Please answer as many as your allowed too. Thank you :)
A regression was run to determine if there is a relationship between the happiness index (y) and life expectancy in years of a given country (x).The results of the regression were:
ˆyy^=a+bxa=-1.68b=0.168
(a) Write the equation of the Least Squares Regression line of the formˆyy^= + x(b) Which is a possible value for the correlation coefficient, rr?
-1.417
1.417
0.702
-0.702
(c) If a country increases its life expectancy, the happiness index will
increase
decrease
(d) If the life expectancy is increased by 0.5 years in a certain country, how much will the happiness index change? Round to two decimal places.(e) Use the regression line to predict the happiness index of a country with a life expectancy of 69 years. Round to two decimal places.
arrow_forward
Use the maximum likehood method in deriving the error variance in regression
arrow_forward
Benign prostatic hyperplasia is a noncancerous
enlargement of the prostate gland that adversely
affects the quality of life (QoL) of millions of men. A
study of minimally invasive procedures for the
treatment for this condition looked at pretreatment
QoL (qol_base) and quality of life after 3 month on
treatment (qol_3mo)
The baseline data for 10 patients and their 3 month
follow-up data is presented below:
MAXFLO_B = maximum urine flow at baseline (urine
flow measurement scale misplaced)
MAXFLO3M = maximum urine flow after 3 months of
treatment
maxflo_b
maxflo3m
7
5
8
18
8
13
9
16
11
8
4
9
12
10
6
8
14
10
13
arrow_forward
Safari
File
Edit
View
History
Bookmarks
Window
Help
Thu Oct 21 9:20 AM
learn-us-east-1-prod-fleet02-xythos.content.blackboardcdn.com
untit
Bb Test Reviews – 202210:13745 Math for Bus and Soc Scs (...
Bb https://learn-us-east-1-prod-fleet02-xythos.content.blackboardcdn.co...
Function Table
Perform the indicated row operations on the following matrix.
[
1 -5 4
Scre
2021-0
2 25
5) –3R1 -R1
1 -5| 4
A)
-6 -6|-15
[
1 -5 4
B)
-1 17 7
-3 15 -12
-3 -5 4
D)
-6 25
thinko
2 2
5
Page 1
W
(o
of
arrow_forward
SEE MORE QUESTIONS
Recommended textbooks for you
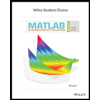
MATLAB: An Introduction with Applications
Statistics
ISBN:9781119256830
Author:Amos Gilat
Publisher:John Wiley & Sons Inc
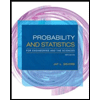
Probability and Statistics for Engineering and th...
Statistics
ISBN:9781305251809
Author:Jay L. Devore
Publisher:Cengage Learning
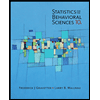
Statistics for The Behavioral Sciences (MindTap C...
Statistics
ISBN:9781305504912
Author:Frederick J Gravetter, Larry B. Wallnau
Publisher:Cengage Learning
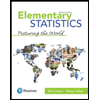
Elementary Statistics: Picturing the World (7th E...
Statistics
ISBN:9780134683416
Author:Ron Larson, Betsy Farber
Publisher:PEARSON
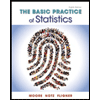
The Basic Practice of Statistics
Statistics
ISBN:9781319042578
Author:David S. Moore, William I. Notz, Michael A. Fligner
Publisher:W. H. Freeman
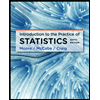
Introduction to the Practice of Statistics
Statistics
ISBN:9781319013387
Author:David S. Moore, George P. McCabe, Bruce A. Craig
Publisher:W. H. Freeman
Related Questions
- 6/p = 5/11 the cross products property way?? idkarrow_forwardDefine Least Squares Regression Unbiased Estimators α^, β^, σ^²?arrow_forwardThe least-squares regression line for a set of (Age, Skill_Score) data is yn = 5.0x + 0.7. The data point for age 6has residual -1.4. What is the skill score for age 6?A) -4.6 B) 4.6 C) 29.3D) 30.7 E) 32.1arrow_forward
- A new bandage has been developed that is thought to aid in the healing of small cuts. The bandage has special air circulation holes as well as antiseptic within the bandage pad. Controlled one centimeter cuts in volunteers were completely healed in an average of 11 days utilizing the old bandage. Below are the healing times for similar cuts utilizing the new bandage. Did the new bandage significantly reduce healing times? Analyze both parametrically and nonparametrically (with both the sign test and Wilcoxon signed-rank test). 9.5, 9.7,10.5, 10.2, 10.8, 10.9, 10.1, 11.3, 9.9, 9.6, 11.2, 11.0, 10.0, 10.4, 11.4, 9.8arrow_forwardT/275T: Statistic X zy Section 5.5 - QNT/275T: Statistics X T_56598163/chapter/5/section/5 qx3zay7 cs for Decision Making home > erence between two population proportions 5.5. 1. HYPOTHESIS TEST FOR THe difference between two population proportions. Jump to level 1 Test statistic = Ex: 0.12 A political campaign is interested in whether a geographic difference existed in support for raising the minimum wage in a certain state. Polls were conducted in the two largest cities in the state about raising the minimum wage. In city 1, a poll of 800 randomly selected voters found that 510 supported raising the minimum wage. In city 2, a poll of 1000 randomly selected voters found that 602 supported raising the minimum wage. What type of hypothesis test should be performed? Two-tailed z-test v ℗1 = 0.637 P2 p = 0.617 p-value = Ex: 0.123 Check + Does sufficient evidence exist to support the claim that the level of support differs Select v between the two cities at the a = 0.1 significance level?…arrow_forwardInterpret the generated results at 5% level of significance.arrow_forward
- Interpreting technology: The following display from the TI-84 Plus calculator presents the least-squares regression line for predicting the price of a certain stock y from the prime interest rate in percent x LinReg y=+abx a=2.29525776 b=0.38970413 r^2=0.4319044662 r=0.65719439 Regression line equation: 2.295225776+.38970413x What is the correlation between the interest rate and the yield of the stock?arrow_forwardCalendar 08.07 WebAssign: Section 8.2: Te X W Section 8.2: Testing Mean with K X i webassign.net/web/Student/Assignment-Responses/submit?dep=23798486&tags=autosave#question3878843_3 E student.fz.k12.mo.us bookmarks I Future Plans Tyler Student É AP Students - Coll. A Launch Dashboard O Canvas Dashboard Calendar a0 Duolingo * MackinVIA ap stats book A ap stats assignmen. E ap us history textbo. CENGAGE WEBASSIGN O EN folkertsemma25@student.fz.k12.mo.us (sign out) Home My Assignments Grades Communication Calendar E My eBooks E MASTER - AP Statistics 20-21, section APStats (Sem 2) SP21, * INSTRUCTOR Section 8.2: Testing Mean with Known SD (Homework) Justin Thomas Springfield Public Schools.MO A Print Assignment Current Score Due Date QUESTION 5 SAT, MAY 29, 2021 1 3 4 TOTAL SCORE 11:59 PM CDT POINTS 1/1 1/1 1/1 6/8 4/4 13/15 86.7% + Request Extension Assignment Submission & Scoring Assignment Submission For this assignment, you submit answers by question parts. The number of submissions…arrow_forwardInterpreting technology: The following display from the TI-84 Plus calculator presents the least-squares regression line for predicting the price of a certain stock y from the prime interest rate in percent x LinReg y=+a+bx a=2.29525776 b=0.38970413 r^2=0.4319044662 r=0.65719439 Predict the price when the prime interest rate is 6%. Round the answer to at least four decimal places.arrow_forward
- I ONLY NEED PART C,D, and E answered please thanks A regression was run to determine if there is a relationship between the happiness index (y) and life expectancy in years of a given country (x).The results of the regression were: ˆyy^=a+bxa=-1.102b=0.074 (a) Write the equation of the Least Squares Regression line of the formˆyy^= + x(b) Which is a possible value for the correlation coefficient, rr? -0.858 -1.07 1.07 0.858 (c) If a country increases its life expectancy, the happiness index will decrease increase (d) If the life expectancy is increased by 2.5 years in a certain country, how much will the happiness index change? Round to two decimal places._____(e) Use the regression line to predict the happiness index of a country with a life expectancy of 63 years. Round to two decimal places._______Use the space below to type your answer AND/OR to upload a picture of your work for all the questions in this problem.arrow_forwardPlease answer as many as your allowed too. Thank you :) A regression was run to determine if there is a relationship between the happiness index (y) and life expectancy in years of a given country (x).The results of the regression were: ˆyy^=a+bxa=-1.68b=0.168 (a) Write the equation of the Least Squares Regression line of the formˆyy^= + x(b) Which is a possible value for the correlation coefficient, rr? -1.417 1.417 0.702 -0.702 (c) If a country increases its life expectancy, the happiness index will increase decrease (d) If the life expectancy is increased by 0.5 years in a certain country, how much will the happiness index change? Round to two decimal places.(e) Use the regression line to predict the happiness index of a country with a life expectancy of 69 years. Round to two decimal places.arrow_forwardUse the maximum likehood method in deriving the error variance in regressionarrow_forward
arrow_back_ios
SEE MORE QUESTIONS
arrow_forward_ios
Recommended textbooks for you
- MATLAB: An Introduction with ApplicationsStatisticsISBN:9781119256830Author:Amos GilatPublisher:John Wiley & Sons IncProbability and Statistics for Engineering and th...StatisticsISBN:9781305251809Author:Jay L. DevorePublisher:Cengage LearningStatistics for The Behavioral Sciences (MindTap C...StatisticsISBN:9781305504912Author:Frederick J Gravetter, Larry B. WallnauPublisher:Cengage Learning
- Elementary Statistics: Picturing the World (7th E...StatisticsISBN:9780134683416Author:Ron Larson, Betsy FarberPublisher:PEARSONThe Basic Practice of StatisticsStatisticsISBN:9781319042578Author:David S. Moore, William I. Notz, Michael A. FlignerPublisher:W. H. FreemanIntroduction to the Practice of StatisticsStatisticsISBN:9781319013387Author:David S. Moore, George P. McCabe, Bruce A. CraigPublisher:W. H. Freeman
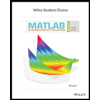
MATLAB: An Introduction with Applications
Statistics
ISBN:9781119256830
Author:Amos Gilat
Publisher:John Wiley & Sons Inc
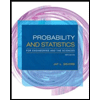
Probability and Statistics for Engineering and th...
Statistics
ISBN:9781305251809
Author:Jay L. Devore
Publisher:Cengage Learning
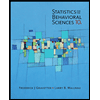
Statistics for The Behavioral Sciences (MindTap C...
Statistics
ISBN:9781305504912
Author:Frederick J Gravetter, Larry B. Wallnau
Publisher:Cengage Learning
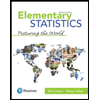
Elementary Statistics: Picturing the World (7th E...
Statistics
ISBN:9780134683416
Author:Ron Larson, Betsy Farber
Publisher:PEARSON
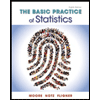
The Basic Practice of Statistics
Statistics
ISBN:9781319042578
Author:David S. Moore, William I. Notz, Michael A. Fligner
Publisher:W. H. Freeman
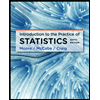
Introduction to the Practice of Statistics
Statistics
ISBN:9781319013387
Author:David S. Moore, George P. McCabe, Bruce A. Craig
Publisher:W. H. Freeman