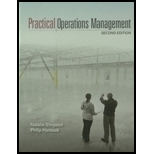
Concept explainers
A
Interpretation:Determine the forecast error for the month of April.
Concept Introduction:Forecast error indicates the percent of error in the forecasted value based on the actual outcome.
B
Interpretation:Determine the error percent for the month of July.
Concept Introduction: Forecast error indicates the percent of error in the forecasted value based on the actual outcome.
C
Interpretation: Determine the Mean Error, mean squared error, mean absolute percent error, mean absolute deviation and tracking signal in this 5-month
Concept Introduction: Using regression, we will be able to define relationship between any two variables, denoting the cause and effect. The method can also be used to forecast the future depending on the past performances.
D
Interpretation: Determine the forecast in June as per the 3 month moving average.
Concept Introduction: Forecast error indicates the percent of error in the forecasted value based on the actual outcome.
E
Interpretation: Find out the forecast based on the simple exponential smoothing for the month of August.
Concept Introduction: Forecast error indicates the percent of error in the forecasted value based on the actual outcome.

Want to see the full answer?
Check out a sample textbook solution
Chapter 4 Solutions
Practical Operations Management
- Women who ask for what they want in negotiation are less well-liked than women who do not self-advocate. However, nonassertive, other-advocating women suffer a leadership backlash and are regarded as less competent because their behavior is regarded to be _____ and _____. A. high-negative feminine; low-positive masculine B. high-positive feminine; high-positive masculine C. high-negative masculine; low-negative feminine D. low-positive masculine; low-positive femininearrow_forwardThere are five most recognized personality traits that can reliably be measured and predict negotiator behavior in a number of different situations. All of the following are one of those "Big 5" personality traits except _____. A. conscientiousness B. introversion C. agreeableness D. openness to experiencearrow_forwardWith regard to reputation in negotiation, negotiators who use adversarial, stubborn, and ethically questionable behavior often have the effect of _____. A. improving their business relationships B. decreasing their effectiveness as a negotiator C. improving their business relationships D. decreasing their group statusarrow_forward
- When it comes to assertiveness, there is only a modest link between negotiators' self-views and how the counterparty sees them. Many negotiators come away from a negotiation thinking they came on too strong with the counterparty. The _____ refers to the fact that negotiators believe they are coming on too strong with the counterparty, but they actually are not. A. Collective trap illusion B. Attribution error C. Aggressive anchoring bias D. Line-crossing illusionarrow_forwardAs you think about the issue of using chatbots in contract negotiations, consider whether other facets and concepts of negotiations that we have discussed and whether they would be adequately addressed.arrow_forwardWhile I am not a fan of AI as of yet, I do understand the endless possibilities. Based on the research, it is clear that AI has great potential for negotiation (Yang, 2025). Herold et al. (2025) suggested that AI can flag potential risks and liabilities, allowing negotiators to address them and mitigate potential problems proactively. AI can draft new contract templates by examining industry standards and past contracts, and AI technology can help lawyers spot errors and inconsistencies in contract drafts. In relation to risk management, AI can flag possible risks and liabilities, allowing negotiators to proactively address them and lessen potential problems, which can speed up the negotiation process, making the negotiation efficient because AI can industrialize tasks like document review, redlining, and finding potential issues, significantly reducing negotiation time. Lastly, AI can analyze vast amounts of data and identify errors, inconsistencies, and irregularities in…arrow_forward
- What is a main thought on using AI in contract negotiations?arrow_forwardWhat are some people thoughts on using AI in contract negotiations?arrow_forward3. Develop a high-level or summary: a. Risk Management Plan Focus on specific, actionable steps for each risk and mitigation strategy.Provide detailed timelines for procurement, stakeholder engagement, and risk monitoring.Avoid over-simplifying and add more technical details in areas like quality assurance and financial control measures. Add a risk prioritization method and mention how risks will be monitored and reviewed throughout the project lifecycle. Overall, it is well organized andc overs key risks.arrow_forward
- 3. Develop a high-level or summary: Human Resource Management Plan Provide more concrete timelines and actionable steps for human resource management.Include more detailed risk management strategies and link them more explicitly to the overall project plan.Expand on how training and development will be evaluated and tracked.Also, the overall length is good, but some sections could be condensed by eliminating repetition (e.g., you discuss stakeholder communication and engagement in two sections without adding new information).Try not to repeat the same risk management ideas (e.g., resource sharing and stakeholder concerns) in multiple sections without adding value.arrow_forwardBased on the U.S. Department of Transporation's publication on the number of inrternatioal passengers that come through New York airport (JFK) in 2012, how would I estimate the passenger volume for the coming year?arrow_forwardWhat are the role of trends and seasonality based on the Department of Transportation publication of the number of international passengers that come through New York (JFK) in 2012?arrow_forward
- MarketingMarketingISBN:9780357033791Author:Pride, William MPublisher:South Western Educational PublishingContemporary MarketingMarketingISBN:9780357033777Author:Louis E. Boone, David L. KurtzPublisher:Cengage LearningPractical Management ScienceOperations ManagementISBN:9781337406659Author:WINSTON, Wayne L.Publisher:Cengage,
- Purchasing and Supply Chain ManagementOperations ManagementISBN:9781285869681Author:Robert M. Monczka, Robert B. Handfield, Larry C. Giunipero, James L. PattersonPublisher:Cengage Learning
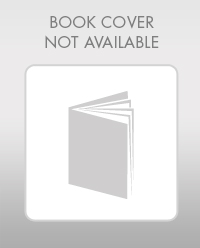
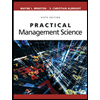
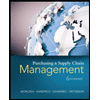