Machine Learning is the science of learning from experience. Suppose Alice is repeatedly doing an experiment. In each experiment, she tosses n coins. She does this experiment m times. In the first round, x1 coins yielded a head and y1 coins yielded a tail. Notice that, x1 + y1 = n. In the second round, x2 coins yielded a head and y2 coins yielded a tail. Once again, x2 + y2 = n. She does this experiment m times. Your job is to estimate the probability p of a coin yielding a head. 1. What is your guess on the value of p? 2. In Maximum Likelihood Estimation, we want to find a parameter p that maximizes all the observations in the dataset. If the dataset is a matrix A, where each row a1, a2, · · · , am are individual observations, we want to maximize P(A) = P(a1)P(a2)· · · P(am) because individual experiments are independent. Maximizing this is equivalent to maximizing log P(A) = log P(a1) + log P(a2) +· · ·+ log P(am). Maximizing this quantity is equivalent to minimizing the − log P(A) = − log P(a1) − log P(a2) − · · · − log P(am). 3. Here you need to find out P(ai) for yourself.
Please provide step-by-step solution for the following:
Machine Learning is the science of learning from experience. Suppose Alice is repeatedly doing an
experiment. In each experiment, she tosses n coins. She does this experiment m times. In the first
round, x1 coins yielded a head and y1 coins yielded a tail. Notice that, x1 + y1 = n. In the second
round, x2 coins yielded a head and y2 coins yielded a tail. Once again, x2 + y2 = n. She does this
experiment m times. Your job is to estimate the probability p of a coin yielding a head.
1. What is your guess on the value of p?
2. In Maximum Likelihood Estimation, we want to find a parameter p that maximizes all the
observations in the dataset. If the dataset is a matrix A, where each row a1, a2, · · · , am are
individual observations, we want to maximize P(A) = P(a1)P(a2)· · · P(am) because individual experiments are independent. Maximizing this is equivalent to maximizing log P(A) =
log P(a1) + log P(a2) +· · ·+ log P(am). Maximizing this quantity is equivalent to minimizing the
− log P(A) = − log P(a1) − log P(a2) − · · · − log P(am).
3. Here you need to find out P(ai) for yourself.


Step by step
Solved in 3 steps with 33 images

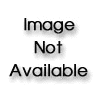
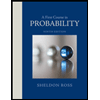
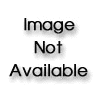
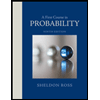