ld Price ($ thousands) SqFt 1 153000 920 1 0 33 Yes Baths Bedrooms Garage Age of Property Lot Size Property Condition Basement Outdoor Amenities School Quality Crime Rate Proximity to Amenities Transportation Accessibility 3280 Poor Property Tax Rate None 6 1.83 3.5 No 186 2 163150 750 1 2 0 38 2772 Poor No None 9 2.34 3.9 Yes 104 3 164900 900 1 2 0 13 3530 Excellent Yes None 6 2.98 3.9 No 2.11 4 170175 1500 т 2 0 31 5561 Fair Yes Deck 7 2.49 4.8 No 184 5 176900 1030 2 2 0 47 3247 Excellent No None 3 2.82 4.3 No 1.95 6 187700 1090 2 0 36 3898 Pocr No Garden 8 2.68 4 No 15 7 295000 1280 2 2 1 45 4617 Excellent Yes None 9 2.66 5 No 2.22 8 251650 1365 1 2 1 24 4674 Good No Garden 8 2.69 3.8 No 2.32 9 472990 1690 3 3 1 15 6315 Fair Yes Deck g 1.2 4 No 15 10 473000 1765 3 3 1 29 6944 Fair Yes Deck 9 1.74 4.2 No 1.88 11 773650 2780 3.5 4 2 8 10913 Fair Yes Deck 8 1 1.6 No 1.63 674000 1910 3 3 2 5 6591 Poor Yes Garden 6 1 2.8 No 1.95 13 885000 1920 4 4 2 29 5977 Fair No Deck 7 1 1.8 No 2.19 14 780000 1930 4 4 2 47 7691 Good Yes Garden 8 1 2.1 Yes 23 15 385900 950 1.5 3 1 4 3647 Poor Yes Deck 6 1.94 4 No 134 16 284650 850 1 2 1 12 2656 Fair No None 5 1.94 3.7 No 1.09 17 189500 950 2 1 18 392800 1025 2 2 19 299600 1560 1.5 2 20 115350 980 1.5 1 21 120170 750 1.5 1 22 164900 840 1 1 23 574900 2180 2.5 3 24 970000 3400 3.5 4 25 810000 2790 3 4 26 840000 2860 3 4 27 185500 1350 1 1 28 289600 1100 1.5 2 OHHOOOHMNNOO 0 45 3725 Fair No None 7 2.93 4.1 Yes 1.93 1 2 3967 Excellent No Garden 6 1.65 3.6 No 1.66 1 27 5489 Excellent No Pool 7 1.77 3.8 Yes 197 0 31 3519 Fair No Deck 7 3.06 4 Yes 1.19 0 36 2549 Good No None 6 1.9 5 No 1.63 0 36 2566 Poor No None 6 2.7 5 Yes 15 1 26 7271 Good Yes Garden 6 1 3.3 No 2.27 3 43 12930 Fair Yes Garden 9 1 1.6 No 2.94 2 27 8383 Good Yes Garden 8 1 1.9 No 2.79 2 5 9534 Excellent No Deck 9 1 1.8 Yes 28 0 20 4588 Good Yes None 6 2.59 5 Yes 2.02 0 11 3891 Fair No Pool 6 1.07 4.5 No 1.93 29 389800 1250 2 3 1 10 4900 Fair Yes None 8 1.38 3.3 No 2.29 30 689500 1870 3 3 1 40 6258 Good Yes Garden 9 1.22 2.8 No 19 31 889100 2850 3.5 4 2 38 9539 Good Yes Garden 7 1 1.9 Yes 2.15 32 283700 985 1.5 2 33 160450 980 1 1 34 195989 1100 1.5 1 35 999900 3250 4 4 36 225340 1150 2 2 COONO 0 6 3681 Good No None 6 2.2 4.3 No 1.09 0 8 3383 Poor Yes None 8 1.79 4.7 No 2.08 0 23 3547 Fair No Garden 8 2.64 4.9 No 1.12 2 47 11220 Good No Garden 8 1 1.2 Yes 2.21 0 26 3612 Good No None 7 2.27 4.3 No 1.22 37 125750 950 1.5 1 0 46 3018 Good No Deck 6 3.19 3.7 No 2.14 38 124700 890 2 1 0 43 3114 Fair No None 3 2.24 4 No 2.18 39 200500 1200 2 2 1 12 4103 Fair Yes Deck 9 2.08 4.8 Yes 1.72 40 128500 980 1 1 0 26 3837 Poor Yes None 8 1.33 4.1 No 2.1 41 174360 1100 1.5 1 1 13 3699 Excellent No Garden 7 2.88 4 No 1.37 42 179800 1210 2 1 0 40 4333 Poor Yes Deck 7 2.56 4.4 Yes 2.15 43 205450 1350 2.5 2 1 18 4904 Fair Yes Garden 7 1.47 4.7 No 169 44 779800 2600 3 4 2 25 7834 Excellent Yes Garden 9 1 1.9 No 199 45 128800 985 1.5 1 0 33 3609 Fair No None 8 2.13 2.8 Yes 1.11 46 522200 2345 3 3 1 47 7452 Fair No Pool 6 1.66 2.8 Yes 1.77 47 1173200 3250 3.5 5 2 40 12873 Poor No Deck 9 1 1.7 No 1.59 48 1824200 3875 4 5 3 43 12201 Good Yes Garden 8 1 1 Yes 2.91 49 2475200 4560 5 6 3 12 15571 Good No Garden 8 1 1 No 3.78 50 3126200 5870 5.5 7 4 44 18111 Fair Yes Pool 9 1 1 No 2
You must use Excel to perform the regression analysis. Provide the answers under the space provided for each question. You must provide the Excel output for the question along with the answers. Round off the values on the output to three-five decimal places if appropriate.
Examine the interaction effect between Lot Size and Outdoor Amenities (deck or garden) on house price in a multiple regression model.
Questions:
1. Include an interaction term for Lot Size × Outdoor Amenities in the regression model, along with the individual terms for Lot Size and Outdoor Amenities. (3p)
2. Analyze the p-value of the interaction term to determine if the interaction significantly contributes to predicting house price. (α = 0.05) (3p)
3. Interpret the coefficient of the interaction term to understand how the presence of outdoor amenities may add value to properties with larger lot sizes. (3p)
4. Write the corresponding regression equation substituting each coefficient and independent variables along with the interaction term. What is the predicted price for homes with a lot size of 5,000 square feet that include a garden?(4p)
Conduct a simple linear regression analysis for each independent variable associated with the external factors, using house price as the dependent variable. Make sure to use the indicator variables for the categorical data. (Significance level of α = 0.05). For each regression:
1. Report the p-value of the independent variable and indicate whether it is a significant predictor of house price (based on the p-value being less than 0.05). (3p)
2. Report the explained variability (R-squared value) for each variable, whether it is significant based on the p-value. (3p)
3. Identify and list any variables that are not significant predictors of house price (i.e., those with p-values greater than 0.05). (3p)
Conduct a multiple regression analysis using all significant external factors, such as School Quality, Crime Rate, Proximity to Amenities, Transportation Accessibility, and Property Tax Rate to predict house price.
1. Report the p-values for each external factor to assess their significance as predictors of house price. (3p)
2. Interpret the coefficients for each significant variable to understand the direction and strength of its relationship with house price. (3p)
3. Evaluate the overall model fit (e.g., R-squared value) to determine how well these external factors explain the variability in house price. Is the overall model statistically significant at .05 level of significance? (3p)
4. In a multiple regression model for house price using external factors, analyze any insignificant variables (those with p-values greater than 0.05). If any are identified, check multicollinearity and identify highly correlated variables. (3p)
List pairs of variables with high correlation coefficients and consider potential reasons for their correlation.
Based on the findings, consider approaches to address multicollinearity, such as removing highly correlated variables to improve the model and rerun the regression analysis.
1. Write the multiple regression equation by substituting each coefficient and significant external factor as independent variables into the model. Then, use this equation to make a prediction for house price based on the following values for the external factors: School Quality:9, Crime Rate:1, Property Tax Rate: 2.5. (5p)


Step by step
Solved in 2 steps

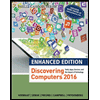
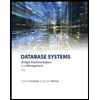
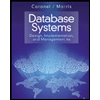
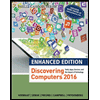
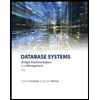
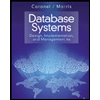
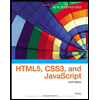
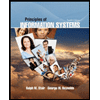