Individual Assignment 2 2023 (1)
docx
keyboard_arrow_up
School
Multimedia University of Kenya *
*We aren’t endorsed by this school
Course
MANAGERIAL
Subject
Business
Date
Nov 24, 2024
Type
docx
Pages
12
Uploaded by mongaresams
Assessment (non-exam) Brief
Module code/name
MSIN0093: Business Strategy and Analytics
Module leader name
Dr. Anil Doshi
Academic year
2023/24
Term
Assessment title
Individual Assignment 2
Individual/group
assessment
Submission deadlines:
Students should submit all work by the published deadline date and time. Students
experiencing sudden or unexpected events beyond your control which impact your ability to complete assessed work
by the set deadlines may request mitigation via the
extenuating circumstances procedure
. Students with disabilities
or ongoing, long-term conditions should explore a
Summary of Reasonable Adjustments
.
Return and status of marked assessments:
Students should expect to receive feedback within one calendar month of
the submission deadline, as per UCL guidelines. The module team will update you if there are delays through
unforeseen circumstances (e.g. ill health). All results when first published are provisional until confirmed by the
Examination Board.
Copyright Note to students:
Copyright of this assessment brief is with UCL and the module leader(s) named above. If
this brief draws upon work by third parties (e.g. Case Study publishers) such third parties also hold copyright. It must
not be copied, reproduced, transferred, distributed, leased, licensed or shared with any other individual(s) and/or
organisations, including web-based organisations, without permission of the copyright holder(s) at any point in time.
Academic Misconduct:
Academic Misconduct is defined as any action or attempted action that may result in a
student obtaining an unfair academic advantage.
Academic misconduct includes plagiarism, obtaining help
from/sharing work with others be they individuals and/or organisations or any other form of cheating.
Refer to
Academic Manual Chapter 6, Section 9: Student Academic Misconduct Procedure - 9.2 Definitions
.
Referencing:
You must reference and provide full citation for ALL sources used, including AI sources, articles, text
books, lecture slides and module materials.
This includes any direct quotes and paraphrased text.
If in doubt,
reference it.
If you need further guidance on referencing please see
UCL’s referencing tutorial for students
. Failure to
cite references correctly may result in your work being referred to the Academic Misconduct Panel.
Use of Artificial Intelligence (AI) Tools in your Assessment:
Your module leader will explain to you if and how AI tools
can be used to support your assessment. In some assessments, the use of generative AI is
not permitted
at all. In
others, AI may be used in an
assistive
role which means students are permitted to use AI tools to support the
development of specific skills required for the assessment as specified by the module leader. In others, the use of AI
tools may be an
integral
component of the assessment; in these cases the assessment will provide an opportunity to
demonstrate effective and responsible use of AI. See page 3 of this brief to check which category use of AI falls into
for this assessment. Students should refer to the
UCL guidance on acknowledging use of AI and referencing AI
. Failure
to correctly reference use of AI in assessments may result in students being reported via the Academic Misconduct
procedure. Refer to the section of the UCL Assessment success guide on
Engaging with AI in your education and
assessment
.
For staff reference only: template version 1.0 September 2023
Content of this assessment brief
Section
Content
A
Core information
B
Coursework brief and requirements
C
Module learning outcomes covered in this assessment
D
Groupwork instructions (if applicable)
E
How your work is assessed
F
Additional information
Section A: Core information
Submission date
Submission time
2:00PM
Assessment is marked out of:
100
% weighting of this assessment
within total module mark
40%
Maximum word count/page
length/duration
2,000 words (write word count on title page). Guidance on length
of responses for each question is provided. The word count
requirement is for the overall submission. Word counts can be
greater than or less than guidance of individual problems as long
as total word count is less than 2,000 words.
Footnotes, appendices, tables,
figures, diagrams, charts included
in/excluded from word count/page
length?
Excluded
Bibliographies, reference lists
included in/excluded from word
count/page length?
Excluded
Penalty for exceeding word
count/page length
Penalty for late submission
Standard UCL penalties apply. Students should refer to
https://www.ucl.ac.uk/academic-manual/chapters/chapter-4-
assessment-framework-taught-programmes/section-3-module-
assessment#3.12
Artificial Intelligence (AI) category
Submitting your assessment
Submit a single document (PDFs strongly preferred) to the
Moodle assignment submission inbox.
Anonymity of identity. Normally, all
submissions are anonymous unless
the nature of the submission is such
that anonymity is not appropriate,
illustratively as in presentations or
where minutes of group meetings
are required as part of a group work
submission
Your preview ends here
Eager to read complete document? Join bartleby learn and gain access to the full version
- Access to all documents
- Unlimited textbook solutions
- 24/7 expert homework help
Section B: Assessment Brief and Requirements
Please complete each problem in the assignment. Any additional criteria specific to this assessment are
detailed in section F.
1.
[300 words, Week 4,5] IdeaWeb follow-up analysis.
Read the IdeaWeb (C) case attached (ignore the
case questions). Say Duran showed you these two regressions. State three interesting observations from
the regression results. For each observation, refer to one or more specific coefficients. You do not need to
analyse every coefficient in the regressions. The idea of this question is for you to explain what you see as
the most interesting or important results.
2.
[700 words, Week 6] Experiment proposal.
You have joined the data science team of the company of
your dreams. (Choose a well-known firm such as McDonald's or Alibaba. Indicate the company in the first
sentence of your answer.) The head of the data science team gives you your first assignment: “At our
company, we depend on experimentation to find ways to improve performance. I would like you to
propose and design an experiment to test some strategic aspect of our business. Your proposal can be
related to the company's user interface, products, customer experience, or another area of the company.”
Draft a proposal for an experiment. (You do not need to run the experiment and you do not need any data
for this problem.)
Your response should include the following elements:
a.
Identification of the aspect of the business or operations that you would like to test and its
strategic relevance.
b.
A description of the overall evaluation criterion (OEC) you will use and a brief justification of the
choice.
c.
A description of the current implementation (i.e., the control) and your proposed intervention (i.e.,
the treatment). Ensure that your proposed intervention is clearly described. You are encouraged to
be creative in your description (verbal descriptions, sketches, screenshots, etc. are all acceptable).
Design your experiment with only one treatment.
d.
What is the population of interest? Describe how you would randomly assign subjects of that
population into the treatment and control.
e.
The null and alternative hypotheses.
f.
What are the policy implications for your company if the null hypothesis is rejected? What are the
implications if the null hypothesis is not rejected?
3.
[300 words, Week 7]. Causal diagrams in real estate.
You work as a data analyst at a real estate
company. Management has asked you to assess whether improvements in landscaping cause increases in
home values. You decide to sketch a causal diagram. Your causal diagram includes the following variables:
landscaping quality (independent variable of interest)
home value (dependent variable of interest)
house age
lot size
outdoor space functionality (how the outdoor space can be used)
a.
[Image] Assume you run the following regression to show the causal effect of “landscaping
quality” on “home value:”
lot
¿¿
value
=
β
0
+
β
1
(
landscapingquality
)
+
β
2
(
year built
)+
β
3
(
outdoor space functionality
)+
β
4
¿
Neatly sketch the causal diagram that implies this regression identifies the causal relationship of
interest.
b.
[1-2 sentences] Does your causal diagram include an arrow from “lot size” to “outdoor space
functionality”? Why or why not?
c.
[1-2 sentences] Does your causal diagram include an arrow from “outdoor space functionality” to
“lot size”? Why or why not?
d.
[1-2 sentences] Propose one way you might measure “landscaping quality.”
e.
[Brief paragraph] Even if your causal diagram is correct, state two reasons why your regression
might not estimate the true causal relationship of “landscaping quality” on “home value?”
4.
[450 words, Week 8, 9]
You work at a company called Beto Enterprises, which operates call centres. Call
agents at Beto take customer service calls on behalf of client firms and troubleshoot the problems that
customers are facing. The company has call centres around the world, with locations in Austin (USA),
Bangalore (India), Bucharest (Romania), and Buenos Aires (Argentina).
Recently, the company purchased and deployed new software for agents to use during calls in the Austin
location (there are 924 agents in the Austin office). The new software is meant to provide a streamlined
interface for agents designed to reduce the amount of time required to search for solutions. Management
expects that the new software will speed up the average time an agent spends on a call, a key
performance indicator used to measure agent productivity.
Due to a technical error that arose when purchasing the software licenses, the software was not rolled out
to every agent at the same time. Specifically, when the software was first rolled out to agents' desktops,
only 491 agents had access to the new software; the remaining 433 continued to use the old software.
Four weeks later, the new software was installed on the remaining machines and all agents in Austin were
running the new software.
You are the chief data scientist at Beto. Management has asked you to measure the impact of the software
on the average time spent on calls. You collect a weekly dataset of the average time that agents spent on
calls for four weeks prior to and after the
initial
deployment. (Note: There is no dataset that you need to
download to answer this problem. The results and any information you might need are contained in this
problem.) A description of the variables in the dataset can be found in Table 1.
One of your data analysts takes the data and runs the regression found in Column 1 of Table 2. Your
analyst claims, “I wanted to focus my analysis on the 491 agents who received the software in the first roll
out. The coefficient on the
got software
variable is –2.018 and it is statistically significant. So, the software
saved about 2 minutes per call, on average.”
You use the data and run your own regression, including all the agents in the Austin office. That regression
is shown in Column 2.
a.
[1-2 sentences] Why is your analyst's work (presented in Column 1) invalid?
b.
[1-2 sentences] Looking at your regression in Column 2, how would you report the impact of the
new software to management?
c.
[1 sentence] Why was the
female
variable dropped from both regressions?
d.
[1-2 sentences] What is the role of the agent fixed effects in the regressions?
e.
[1-2 sentences] What is the role of the week dummies in the regressions?
f.
[1-2 sentences] Why is difference-in-differences more appropriate than synthetic controls to
analyse this dataset?
g.
[1-2 sentences] The analysis was conducted in the Austin office. Why might the improvement not
Your preview ends here
Eager to read complete document? Join bartleby learn and gain access to the full version
- Access to all documents
- Unlimited textbook solutions
- 24/7 expert homework help
be the same for the other regional offices?
Table 1: Software Roll Out Variable Descriptions
Variable
Description
agent id
an anonymized agent identifier
avg timeoncall
the average number of minutes spent on calls during the week
got software
=1 if the agent was using the new software that week, =0 otherwise
weeknum
the week of the data period (ranges from -3 to 4; the software rolled out between
weeks 0 and 1)
mentor hours
number of hours spent mentoring other agents in the prior week
Female
=1 if agent identifies as female, =0 otherwise
Table 2: Regression Results
DV: avg timeoncall
(1)
(2)
got software
–2.018***
–1.518***
(0.059)
(0.007)
mentor hours
–0.105***
–0.095***
(0.003)
(0.011)
female
[dropped]
[dropped]
constant
16.937***
17.426***
(1.669)
(1.845)
Agent fixed effects?
Yes
Yes
Week dummies included?
Yes
Yes
Agent-weeks (num obs)
3928
7392
Number of agents
491
924
F-Stat
69.7
74.2
Adj R-squared
0.44
0.48
5.
[250 words, Week 10]. Data ethics.
Answer the following questions about different types of data ethical
considerations:
a.
[1-2 sentences] Why might bias in sampling lead to ethical concerns?
b.
[1-2 sentences] Why might bias in variables perpetuate historical outcomes?
c.
[1-2 sentences] Why might collecting data for one purpose and using it for a different purpose lead
to ethical concerns?
Section C: Module Learning Outcomes covered in this
Assessment
This assessment contributes towards the achievement of the following stated module Learning
Outcomes as highlighted below:
Apply economic principles to incorporate data and data analytics into a company's value
proposition
Consider the ethical implications of data applications
Design surveys and experiments and analyze the resulting data
Apply methods on observational data to arrive at causal inferences
Section D: Groupwork Instructions (where
relevant/appropriate)
na
Your preview ends here
Eager to read complete document? Join bartleby learn and gain access to the full version
- Access to all documents
- Unlimited textbook solutions
- 24/7 expert homework help
Section E: How your work is assessed
Within each section of this assessment you may be assessed on the following aspects, as applicable and
appropriate to this assessment, and should thus consider these aspects when fulfilling the requirements of
each section:
The accuracy of any calculations required.
The strengths and quality of your overall analysis and evaluation;
Appropriate use of relevant theoretical models, concepts and frameworks;
The rationale and evidence that you provide in support of your arguments;
The credibility and viability of the evidenced conclusions/recommendations/plans of action you
put forward;
Structure and coherence of your considerations and reports;
Appropriate and relevant use of, as and where relevant and appropriate, real world examples,
academic materials and referenced sources. Any references should use either the Harvard OR
Vancouver referencing system (see
References, Citations and Avoiding Plagiarism
)
Academic judgement regarding the blend of scope, thrust and communication of ideas,
contentions, evidence, knowledge, arguments, conclusions.
Each assessment requirement(s) has allocated marks/weightings.
Student submissions are reviewed/scrutinised by an internal assessor and are available to an External
Examiner for further review/scrutiny before consideration by the relevant Examination Board.
It is not uncommon for some students to feel that their submissions deserve higher marks (irrespective of
whether they actually deserve higher marks). To help you assess the relative strengths and weaknesses of
your submission please refer to
SOM
Assessment Criteria Guidelines
, located on the Assessment tab of the
SOM Student Information Centre Moodle site.
The above is an important link as it specifies the criteria for attaining the pass/fail bandings shown below:
At UG Levels 4, 5 and 6:
80% to 100%: Outstanding Pass - 1st;
70% to 79%: Excellent Pass - 1st; 60%-69%: Very Good Pass - 2.1;
50% to 59%: Good Pass - 2.2; 40% to 49%:
Satisfactory Pass - 3rd; 20% to 39%:
Insufficient to Pass -
Fail;
0% to 19%:
Poor and Insufficient to Pass - Fail.
At PG Level 7:
86% to 100%: Outstanding Pass - Distinction;
70% to 85%: Excellent Pass - Distinction; 60%-69%: Good Pass
- Merit; 50% to 59%: Satisfactory - Pass; 40% to 49%:
Insufficient to Pass - Fail;
0% to 39%:
Poor and
Insufficient to Pass - Fail.
You are strongly advised to review these criteria before you start your work and during your work, and
before you submit.
You are strongly advised to
not
compare your mark with marks of other submissions from your student
colleagues. Each submission has its own range of characteristics which differ from others in terms of
breadth, scope, depth, insights, and subtleties and nuances. On the surface one submission may appear to
be similar to another but invariably, digging beneath the surface reveals a range of differing characteristics.
Students who wish to request a review of a decision made by the Board of Examiners should refer to
the
UCL Academic Appeals Procedure
, taking note of the
acceptable grounds
for such appeals.
Note that the purpose of this procedure is not to dispute academic judgement – it is to ensure correct
application of UCL’s regulations and procedures. The appeals process is evidence-based and circumstances
must be supported by independent evidence.
Section F: Additional information from module leader
(as appropriate)
• Please see the
rubric
for the module for the categories used to assess the submission. While comments are
provided for each question, the submission is assessed as a whole.
• All written responses must be written in paragraph format with complete sentences. Slides, outlines, or notes
will be penalized. (The irony of my writing this point as part of a bulleted list is not lost on me.)
• I highly recommend that you proofread your assignment before submitting it.
• Do not include code in the text of the report.
• Do not over-reach in your conclusions.
• Take care to properly design any tables and graphs. Maintain consistency within and across tables and graphs
(title in the same place, fonts, labels, colors, etc.). Make sure they have a title and some way to refer to them in
the text (e.g., Figure 1).
Each visualization should be referred to in the text, but each should also be interpretable
without looking at the text.
Your preview ends here
Eager to read complete document? Join bartleby learn and gain access to the full version
- Access to all documents
- Unlimited textbook solutions
- 24/7 expert homework help
Related Documents
Recommended textbooks for you
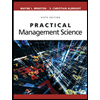
Practical Management Science
Operations Management
ISBN:9781337406659
Author:WINSTON, Wayne L.
Publisher:Cengage,
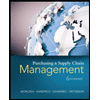
Purchasing and Supply Chain Management
Operations Management
ISBN:9781285869681
Author:Robert M. Monczka, Robert B. Handfield, Larry C. Giunipero, James L. Patterson
Publisher:Cengage Learning
Recommended textbooks for you
- Practical Management ScienceOperations ManagementISBN:9781337406659Author:WINSTON, Wayne L.Publisher:Cengage,Purchasing and Supply Chain ManagementOperations ManagementISBN:9781285869681Author:Robert M. Monczka, Robert B. Handfield, Larry C. Giunipero, James L. PattersonPublisher:Cengage Learning
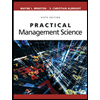
Practical Management Science
Operations Management
ISBN:9781337406659
Author:WINSTON, Wayne L.
Publisher:Cengage,
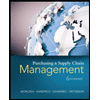
Purchasing and Supply Chain Management
Operations Management
ISBN:9781285869681
Author:Robert M. Monczka, Robert B. Handfield, Larry C. Giunipero, James L. Patterson
Publisher:Cengage Learning