Primary Production
docx
keyboard_arrow_up
School
University of Minnesota-Twin Cities *
*We aren’t endorsed by this school
Course
1961
Subject
Biology
Date
Feb 20, 2024
Type
docx
Pages
16
Uploaded by ProfessorEnergyKangaroo34
Primary Production
Analyzing Primary Production Across Space & Time
Connections
●
This activity relates to the Global Change Ecology research area in our foundations lab courses.
●
You will make graphs in this activity, so reflect back on what you’ve learned so far from the zebrafish/caffeine figure-making activity. Representing your data graphically is an important
skill to develop in this field.
●
This activity is connected to research happening at the U of MN Cedar Creek Ecosystem Science Reserve
, which is a field station not far from our campus.
Goals/Skills
After completing this lab activity you will:
●
Understand the source of primary productivity data.
●
Have experience creating graphs to show the relationship between primary production and nutrient availability.
●
Be able to describe patterns in primary production over space and time.
●
Be able to compare and contrast primary production in aquatic and terrestrial ecosystems.
●
Understand when, why, and how to carry out a t-test.
Make your own copy of this document
Remember to make a copy of this document (File>Make a copy), or download a copy (File>Download) to use as your working document. Introduction
Ecological questions require quantitative data. How researchers gather those data vary from project to project, but one of the important aspects of ecological research is figuring out how to quantify what is happening ecologically. Primary production
is the conversion of solar energy into
chemical energy stored in the bonds of reduced sugars and represents the base of the energy pyramid in an ecosystem. Facilitated by photosynthetic organisms, primary production can be quantified by measuring the accumulation of photosynthetic biomass over a period of time.
In this activity, you will explore datasets on end-of-season biomass harvested from plots at Cedar Creek and other field sites. Biomass measurements give an indication of the net primary productivity of an area (the amount of carbon fixed minus the amount of carbon used in respiration). The primary productivity of an ecosystem is dependent on the availability of factors that limit plant growth. Limiting factors for productivity can include sunlight, water, and nutrients. At the Cedar Creek station, and at other sites around the world, there are experiments set up to understand how nutrient input affects grassland ecosystems. The researchers conducting these experiments have joined together into a Nutrient Network or NutNet. You can read more about NutNet here (
https://nutnet.umn.edu/
). In short, this global experimental network is observing the effects of nutrient addition and herbivores on grasslands.
JMP Software
This lab activity introduces a new statistical software option you can use for data analysis and graphing. The university has a license for JMP Pro - it is free to all students and staff. You may have already downloaded the software as part of your pre-class work, if you want to work on your personal computer during lab (rather than one of our lab Macbooks). If you still need to download JMP, go to this page for instructions and download links
. Select the student version, and the version that goes with your operating system. If you have trouble getting JMP onto your computer, you can try using the Windows Terminal Services (see the special instructions for Chromebook users below, and choose the instructions appropriate for your computer). You also have the option of doing this lab activity on the Macbooks available in our lab rooms.
Special instructions for Chromebook users:
You are able to access JMP (and other software) via the Windows Terminal Services at the U of MN.
If you’re on campus and connected to the university’s network, follow the instructions here
to use the Windows Terminal Services (you want the instruction for Android OS/Chrome OS).
If you’re off campus, you’ll need to start by installing, configuring, and connecting to the U of
MN’s VPN. If you already have Cisco AnyConnect and are able to get on the VPN you can skip this step. Instructions are here
. Once the VPN is set up, you can go to the Windows Terminal Services instructions
(choose the instructions for Android OS/Chrome OS).
Once you’ve installed JMP, the first time you open it you may get a popup window saying the license is expired
. This issue is addressed on the site linked above. If you get that message, go back to the site linked above and select the student 2021-2022 JMP license files link. This will download a zipped file. Unzip that file. Go back to the popup message in JMP, click the link to open the license file, navigate to the unzipped folder, and choose the file inside the folder that has the highest version number (each file is named JMP### - pick the highest number). Click “open”, and JMP should now see that you have an up to date license via the university. If this doesn’t work,
check which version of JMP you’ve downloaded, and pick the file with the matching version number.
Part 1: Analyzing biomass data from Cedar Creek
In the initial year of a field experiment, it is important to verify that you have standard starting conditions across your field sites. In order to examine the impact of fertilizers on grassland productivity, we must first establish that all biomass production at each of the study plots is the same. Specifically, we’ll use the data collected by students in fall 2018, which was year zero in the Nutrient Network Education (NutNEd) plots at Cedar Creek. We want to compare the living biomass measured in the control plots and fertilized plots. Following this, we’ll compare the living biomass measured in the control and fertilized plots a few years later (year three, 2021). A t-test can be performed to determine if there is a significant difference in a given measurement between two groups. To perform a t-test, one first calculates the value of the t-statistic, which is then interpreted to determine a p-value.
Sample Distributions
Before you begin your t-tests with the NutNEd data, let’s think about sample distributions and their relationship to statistical tests. Below are four graphs, each containing two sample distributions. The distribution in gray is from samples that were treated with Compound X, and the distribution in red is from control samples. Using your knowledge of distributions and summary statistics, rank these four graphs based on how confident you would be that Compound X has an effect (1 = most confident, 4 = least confident), and write out your reasoning. Wait for your lab instructor before moving on to the next section.
Your ranking:
Your reasoning:
What is a t-statistic?
Below is the formula to calculate
a t-statistic.
Describe, as best as you can in a
short phrase, what each boxed
quantity represents (wait for your
lab instructor before moving to
the section after this).
Your preview ends here
Eager to read complete document? Join bartleby learn and gain access to the full version
- Access to all documents
- Unlimited textbook solutions
- 24/7 expert homework help
A:
B:
C:
D:
Below is data from an experiment testing the effect of radiation on the growth of a bacteria species.
Using the two sample distributions below, predict the size (small or large) of the t-statistic and p-
value that would result from a t-test. Highlight your choices below the graph, and explain your reasoning. Wait for your lab instructor before moving to the next section.
The t-statistic will be: Large
Small
The p-value will be:
Large
Small
Statistical conclusion:
Significant
Not Significant
Explain your reasoning here:
Now, assume you ran a second experiment using the same bacteria species. This time you were testing the effect of temperature on its growth, and you found a p-value of 0.07. Describe how you would interpret this result. Wait for your lab instructor before moving on to the next section.
T-test on year zero NutNEd data - Procedure
Remember that our first goal is to run a t-test to determine whether or not there was a significant difference between the control plots and fertilized plots in year zero at the NutNEd plots (year zero being the year the plots were first established). The fertilized plots were treated with nitrogen, phosphorus, and potassium. This set of nutrients is labeled “NPK” in the NutNEd data. To make things a bit more streamlined for you, we’ve already subsetted this dataset so that it only includes the biomass measurements from the control plots and NPK plots from year zero in NutNEd. Remember that a t-test tests for differences between two groups. In this case, we do not expect a significant difference between the two groups, so we want to test for a difference in either direction (i.e., test whether the NPK group has either higher or lower biomass than the control group). Why do we expect that there would be no difference between the control and treatment groups? If you’re not sure, revisit the paragraph at the beginning of this section (“Analyzing biomass data…”).
1.
Download the necessary data file to your computer. The file you need is “Dataset 1 NutNEd
2018”, which is on this week’s Canvas page. This file contains the biomass data for the NutNEd plots at Cedar Creek that was collected by students in this course in Fall of 2018.
○
Do not use Safari browser to download the data files for this lab activity. And if you’re using a Mac, do not open the data file in the Numbers app after downloading (for some reason this modifies the file and it will not open in JMP).
2.
Open JMP
3.
To open the data file in JMP, from the JMP Starter window, select “File” in the pane on the left side of the window, then under “Data Table” choose “Open”. You can also use the “File”
menu at the top of the screen and select “Open”. Navigate to the “Dataset 1 NutNEd 2018” file you downloaded, and select it.
4.
Now you have the file opened as a JMP table. Your table should contain 4 columns:
○
site_name
○
year_trt
○
trt
○
Live_mass
5.
JMP is pretty good at determining what type of data is in each column of your table (numeric data, category names, etc.). Sometimes JMP gets it wrong, though, so it’s always good to check. Look at the “Columns” header to the left of your data table. Here, you’ll see a list of your column names, and each one has a little symbol next to it. The red bar graph symbol is for “nominal” data - these are names, categories, etc. The blue triangle symbol is for “continuous” data - this is numeric data. ○
Check that site_name
and trt are both nominal (red bar graph symbol)
○
Check that year_trt
and live_mass
are both continuous (blue triangle symbol)
○
If you need to change any of these, click on the symbol and choose the correct category instead.
○
You can find more information about JMP’s data types here
if you’re interested
6.
Under the “analyze” menu, select the “fit Y by X” option. This will launch the fit Y by X window.
7.
The goal is now to make a plot with the treatment (control or NPK) as the independent variable on the x-axis and the live_mass (the biomass of plant material) as the dependent variable on the y-axis. Therefore, the “trt” variable is the X factor and the “live_mass” is the Y response. Drag each of these variables from the list on the left side into the X and Y factor boxes. 8.
Click “OK” to run the analysis. A window with your plot will open.
9.
In the upper-left corner of the plot (near the plot’s header), there is a small red triangle that allows you to run various statistical tests. Click the arrow and select “t-test” from the dropdown menu.
10. The t-test analysis table should open below your plot. There are several things listed in this table, but the thing we are looking for is called the p-value. This value is located next to the label that says “Prob>|t|”. This is the p-value for the two-sided t-test, when you are testing for a difference in either direction (i.e., NPK could be higher or lower than the control, rather
than expecting it to be different in only one direction). It is common practice for scientists to use a p-value of 0.05 as the cutoff value for determining statistical significance. If the p-
value of a t-test is greater than 0.05 there is not enough evidence for you to conclude that the difference between your two groups is a real one. In other words, if the p-value is greater than 0.05 any difference seen between the means of the two groups is likely due to chance. Write down the p-value listed in your table. What conclusion would you draw about the difference in live biomass between the control and treatment plots in year zero?
T-test on year three NutNEd data Now we want to test for a difference between the NPK and control plots in year three (2021). Let’s assume we’re hypothesizing that there is
a difference between the fertilized plots and control plots.
Do we expect that the fertilized plots will have more biomass because of the added nutrients? Is it possible that you can have too much of a good thing and the fertilizer application may have
Your preview ends here
Eager to read complete document? Join bartleby learn and gain access to the full version
- Access to all documents
- Unlimited textbook solutions
- 24/7 expert homework help
reduced total plant biomass over time? Think about what your hypothesis would be. Because different students may have different hypotheses here, we’re going to approach this t-test as though we don’t have a set expectation of the difference being in one direction or the other (which of the two groups would have the higher biomass values). We just want to see if there’s a significant difference between the two groups in either direction, like with the year zero data. We’ll explain, though, which p-values you would look at in the JMP t-test output if you wanted to test for a difference in one direction.
1.
Download the necessary data file to your computer: The file you need is “Dataset 2 NutNEd
2021”, which is on this week’s Canvas page. This file contains the biomass data for the NutNEd plots at Cedar Creek that was collected by students in this course in Fall of 2021.
2.
Follow steps 2-9 in the procedure for the year 0 t-test, then come back here for information about interpreting the output.
3.
Now that you have the t-test analysis table below your plot, note the p-value for the two-
sided t-test, which is “Prob>|t|”. If it is greater than 0.05, there is no significant difference between the two groups. If it is below 0.05, there is a significant difference between the two groups. 4.
If you are interested in how you would use this JMP output for a one-sided (or one-tailed) t-
test, note that at the top of your table, underneath the t-test header, it says “NPK-Control”. This tells you the order of the subtraction that JMP used. If you hypothesized that the NPK group’s biomass would be higher than the control group, you would look at the “Prob > t” value as the p-value of interest. If you hypothesized that the NPK group’s biomass would be
lower than the control group, you would look at the “Prob < t” value. What difference do you
notice between these two p-values in your t-test output table? Write down the “Prob > |t|” p-value listed in your table. What conclusion would you draw about the difference in live biomass between the control and treatment plots in year three?
Part 2: Graphing primary production across time & space
Graphical Representations of Data
In this section, you will graph primary production across time (at one NutNet site over several years) and across space (across sites at varying latitudes). Before you start making figures, your lab section will spend some time thinking about how to represent data graphically. Communicating your experimental results effectively is an important skill to have. Previously, you have practiced creating mathematical representations of your data. Here, you will develop your skills creating effective visual representations of your data.
In this lab, you have been provided data from the Nutrient Network, a global network of ecologists performing standardized experiments to better understand how fertilization impacts diversity and
productivity in grassland ecosystems. We are interested in exploring how primary production changes over time in response to the addition of nutrients.
Using the data from the above tables, draft a graph that shows how primary production changes over time. You can draft your graph on a piece of paper. Once you have a draft you are happy with, draw your graph on a piece of large chart paper provided by your lab instructor. Keep your chart paper at your bench until everyone is finished drawing and ready to move forward. Take a photo of your graph (to turn in with your post-lab assignment). Answer these questions:
Q1.What kind of graph did you create?
Q2.Why did you choose this type of graph? (Be prepared to share your reasoning with the class)
After everyone displays their graphs, compare your graph with the ones drawn by other groups. Describe the features of the graph that are similar and different between your graph and ones made by other groups. Note that we are not discussing data here, but the features of the graphs themselves.
Q3. What similarities and differences did you notice between your graph and the other students' graphs? List them below:
●
Similarities:
●
Differences:
Q4. After our class discussion, which type of graph do you think would be the best to use with these data? Why?
Q5. After our class discussion, what features should be on a graph to clearly communicate results? Primary Production Across Time
With one of the Cedar Creek NutNet datasets, you will use a simple linear model to answer the following questions: ●
How has primary production changed over time in grassland plots that have been fertilized with NPK?
●
How does this pattern compare to what happened in the control plots over time?
You will graph the annual primary production values for each year of the Cedar Creek NutNet experiment in both the control plots and the fully fertilized plots. You will use a simple linear regression to determine if productivity in these two plots has increased over time, and compare the
rate of increase in the fertilized plots to the rate of increase in the control plots.
Graphing - Procedure
1.
Download the necessary data file to your computer: The file you need is “Dataset 3 NutNet Cedar Creek”, which is on this week’s Canvas page. This file contains similar data to the file
you used for the t-test. However, these data are from the long-term NutNet plots (rather than our NutNEd plots used for education). So, while the column headers are the same you’ll notice that there are 10 years worth of data in this set.
2.
In JMP, choose “File”, and “Open”. Navigate to your saved file and select it.
3.
You’ll see a data table with 4 columns: ○
site_name
○
year_trt
○
trt
○
Live_mass
4.
Check the data type for each column in the “Columns” list at the right. They should be the same as the ones in the previous data set you worked with: “nominal” (red bar graph) for the site name and treatment columns, and continuous (blue triangle) for the year and mass columns.
5.
Graph the live mass (ANPP) vs. treatment year for the control and NPK plots, following these steps:
a.
Click the “graph” menu on the toolbar at the top of the screen, and open the graph builder.
Your preview ends here
Eager to read complete document? Join bartleby learn and gain access to the full version
- Access to all documents
- Unlimited textbook solutions
- 24/7 expert homework help
b.
In the graph builder window, drag the live_mass variable to the y-axis of your graph.
c.
Drag the year_trt variable to the x-axis.
d.
You want separate regression lines for the control and NPK plots. To achieve this, drag the trt variable into the overlay box on the right side of the window. This should separate your graph into two sets of dots with different colors, representing the two treatment types (control and NPK).
e.
Need different colors? If you need or want to change the colors of the graph, you can double-click on one of the legend items to the right of the graph (“Control” or “NPK”). In the window that opens, click on the bar next to “color theme” and choose something that works for you. If you want to get back to JMP’s default colors, choose the top bar under the “Qualitative” category of color themes.
f.
Now you’ll need to change the graph type to linear regression. The icons along the top of the graph builder window show you your graph type options. Select the icon that looks like a scatter plot with a straight diagonal line on it (it's called “Line of Fit” if you hover over it to see the name).
g.
Click “done” near the top of the graph builder window.
h.
In the window with your graph, you can click on the legend, axes, and other features
to customize them. Modify your figure by providing appropriate axis labels, legend labels (what red & blue represent), etc. Once you have a figure you are happy with, save it by going to File > Export, and export as an image file. You will include this graph as part of your post-lab assignment.
Analysis - Procedure
As a final step in this section on analyzing primary production across time, you will run some statistical tests. You will use the “Analyze” tab to test the significance of the slopes for each treatment group. In other words, we want to determine if there is statistical evidence that the slopes
of our two lines are significantly different from zero (a slope of zero is a flat line). Then you will compare our slope parameters to each other to determine whether or not the fertilized plots have a
significantly larger slope value than the control plots (i.e., a faster rate of biomass growth over time). There is actually a statistical test to determine if this difference in slopes is significant, and it is called an ANCOVA (Analysis of Covariance), but an ANCOVA is beyond the scope of this lab activity.
1.
Under the “analyze” menu, select the “fit Y by X” option. This will launch the fit Y by X window
2.
Add year_trt as the X factor and live_mass as the Y response. This will result in a simple linear model relating these two variables. We want to see if there is a difference between the control plots and treatment plots, so add the trt variable to the “By” option.
3.
Click OK to run the analysis, which will open the Bivariate relationship window.
4.
In the Bivariate relationship window, you should see two graphs: one for the control plots and one for the NPK plots.
5.
In the upper left corner of each plot, you should see a small red triangle. On the control plot,
click the red triangle and choose “Fit Line” to produce the line that best fits the data. This line should now show on your plot, and the linear fit information shows below the graph.
This linear fit section contains a lot of information, but for our purposes we are interested in the Analysis of Variance tab and the Parameter Estimates tab.
6.
The Analysis of Variance tab tells you if the slope of the line (the best fit line) is significantly different from zero. In the bottom right corner, there is a section labeled Prob>F. This is referred to as the p-value for this statistical test. If the p-value is less than 0.05, it means that the slope of the line is significantly different from zero. Record the p-value here. Is it significantly different from zero? 7.
Under the parameter estimates, you will see the slope and intercept estimates for the simple linear model describing your best fit line. The slope estimate is found in the year_trt row of the table. Record the estimate for your slope parameter. 8.
Repeat steps 5-7 for the NPK plot, and record the p-value, whether it’s significant, the slope
parameter, and your interpretation.
9.
Compare the slope estimates and the p-values from the two groups (control and NPK). What is your conclusion regarding the effect of NPK on biomass production?
Primary Production Across Space
In addition to understanding how productivity changes over time, the Nutrient Network experiment allows us to study how fertilization impacts primary productivity at different grassland sites across the planet. To start, you will compare the live biomass in fertilized and control plots at the original Nutrient Network sites that have now been running for 10 years. Then we will explore how this fertilization effect varies by latitude.
Graphing live biomass at multiple NutNet sites - Procedure
1.
Download the necessary data file to your computer: The file you need is “Dataset 4 NutNet Global Year 10”, which is on this week’s Canvas page. This file includes live_mass data like
the previous data sets you worked with, but this set has data from different global sites and all of the data are from year 10. 2.
In JMP, choose “File”, and “Open”. Navigate to your saved file and select it.
3.
Your data table should contain 5 columns:
a.
site_name
b.
latitude
c.
year_trt
d.
trt
e.
live_mass
4.
As always, make sure the categorical data (site names and treatments) are “nominal” data types under the “Columns” list, and that all the numeric columns are “continuous”.
5.
To graph live biomass in control plots and fertilized plots by study site, begin by clicking the “Graph” menu in the toolbar at the top of the screen, and open the graph builder.
6.
In the graph builder, drag the site_name variable to the X axis and the live_mass to the Y axis.
10. To show the difference between the control and treatment plots, drag the trt variable to the “Overlay” box.
11. This figure can be displayed as a bar graph or a box and whisker plot. These options are found on the icon bar above the plot. Experiment with the two types of plots and choose the
plot that you feel is the best way to represent these data.
12. Once you have picked your plot type, click “done”. Label your axes and make sure your legend is clear (change it if necessary). You can also change the colors if you need to or prefer to.
●
Observe the variability in the difference between fertilized plots and control plots at different study sites. What patterns do you see?
13. Save your graph by going to File > Export, and export as an image file. You will include this graph as part of your post-lab assignment.
14. Keep the current data table open in JMP, as you’ll continue working with this data set in the last section, below.
Fertilization Effect - Procedure
You will now calculate the fertilization effect by dividing the mean live biomass in the fertilized plots by the live biomass in the control plots for each of the sites.
1.
If you accidentally closed the “Dataset 4 NutNet Global Year 10” data table in JMP when you finished the previous section, open that file in JMP again.
2.
In the menu bar at the top of the screen, click the “Tables” menu and then select “summary”. This will load the summary window. 3.
We want the mean live mass number for each study site, so click on the live_mass variable,
and then select “mean” from the “Statistics” dropdown menu. You should now see Mean(live_mass) in the window next to “Statistics”.
4.
Click on the site_name variable and then click the “Group” button. This will give you the mean live_mass number for each site in a new table. 5.
We also need to include our treatment type, though (control or NPK). Click on the trt variable and then click the “Subgroup” button.
6.
Finally, we want this new data table to include the latitude value for each site. There isn’t a great way to accomplish this using the summary table feature, so we are going to use a work-around instead. Since the latitude value is the same for all data points from the same study site, we can simply include the mean latitude in our data table to allow us to use it for graphing. To do this, click latitude and then under the Statistics dropdown, select “mean”.
7.
Click OK. You should get a new data table that has 6 columns.
8.
Now we need to calculate the fertilization effect for each site. So, create a new column at the end (the far right) of your data table by double-clicking in the empty column. This will create a column called “column 7” that has no data in it. 9.
Double-click on the column 7 header to open the column options window.
10. At the bottom of this window you should see the column properties dropdown. Click on the dropdown to reveal the column properties, and select “formula”.
Your preview ends here
Eager to read complete document? Join bartleby learn and gain access to the full version
- Access to all documents
- Unlimited textbook solutions
- 24/7 expert homework help
11. In the formula window, click the “edit formula” button. This will open the formula editor.
12. In the formula editor, use the panel on the left and the operators at the top to enter a formula that will divide the live mass in the treatment plots by the live mass in the control plots.
13. Click OK. Back in the “column 7” window, click on “Column Name” and name this column “Fertilization Effect”.
14. Click OK, and view your nice new Fertilization Effect column in your table!
15. Finally, you will plot your calculated fertilization effect against latitude, and describe the relationship between them.
a.
With your new data table selected, open the graph builder window (Graph > Graph Builder).
b.
Using the same procedure as you did previously (Primary Production Across Time - graphing procedure), create a scatter plot with a regression line showing the fertilization effect by latitude. Remember to save your plot. You will include this graph as part of your post-lab assignment.
●
What pattern does this figure show? How would you interpret this pattern?
Part 3: Comparing the Impact of Different Fertilizers
You will now use a data set that once again includes multiple NutNet sites, and has biomass data across multiple years. The key difference is that this data set includes not NPK plots, but plots that were each treated with only one of the nutrients (N, P, or K). So, this data set includes four plot types per location: control (no fertilizer), N, P, and K plots. You will use a simple linear regression to determine if productivity in these plots has increased or decreased over time, and compare the rate of increase (biomass increase) in the fertilized plots to that of the control plots.
Graphing live biomass at sites treated with different fertilizers (N, P, or K) - Procedure
1.
Download the necessary data file to your computer: “Dataset 5 NutNet Global Fertilizer Impact”, which is on this week’s Canvas page.
2.
In JMP, choose “File”, and “Open”. Navigate to your saved file and select it.
3.
Your data table should contain 4 columns:
a.
site_name
b.
year_trt
c.
trt
d.
live_mass
4.
Double check that the live_mass data column is formatted as a continuous numeric variable. You can do this by double-clicking on the column header to check the column properties.
5.
Graph live biomass (ANPP) vs. treatment year for all treatment types, following these steps:
a.
Click the “graph” menu on the toolbar at the top of the screen, and open the graph builder.
b.
In the graph builder, drag the year_trt variable to the x-axis. Drag the live_mass variable to the y-axis.
c.
You want separate regression lines for each treatment type (N, P, K, control). To achieve this, drag the trt variable into the “Overlay” box. This should separate your data into different colors based on the treatment type.
d.
You want to look at relationships between the control, N, P, and K treatments at each NutNet site, rather than having them all mixed together in one graph. To do this, you can use the “wrap” function in JMP. Drag the site_name variable into the “Wrap” box that’s at the upper-right corner of the graph.
e.
Next, you need to change the graph type to linear regression by selecting the graph type icon at the top that shows a straight line on a scatter plot. f.
Once you have changed the graph type, you can click “done” in the graph builder window.
g.
Compare and contrast the regression lines from each different plot. Does it appear the nutrient treatments had the same effect at each site? Would you feel confident saying there was a single nutrient (N, P, or K) that limited primary production at all sites?
h.
In this window, you can click on the legend, axes, and other aspects of the graph to customize the visualization. Modify your figure as needed. Once you are happy with your figure, save it by going to File > Export, and export as an image file. You will include this graph as a part of your post-lab assignment.
Analyzing effect of each fertilizer type (N, P, K) at each site
You will now analyze the slopes of the regression lines on the plot you created, in order to determine if there is statistical evidence that the slopes of these lines are significantly different from
zero (zero slope is a flat, horizontal line). You will compare the slope parameters to determine which fertilized plots have the largest slope value compared to the control plots (i.e., a faster rate of
biomass growth over time).
Analyzing the effect of each fertilizer - Procedure
1.
Open the Fit Y by X program under the “Analyze” menu. This will open the Y by X model window.
2.
Add year_trt as the X factor and live_mass as the Y response. This will result in a simple linear model relating these two variables. 3.
We want to see if there is a difference between the control plots and treatments, as well as if those relationships were the same at each site. So, add the trt and site_name variables to
the “By” option. 4.
Hit OK to run the analysis.
5.
You should now see a Bivariate relationship window. This contains 16 scatter plots, one for each treatment at each of the sites. In the upper left corner of the plots, you should see the
small red triangle. Click the triangle for the first plot, and choose “Fit Line” to produce the best fit line.
6.
The best fit line should now show on the bivariate plot, and the linear fit information is below
the plot. Like last time, we are interested in the Analysis of Variance and Parameter Estimates tabs.
7.
The Analysis of Variance tab tells you if the slope of the line is significantly different from zero. Remember that the Prob>F is your p-value for the statistical test. If p<0.05, it means that there is statistical evidence that the slope of the line is different from zero.
8.
Under the parameter estimates, you will see the slope and intercept estimates for the simple linear model describing the best fit line. The slope estimate is found in the year_trt row of the table.
9.
Follow steps 5-8 above for each of the Bivariate plots (i.e., click the red triangle, add the fit line, look at the linear fit information). In the table below, record the slope estimate for each of the 16 regression lines. If the p-value for a line is greater than 0.05 you should record a zero for the slope estimate (if p>0.05 we cannot say that the slope is significantly different from zero). You will turn this table in as part of your post-lab assignment.
10. After you have completed the table, compare the slope estimates for each different treatment type within the same site. What can you conclude about the effect of the different fertilizer treatments on biomass production at each of the 4 sites?
Site Name
Treatment Type (control, N, P, or K)
Slope Estimate
Your preview ends here
Eager to read complete document? Join bartleby learn and gain access to the full version
- Access to all documents
- Unlimited textbook solutions
- 24/7 expert homework help
Post-lab Assignment
Remember to go to Canvas and do the post-lab assignment. You will turn in the 4 figures you made and answer the post-lab questions (which include pasting in the table you made in Part 3).
Related Documents
Recommended textbooks for you
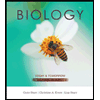
Biology Today and Tomorrow without Physiology (Mi...
Biology
ISBN:9781305117396
Author:Cecie Starr, Christine Evers, Lisa Starr
Publisher:Cengage Learning
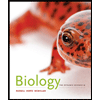
Biology: The Dynamic Science (MindTap Course List)
Biology
ISBN:9781305389892
Author:Peter J. Russell, Paul E. Hertz, Beverly McMillan
Publisher:Cengage Learning
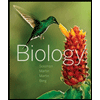
Biology (MindTap Course List)
Biology
ISBN:9781337392938
Author:Eldra Solomon, Charles Martin, Diana W. Martin, Linda R. Berg
Publisher:Cengage Learning
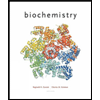
Biochemistry
Biochemistry
ISBN:9781305577206
Author:Reginald H. Garrett, Charles M. Grisham
Publisher:Cengage Learning
Recommended textbooks for you
- Biology Today and Tomorrow without Physiology (Mi...BiologyISBN:9781305117396Author:Cecie Starr, Christine Evers, Lisa StarrPublisher:Cengage LearningBiology: The Dynamic Science (MindTap Course List)BiologyISBN:9781305389892Author:Peter J. Russell, Paul E. Hertz, Beverly McMillanPublisher:Cengage LearningBiology (MindTap Course List)BiologyISBN:9781337392938Author:Eldra Solomon, Charles Martin, Diana W. Martin, Linda R. BergPublisher:Cengage Learning
- BiochemistryBiochemistryISBN:9781305577206Author:Reginald H. Garrett, Charles M. GrishamPublisher:Cengage Learning
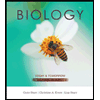
Biology Today and Tomorrow without Physiology (Mi...
Biology
ISBN:9781305117396
Author:Cecie Starr, Christine Evers, Lisa Starr
Publisher:Cengage Learning
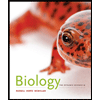
Biology: The Dynamic Science (MindTap Course List)
Biology
ISBN:9781305389892
Author:Peter J. Russell, Paul E. Hertz, Beverly McMillan
Publisher:Cengage Learning
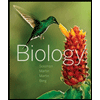
Biology (MindTap Course List)
Biology
ISBN:9781337392938
Author:Eldra Solomon, Charles Martin, Diana W. Martin, Linda R. Berg
Publisher:Cengage Learning
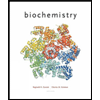
Biochemistry
Biochemistry
ISBN:9781305577206
Author:Reginald H. Garrett, Charles M. Grisham
Publisher:Cengage Learning