Zebrafish & Caffeine S24 version
docx
keyboard_arrow_up
School
University of Minnesota-Twin Cities *
*We aren’t endorsed by this school
Course
1961
Subject
Biology
Date
Feb 20, 2024
Type
docx
Pages
23
Uploaded by ProfessorEnergyKangaroo34
Zebrafish & Caffeine
Caffeine’s effect on zebrafish embryonic heart rate
Connections
●
You will be introduced to a model organism that can be used to study human disease, toxicology, and development. ●
Minnesota is “the land of 10,000 lakes”, and the Mississippi River runs right through our campus. Zebrafish are one organism that can help scientists study the effect of various toxins that end up in aquatic systems due to human activities.
●
You will have the opportunity to put the pipetting and diluting skills you learned last week into practice.
●
This lab activity relates to the Environmental Toxicology research area in our foundations lab courses.
Goals/Skills
After completing this exercise, you will:
●
Have experience using a dissecting microscope.
●
Have been introduced to a model organism (
Danio rerio
, the zebrafish).
●
Have experience describing sources of variability in data, and practiced creating and interpreting error bars on a graph.
●
Have experience using the R statistical software to analyze data and make a graph.
Make your own copy of this document
Remember to make a copy of this document (File>Make a copy), or download a copy (File>Download) to use as your working document.
Introduction
Biomedical research answers questions about human health and leads to new medical treatments and understanding of human diseases. But research using humans is complicated, takes a long time, and (very importantly) is very ethically constrained. Ecosystems are important to study because of their wide ranging impacts and because of the potential for human toxins to affect them. But they are also complex and can be hard to access. So what does a biologist do if they want to answer basic questions about human diseases, complex ecosystems, or potential toxicological effects? Often they use a model organism
, an organism chosen for study because it is easier to study in a biology lab, has lots of established tools and procedures to study it, and has enough similarities to other organisms that a researcher can generalize their findings.
The zebrafish, Danio rerio
, is a small freshwater fish that is native to South Asia, in the same family
as carps and minnows. This species is an important model organism used in biomedical research and environmental toxicity studies. Zebrafish are a useful model organism for studying vertebrate development and physiology for several reasons. They are small in size, develop rapidly, and reproduce quickly. Zebrafish embryos (fertilized, growing eggs) have transparent bodies, which means that development of the tiny fish inside can be easily observed. Because of these advantages that allow for convenient study in the laboratory, zebrafish biology is very well understood. This species also has a relatively high degree of genetic conservation (similarity) with humans, which makes them a good model for studying human genes and diseases (Howe et al. 2013).
Environmental toxicology is the study of the effects of environmental contaminants on organisms or
ecosystems. Caffeine is a commonly-found contaminant in aquatic systems, and its source is domestic wastewater (Ferreira 2005). Caffeine has multiple actions on animal physiology. It has been shown to increase blood pressure, respiration rate, mental alertness, and gastrointestinal motility (Battig 1984). The effects of caffeine on human heart rate are ambiguous, with reports of increased, decreased, and unchanged heart rates (Green & Suls 1996; Bichler et al. 2006; McClaran & Wetter 2007).
In this lab, you will study the effects of caffeine on the heart rates of zebrafish embryos that are 3 days post-fertilization (3 dpf). Cardiovascular development reaches functionality in zebrafish by 2 dpf, and you will see that zebrafish have a 2-chamber cardiac system. The genes involved in zebrafish cardiovascular development are well-known, and it is therefore well-established that zebrafish are a good model for human cardiovascular development (Bakers 2011).
Working with zebrafish
The proper care of animals and concern for animal welfare is fundamental in any laboratory that utilizes animals. Animals employed as laboratory subjects must be treated in a manner that minimizes discomfort, pain, and suffering to the maximum extent that is practical. All research programs involving vertebrates, including zebrafish, have approved protocols by the Institutional Animal Care and Use Committee (IACUC). The zebrafish are to be treated with caution and
respect. Animal care is a priority when working in the laboratory and is highly monitored by the University. Because the zebrafish embryos you are working with in this lab are only 3 dpf, you will not be required to receive any specialized training in the handling and use of the embryos. Those of you who carry on in the zebrafish project later in the semester will receive IACUC training in order to work with embryos older than 3 dpf. Although you do not need special training to perform this experiment, you should pay very close attention to the protocol and your lab instructor to be sure that you are handling and treating the embryos in an appropriate manner.
Day 1
Part 1: Class Discussion and Experimental Design
Lab experiments are done to answer a specific question or test a specific hypothesis. Part of the process of designing an experiment is to ensure that it addresses your question or hypothesis. Experiments should also be designed carefully so you can have confidence in the results. Your lab instructor will lead a class discussion about experimental design for this experiment. Then, as a class, you will come up with an experimental design that you will carry out during the next lab session.
You will be measuring the heart rate of zebrafish embryos treated with or without caffeine. To accomplish this, you will have a dissecting microscope available to you, as well as a timekeeping device (e.g., clock with a second hand, stopwatch, your phone). Your goal is to decide how to collect the most reliable and accurate heart rate data possible. Note: Do not answer these questions before class, we will work on them together in class.
1.
Outline two possible plans for collecting 15 heart rate measurements for both your experimental and comparison conditions. (Possible parameters to consider might include the number of fish to be sampled, the number of times an individual fish will be measured, etc.). Type or write your answers below (or write them on a piece of paper and transfer them here later). Wait for your lab instructor before moving on to question 2.
2.
Using the procedure included in your lab manual (see below) and discussions from class, outline the strategy that you will use for data collection. Type or write your answer below (or
write it on a piece of paper and transfer them later).
Part 2: Preparing for the Experiment
Your preview ends here
Eager to read complete document? Join bartleby learn and gain access to the full version
- Access to all documents
- Unlimited textbook solutions
- 24/7 expert homework help
You will work with a lab partner to complete this experiment during the next lab session. Today, you’ll do some planning and preparation so that you’re ready to jump into the experiment next time.
The first thing you’ll need to do on experiment day is prepare your caffeine solution. We are going to treat the embryos with 8 mM caffeine concentration (mM is millimolar). The data from all groups will be compiled at the end of the lab so that we have a relatively large dataset.
You will be provided with a stock solution of 100 mM caffeine, which you will dilute to arrive at the concentration you need.
One important thing to note about the caffeine concentrations used in this experiment: You will be transferring zebrafish embryos into a petri dish that contains your caffeine solution. The embryos will be in embryo water (water that is slightly saline). When you transfer the embryos, you will also be transferring some of the embryo water (1 ml) to the petri dish containing the caffeine solution. This volume of embryo water must be taken into account when making your dilutions. In other words, if you mix up 10 ml of the desired final caffeine concentration in a petri dish, and then add the embryos along with 1 ml of embryo water, you will have diluted your caffeine concentration beyond the desired concentration. Carry out the dilution calculation below, and make sure you take clear notes and record your numbers so you can refer back to this section when you’re ready to set up your experiment next time.
Dilution calculation
Final volume of solution you will have in your petri dish: 10 ml
Concentration of caffeine stock: 100 mM
Remember: C
1
V
1
= C
2
V
2
where C
1
is the concentration of the stock solution, V
1
is the amount of stock solution you will add to your petri dish, C
2
is the final concentration you want, and V
2
is the final volume of solution in your petri dish.
The caffeine concentration in the stock solution (C
1
): ______________
The caffeine concentration you are testing (C
2
): ______________
The final volume in your petri dish (embryos + caffeine) (V
2
): ______________
Now use algebra to solve for V1 and the above values to calculate the volume of caffeine stock (V
1
) you’ll need
The volume of caffeine stock (V
1
) you’ll need: ______________
Check your dilution math with your lab instructor, or a few other students, before moving to the next step.
Dilution planning
Now, make sure you understand how you will prepare your caffeine dilution. You will add the amount of caffeine stock you calculated above (when you solved for V
1
), then enough embryo water to bring the volume in the dish up to 8.5 ml. Then you will add 0.5 ml of an anesthetic solution, and 5 embryos along with 1 ml of embryo water. This will bring the total volume up to 10 ml and give the appropriate dilution of caffeine. Check your dilution calculations with other students, or check with your lab instructor.
Controls
Controls are an important part of any experimental design. You will need a control that acts as a baseline for zebrafish embryo heart rate. You will compare the heart rates of caffeine-treated embryos to the heart rates of untreated embryos to see if the caffeine causes a difference or not.
Part 3: Introducing the command line, R, & RStudio
So far, you’ve designed your experiment and done some planning. During the next lab, you’ll run your experiment, collect data, and come up with some quantitative ways to describe those data. You’ll also want to know what your results actually say about caffeine’s effect on heart rate. To answer that question, you must analyze your data and communicate those results to others. Figures are essential tools for communicating your results. Today, you’ll prepare for making figures
showing the results (compiled from everyone in the class), so you’ll know how to handle the heart rate data after you run the experiment. R Programming Language & Software
As the amount and complexity of biological data has increased, the tools needed to analyze that data have become more powerful. The most prominent tool used by biologists, statisticians, and data scientists is the R statistical computing system. R has two aspects: a statistical software program and a programming language. The software is what you will open on your computer to analyze your data today. The programming language is the way that users (you!) tell the R software what to do. Instead of clicking, dragging and entering numerical values, like you would in an Excel spreadsheet, in R, you type commands written in the R language that tell the software what to do with your data. It can be difficult to learn to use R initially, but the payoff for learning is enormous. By becoming familiar with R, you will be able to perform modern biological data analysis, work with data sets too large to handle in a visual spreadsheet, and be able to share methods with fellow researchers. To help introduce you to R, in this boot camp section of the course you will perform most data analysis using R. It may be challenging and frustrating, but this powerful software will be an important addition to your collection of biology lab techniques. History of R
R was developed at Bell Laboratories
about thirty years ago, and has been growing ever since. Programmers and bioinformaticians have been creating packages for R, so it has grown considerably in its capabilities over the years. This means using R is somewhat like exploring a giant castle. You will need to spend time walking around in the castle and will likely need to revisit many of the same rooms multiple times before you come to understand where everything is. With some internet searching, you will find many helpful people explaining some interesting applications
of R tools in fields from biology to finance.
Biol 1961 R Guide
We have an R Guide
on Canvas (in the Lab Guides section). This is a guide you can refer to when working with R in future lab exercises. Some of the material in this lab manual is copied from that guide for convenience (so you don’t have to keep flipping between the two documents). Accessing R & RStudio via the RStudio Cloud website
To work with R, we will use RStudio, which is free software that contains R and has some added features that make it much easier and more useful to work with than using R on its own. Technically, RStudio is an Integrated Development Environment (IDE) that works as a single app (interface) for R to create a somewhat personalized console with different panes containing different information about the program you are working on. This may sound confusing, but it will make more sense as you use the software.
Most people who use RStudio install the necessary software on their own computers and work from there. However, to make your first experience with R and RStudio a little simpler, we will use a web-based version called RStudio Cloud (a.k.a. Posit). This will make it easier for you to load the
software, access class data, and get help from instructors.
Orientation to RStudio Cloud
To load RStudio Cloud, use a web browser to access the course workspace. The same link is available on the Week 2 course Canvas page. [This link is currently not working. Please use these alternate instructions to access RStudio Cloud
]
When you open RStudio Cloud, you will need to log in. Click on the "Sign in with Google" link, and use your UMN email & login information to sign into the RStudio Cloud webpage. Alternatively, you
can use your UMN email to set up a new account. Either way of logging in will take you to the workspace for this class, a kind of home page. On the page, you should see a link for an assignment called “Introduction to R”. Click on the start assignment button to open it. This will automatically make a personal copy of the assignment for you. Once open, you will see three panels in the RStudio window (and possibly an RStudio Cloud menu on the left). Take a moment to
orient yourself to what each of these panels do:
Your preview ends here
Eager to read complete document? Join bartleby learn and gain access to the full version
- Access to all documents
- Unlimited textbook solutions
- 24/7 expert homework help
Console
: The left half of the screen is the console. This is where you will input commands.
Environment/variable inspector
: The upper-right panel is the variable inspector. As you work with your data and store them in variables, this panel will show you what variables you have set up and what they contain. For now, you can think of a variable as a jar that contains information. You create the jars, give them a name, and put things into them (numbers, equations, letters, data tables, etc.). Then, instead of typing out the contents of the jar every time you need it, you can just use the name of the jar. Variables make life easier in R! Notice that this panel also has a history tab where you can see all of the recent commands you input into R. File system
: Just like going into your documents, this lists the files and folders you are working with. This is just like the 'finder' on a Mac or the 'windows explorer' on a PC. Notice that there are a
few other tabs, also. You will use the plots tab today when you make graphs from your data. The packages tab shows you all of the function bundles you can import when writing programs. The help panel will be useful to you once you start exploring how various functions are used. The viewer tab is for more advanced interactive graphics, and is outside the scope of this course.
Dropdown menus:
These are the options listed across the top of RStudio (just like most other programs on our computers). These menus include many options you might need. For example, File, Save will save our work.
Working with the Command Line
Most computers and software that we use have a g
raphical u
ser i
nterface (GUI). To interact with the computer, you use a mouse to click on app icons, files, buttons and menus. You can open windows to navigate around the computer and see file icons inside of folders. R, on the other hand,
uses a command line interface. Instead of clicking on things, you give the computer written commands (input), the computer does whatever you just told it to do and it prints something back
out, usually in text format (output). The place where you enter commands is called the console (or sometimes the terminal). In RStudio, the console is by default on the left side of the window. When the console is ready for you to enter a command, you will see the prompt: a > symbol. To use R, you will type a command and then press “Enter”. For example, if you input 2+2 at the console prompt, you will get the output 4. Try that command now in your RStudio Cloud window. In
addition to simple arithmetic, you will also use functions. To use a function, you type its name, followed by parentheses. Most functions will require some input information, which you type between the parentheses. For example, if you type mean(your_data) at the console prompt, R will give you the mean of whatever you recorded in R as your_data. If you type ls() at the console prompt, you will get a list of the variables and objects currently loaded into your R session. One note, upper and lower case letters matter when using R. Make sure you don’t accidentally capitalize letters. Scripts
Instead of typing a series of commands directly into the console prompt, you can also save a list of commands in a text file and run all of the commands at once. This is called a script
. Scripts that contain R commands are called R scripts
. You can create a new R script by choosing “File > New File > R Script” from the dropdown menu in the upper left corner of R Studio. This will open a new document that you can save, write commands into (one per line) and run in the console. When you
save an R script, it will have a “.R” file extension. Scripts are useful because they allow you to save
and share your R work, repeat and reuse analyses, add and build off previous R code, and find and fix errors. In this class, when you analyze data, you will save your R work in scripts and turn in the scripts alongside the actual figures and results that you produce. What can R do?
To give you a first taste of what R can do, you will use a command to make a scatter plot from last year’s zebrafish heart rate data. Once you have an idea of the type of graphs R can make, we will go back and give you some basic R practice and then break down how to make a graph like the one you just saw.
For this part of the lab manual, when you see a line of text in the bold blue Consolas font (
like this
), it is a command that you should run in RStudio. Typing the commands yourself is a much more effective way to learn R, so resist the urge to copy and paste the commands from the lab manual.
Course instructors have pre-loaded a previous year’s dataset into RStudio Cloud. To make a plot using those data, run the following two commands, each on one line. You don’t need to know exactly what the commands are doing at this point, we just want to show you what R can do with zebrafish heart rate data.
library("ggplot2")
Hit “Return” and wait for the > prompt to return to the console, then:
old_caffeine_data <- read.csv("Fall_2017_CaffeineData_ForPractice.csv")
and then
ggplot(old_caffeine_data, aes(x=as.factor(Caffeine_Concentration),y=BPM)) + geom_point(position="jitter") + xlab("Caffeine Concentration (mM)") + ylab("Heart Rate (bpm)")
What did you see? It should have been a publication-quality plot that showed all of the heart rate measurements, grouped by caffeine concentration with clear axis labels. Your goal will be to write a command that makes a similar high-quality plot with your data from this year. And a few other types of plots as well! To get you started, you will go back to the basics in R.
Basic R Programming and Plotting - Code Academy
We will begin by using an interactive course from the Code Academy website to expose you to some of the basic concepts in using R. These short tutorials will also allow you to practice with some of the commands you will need to analyze your data in R. (Note: You DO NOT need to sign up for any paid “Pro” versions of Code Academy or activate a free trial. All the activities we will use are free)
First go to the Code Academy home page
, Choose the Google login option, and use your UMN email account to log in. Then complete the following steps:
●
Once logged in, at the bottom of the next page, choose “Skip Personalization” ●
From your homepage, click “Catalog” at the top of the page and choose “R”
●
Click on the “Learn R” free course on the center of the page
●
Click the large “Start” button ●
Under the “Learn R: Introduction topic, complete the “Introduction to R Syntax” activity (12 steps)
●
When finished, skip any offers of free trials or upgrades
●
Click on the “Syllabus” menu in the upper left and under the “Learn R: Data Frames” topic choose “Introduction to Data Frames”
. You will complete the first three steps in this tutorial:
○
Introduction
○
What is a data frame?
○
CSVs
○
You can stop with this activity after those three steps
●
Lastly, on the Syllabus, under the “Learn R: Fundamentals of Data Visualization with ggplot2” topic you will go the the “Intro to Visualization with R” activity
●
Complete the “Intro to Visualization with R” activity (12 steps)
○
You will learn the basics of the ggplot2 package here and then apply them to zebrafish data you collect in the next class
Now that you know a bit more about how R works and how to make figures in R, you have a good foundation for working with the zebrafish heart rate data that you’ll generate during this week’s experiment. After all the lab sections conduct the experiment on Wednesday and Thursday this week, we’ll have a large class dataset for you to work with (see Part 6, below, if you want a sneak peek of what you’ll do with the class data).
Your preview ends here
Eager to read complete document? Join bartleby learn and gain access to the full version
- Access to all documents
- Unlimited textbook solutions
- 24/7 expert homework help
Day 2
Part 4: The Experiment!
Before you jump into today’s protocol, refer back to your notes from last time about diluting the caffeine and your plan for collecting data. Note that the embryos will be exposed to caffeine for 15 minutes before you begin counting the heartbeats. Ideally, the control embryos and caffeine-
treated embryos will be “treated” for the same amount of time. This may not be technically possible, but keep this goal in mind as you work today. You should keep track of the elapsed time on each petri dish separately.
1.
Obtain 2 petri dishes, and label them on the bottom (not the lids) with your initials/names, and either “control” or the caffeine concentration that you will have in your experimental dish.
2.
To your control dish, add 8.5 ml of embryo water.
3.
To your caffeine dish, first add the amount of 100 mM stock caffeine solution that you calculated above. Then add the appropriate volume of embryo water to bring the volume in the dish up to 8.5 ml.
4.
To each dish, add 0.5 ml of 20X MS-222. This brings the total volume in each dish up to 9 ml. MS-222 is an anesthetic that will help prevent the embryos from spontaneously moving around while you are collecting data. The final concentration in each dish will be 0.017% MS-222.
5.
Use scissors to cut a little bit off the tip of a P1000-size pipette tip. This will ensure that the hole is large enough for the embryos.
Before you add embryos to your petri dishes:
Make a plan with your partner about how you will time your dishes. If you start both at the same “time zero”, one dish will be sitting for
a longer period of time total (while you collect data from the other dish). You may want to stagger the start time of your dishes.
6.
Using a P1000 with a modified pipette tip, transfer embryos, along with 1 ml of embryo water, into each of your dishes. This brings the volume in the dish up to 10 ml. Transfer the number of embryos you need according to your experimental design (5 or 15). If you are going to transfer 15, you’ll probably want to do it in more than one batch (adjust pipettor volume accordingly, so you only end up transferring 1 ml of embryo water in total).
7.
Wait for 15 minutes.
8.
If there are extra embryos available in the lab, you can transfer one to a third petri dish (in enough embryo water to cover it), and practice counting the heart rate while you wait. Make
sure you can locate the heart, and that you understand how to count the beats. You can use the stopwatch function on your phone, or use one of the stopwatches available in the lab.
9.
After 15 minutes has elapsed, begin collecting data.
Note: Letting the petri dish with embryos sit on the dissecting scope with the light turned on for extended periods of time may affect the heart rate. This is unavoidable, but try to minimize this problem by turning the light off whenever you are not counting heartbeats.
10. Count each embryo’s heartbeats for 30 seconds, and do the number of counts per embryo according to your experimental design (measurement strategy A or B). Record your data in the data table below that matches your measurement strategy.
11. When you’re finished collecting data, calculate the beats per minute (bpm) for each trial and
record it in your data table.
12. Add your bpm data to the class data set (your beat per minute data, not your 30-second data) via the data submission form
. Euthanizing zebrafish
In accordance with our IACUC protocol, zebrafish embryos/larvae from 0-3 dpf are euthanized in a bleach solution (minimum 10% bleach). At this early age, zebrafish have not yet developed pain receptors, so this is not considered a painful procedure.
When your experiment is complete, move the embryos into the designated containers of bleach solution in the lab. The petri dishes can go in the trash once they are empty.
Measurement Strategy A: Data Collection Sheet
Add rows as needed.
Treatment Embryo Number Heart rate (30s)) Heart rate (bpm)
1
2
3
4
5
Record any deviations from the procedure or interesting observations you make while collecting your data below.
Measurement Strategy B: Data Collection Sheet
Raw heart rate data. Add rows as needed.
Treatment Embryo number
Measure
1 (30s)
Measure
2 (30s)
Measure 3 (30s)
Average of 3
Measureme
nts
1
2
3
Data converted into Beats Per Minute (BPM). Add rows as needed.
Treatment Embryo number
Heart rate
1 (bpm)
Heart rate
2 (bpm)
Heart rate
3 (bpm)
Average of
3 Heart
rates
1
2
3
Use the space below to record any deviations from the procedure or interesting observations you make while collecting your data.
Part 5: Generating Mathematical Expressions of data
You just ran an experiment to test the impact of caffeine on zebrafish heart rate. You collected heart rate data on fish treated with or without caffeine, and your lab section used two different measurement strategies to collect heart rate data. In this activity, you will explore the extent to which the experimental design, particularly the measurement strategy, impacted the results you observed. Compare and contrast measurements
Think-Pair-Share
Your preview ends here
Eager to read complete document? Join bartleby learn and gain access to the full version
- Access to all documents
- Unlimited textbook solutions
- 24/7 expert homework help
1.
What impact do you think the measurement strategy your group used had on the data you collected? Discuss with a partner who used the opposite measurement strategy, and predict the similarities and differences that might be observed in the data collected.
Strategy A: Sample 15 individual fish Strategy B: Sample 5 fish, measured 3 times each 2.
List the affordances and constraints of each measurement strategy below:
Strategy A
Strategy B
Affordances
●
●
Constraints
●
●
Class Discussion
Your lab instructor will lead a discussion about the impacts of the two measurement strategies on their data. Generating mathematical expressions
3.
Refer back to the data table
where you recorded your heart rate measurements. Write two mathematical expressions that provide summary statistics of the data you collected, and a short sentence describing what they represent. Try to not look these expressions up in class but develop them on your own.
Discuss your mathematical expressions with your partner, come to a consensus on two mathematical expressions, and write them on the large chart paper to share with the rest of the section. Expression 1: Expression 2: Your lab instructor will tell you when to put your large chart paper up on the board. Until that
time, don’t post your paper.
4.
After class discussion, write the two expressions that your section came to a consensus on below: Expression 1: Expression 2:
Using math expressions to practice analyzing data
5.
Download the Part 5 Data Analysis Sheet
file (also available on Canvas). These data are heart rates from comparison (untreated) zebrafish that were collected by BIOL 1961 students from a previous semester.
Use your section-generated mathematical expressions and corresponding Excel
commands to calculate the summary statistics for each measurement strategy, and write
the results of your calculations in the table below:
Strategy A
Strategy B
Mean
Standard
Deviation
6.
Compare your summary statistics between the two measurement strategies. Do the comparison samples appear to differ from one another? What evidence from your summary
statistics above supports your conclusion? Write your answers below.
Part 6: Working with the zebrafish heart rate data set
You’ll need to wait until the course-wide dataset is available in RStudio Cloud to complete this part of the lab. You’ll have time in lab during week 3 to complete this section before the post-lab is due.
For this part of the analysis,
open the “Zebrafish heart rate analysis” assignment in RStudio Cloud
. With the assignment open, rather than entering code directly into the console window, open
a new script. You will write your code in the script and then run lines of code from the script window/pane in the console. This allows you to better keep track of your commands and to save your work (by saving the script file). Working in a script window also allows for easier editing of lines of code if you get error messages or unexpected results from a line of code. Only keep lines of code that you know work. Open a script window by either clicking on the new document icon at the upper left corner of the RStudio Cloud window (white page with a green plus sign) and selecting “R Script”, or by going to File>New File>R Script. A new pane will appear above your console. You can have multiple scripts
open at one time (they will be in tabs in the scripts pane).
Once you write some code in your script, you should save it. You can do this by selecting the File>Save menu or by typing command/ctrl-S. Make sure that you include your full name in the script file name. At the end of this lab, you will download your script and turn it in as part of your post-lab assignment. You can run lines of code from your script three different ways: 1.
Highlight, copy and paste the code into the console. Then hit Enter
2.
Put your cursor on the line of code you want to run and type Command/ctrl-Enter
3.
Put your cursor on the line of code you want to run and press the Run button in the upper right corner of the Script pane.
You can run multiple lines of code at one time by highlighting the lines you want and using one of the above methods. Make a new script now and record the code you use during this analysis in this script. You will turn it in for credit at the end of this lab. As you may know, we have compiled each lab section’s data from the zebrafish experiment. The data file has been cleaned up slightly (e.g., removing non-numerical data, miscellaneous
Your preview ends here
Eager to read complete document? Join bartleby learn and gain access to the full version
- Access to all documents
- Unlimited textbook solutions
- 24/7 expert homework help
punctuation marks, and anything else people used to signify missing data points). Empty rows were also removed. All of those things could make working with the data in R somewhat more complicated, so those non-numeric entries were removed. The data file is a .csv file and is loaded into an RStudio Cloud assignment called “Zebrafish heart rate analysis”. Next, you need to get the data from the file into R. We’ll label the data frame “caffeine” in R. For this part of the lab manual, when you see a line of text in the red blue Consolas font
(
like this
)
, it is a command that should be added to your script and run from there.
caffeine <- read.csv('heart_rate_data.csv')
The above line tells RStudio to read the data file, which is in “c
omma s
eparated v
alue” or csv format, and and save it to a data frame we’re going to call “caffeine.” A handy command to know in R is the
ls()
command, which gives you a list. If you use this command on a directory you are working within (like your Downloads folder), R will return a list of the files in that directory. Here, you can use the “ls” command to see a list of the variables in the caffeine data set (the column headers). Try this command:
ls(caffeine)
Note that the variables are listed in alphabetical order, not the order they occur in the data set. Do you doubt that the object you’ve just created is a data frame? Try the class() function on “caffeine” to check!
Now change the column names to something more useful
colnames(caffeine) <- c("Trial_Number","Caffeine_Concentration","BPM")
Typing in the name of the data frame, caffeine
, will allow you to see the entire data table in R, including your new column names. This is usually a bit cumbersome, as you may work with some very large data sets in the future. The head() function is usually more convenient, as it shows only the first several rows of data. Try using head() to view the first few rows of “
caffeine
.”
head(caffeine)
Make a note of what columns you see, and what each column contains. To see the end of the data
frame, you can use the tail() function. Remember that you can also use ls() to see what the column
headers are. Graphing the Data - Scatterplot
To make a plot of the data, you’ll first need to tell R that you intend to use ggplot2. You therefore have to call the library function.
library(ggplot2)
library(Hmisc)
Now you’re ready to make your first plot! We will start by making a scatter plot in which each point/dot on the plot represents one data point. The caffeine concentration will be on the x-axis, and the heart rate (bpm) will be on the y-axis.
Recall the intro to ggplot2 and the sample plot you made
during the last lab, and see if you can fill in the blanks to construct a command for your plot:
function name: _____________________________
data = ____________________________________
aes = _____________________________________
x= _______________________________________
y= _______________________________________
geom type: ________________________________
Full command: _________________________________________________________________
______________________________________________________________________________
If you need help constructing your command, refer back to the ggplot2 tutorial, type ?ggplot2 into the console to access the support materials built into R or visit http://ggplot2.tidyverse.org/reference/
. Once you have made your best guess, check it against the full answer on the next page.
The full command to make a scatter plot with your data in ggplot2 is: ggplot(data=caffeine, aes(x=Caffeine_Concentration, y=BPM)) + geom_point()
In the lower-right pane, you should see your scatterplot. If you don’t, make sure the Plots
tab is selected. You should see a plot with data points and x axis labels at 0mM, 2mM, 4mM and 8mM. But why is there a place on the plot for 2, 4, and 6mM when you didn’t collect data at those concentrations? The answer has to do with what type
of data R thinks you are using. Right now, it thinks the data is a continuous numerical variable, so it made a scatter plot. All the data points have an x-axis value of 0 or 8, so that’s where R plotted them. To make the plot more visually consistent with your data, we can turn the x-axis into discrete categories. To do that we use the as.factor() command on our Caffeine_Concentration data:
caffeine$Caffeine_Concentration <- as.factor(caffeine$Caffeine_Concentration)
Now try running your ggplot2 command again and see what your plot looks like. Is there still a 2, 4,
and 6 mM category?
ggplot(data=caffeine, aes(x=Caffeine_Concentration, y=BPM)) + geom_point()
Because the independent variable only has 2 levels (0mM and 8mM caffeine concentrations), it is a bit difficult to get a sense of the data with this graph. There are many data points that are plotted on top of each other in this graph (values that are identical or nearly identical). We can use a technique called “jitter” to slightly scatter the data horizontally, which will allow us to see more of the points and get a better sense of the spread of the data. Use the same command as above, but now add position = “jitter” inside the geom_point() function at the end, as you see below. Remember that you can use the up arrow to scroll through previous lines of code you’ve entered (to save you some typing).
ggplot(data=caffeine, aes(x=Caffeine_Concentration, y=BPM)) + geom_point(position = "jitter")
Now we have a scatterplot with jittered points, so we can see the data more clearly! Jittering can be very useful when you are visualizing your data and looking for patterns. However, jittering can sometimes lead to incorrect interpretation of the data. Therefore, jittering is generally something we
do when we are exploring our data, but not something we often do on figures that will be published or shared broadly. If a plot requires jittering to be meaningful, we often look for other ways to represent our data, such as a boxplot. If you do publish or present a plot with jittered points, you need to make that clear to your audience. Do you notice all the data points at zero in the y-axis? Those represent fish with no heart rate, meaning dead fish. We want to exclude dead fish from our plot. Use the command below to remove fish with BPM of zero from the dataset:
caffeine <- subset(caffeine, caffeine$BPM > 0)
Your preview ends here
Eager to read complete document? Join bartleby learn and gain access to the full version
- Access to all documents
- Unlimited textbook solutions
- 24/7 expert homework help
Turn your attention to the axis labels on the graph. R has labeled the axes, but the labels are not exactly what we want. They are not appropriately descriptive axis labels. To re-make the plot with customized axis labels, use the code below. Note that it is mostly the same code you used before. We’re simply adding the parameters of what we want the x- and y-axis labels to be (xlab and ylab, respectively). You can therefore use the up arrow to get the previous code for the graph, and add the axis label components to the middle.
ggplot(data=caffeine, aes(x=Caffeine_Concentration, y=BPM)) + geom_point(position = "jitter") + xlab("Caffeine Concentration (mM)") + ylab("Heart Rate (bpm)")
Now your plot has nice axis labels! Let’s save your plot using the ggsave() function. First, assign the ggplot command you just used to a variable. This way, instead of typing the entire command over and over, you can just type in the variable name. You can name the variable anything; my_first_plot works great.
my_first_scatter_plot <- ggplot(data=caffeine, aes(x=Caffeine_Concentration, y=BPM)) + geom_point(position = "jitter") + xlab("Caffeine Concentration (mM)") + ylab("Heart Rate (bpm)")
Next, use the ggsave command to save your plot. Make sure to replace YOURNAME with your actual name. The file will save in the current working directory, which we set to be the Desktop earlier. ggsave(filename = "
YOURNAME
_scatterplot.png", my_first_scatter_plot)
Now go to your Files tab, find the file and click to open or download it to see your plot! Save the plot you just made because you will submit it as part of your post lab assignment.
Alternatively, you can export your plot from the lower-right pane in RStudio. Go to the Plots tab, and at the top of the pane choose Export>Save as image.
Graphing the Data – Bar Plot
We can also use ggplot2 to make a bar plot showing the mean heart rate for each caffeine concentration. If you haven’t already loaded the ggplot software into R, make sure to do that now with library(ggplot2)
. Let's look at a bar plot of the average, or mean, heart rate of the zebrafish embryos treated with each caffeine concentration.
ggplot(caffeine, aes(x=Caffeine_Concentration,y=BPM, fill = Caffeine_Concentration))+ geom_bar(position = "dodge", stat = "summary", fun = "mean")
In the above command, notice the data frame used (caffeine), the variable assigned to the X and Y
axes (Caffeine_Concentration and BPM, from the caffeine data frame), and the type of plot we added (geom_bar is a bar graph). This is a good start, but similar to the scatter plot, we can customize the axis labels to make them more informative:
ggplot(caffeine, aes(x=Caffeine_Concentration,y=BPM, fill = Caffeine_Concentration))+
geom_bar(position = "dodge", stat = "summary", fun = "mean")+
xlab("Caffeine Concentration (mM)") + ylab("Heart Rate (bpm)")
While the mean is useful, it certainly doesn't tell the entire story of your data set. It is also important
to understand how much variation there is in your data (in this case, how much variation there is in the heart rate values in each group). To assess how much variation there is around the mean, we calculate the variance
. The variance is calculated by subtracting the mean from each data point, squaring each of those values, summing them up, and dividing by the sum by n
−1 (where n
is the
sample size, or number of data points in that group). The differences between each data point and the mean are squared so that they are all positive numbers (you will have some data points that are lower than the mean, and some that are higher, so without squaring the values you would have
both negative and positive numbers).
Where x
i
is each data point, is the mean, and n
is the sample size.
So, the variance tells us how much variation there is in the data set. However, one problem with the variance is that the units of the variance are not the same as the units of the data set. For your heart rate data, the unit of measurement is bpm (beats per minute). The unit of the variance is bpm
2
, though. To solve this, we often report the standard deviation
instead of the variance. The standard deviation is simply the square root of the variance. By taking the square root of the variance, we are back to the same unit of measurement as our data set.
Luckily for us, there is a simple way to have R calculate the standard deviation for your data and add it to your bar plot:
ggplot(caffeine, aes(x=Caffeine_Concentration,y=BPM, fill = Caffeine_Concentration))+
geom_bar(position = "dodge", stat = "summary", fun = "mean")+
xlab("Caffeine Concentration (mM)") + ylab("Heart Rate (bpm)") +
stat_summary(fun.data = mean_sdl, fun.args = list(mult = 1), show.legend = FALSE)
While the standard deviation can tell us about the variance in our data set, the standard error of the
mean (often known simply as “standard error”), tells us how confident we are that the mean we measured in our sample of zebrafish actually represents the mean for all zebrafish. This is important because the very reason we do experiments is to generalize a conclusion from a small sample to a general population. So let’s make our bar plot with standard errors:
ggplot(caffeine, aes(x=Caffeine_Concentration,y=BPM, fill = Caffeine_Concentration))+
geom_bar(position = "dodge", stat = "summary", fun = "mean")+
xlab("Caffeine Concentration (mM)") + ylab("Heart Rate (bpm)") +
stat_summary(fun.data = mean_se, show.legend = FALSE) Note: Sometimes the standard error is so small it is hard to see on the bar.
You can now see the mean value for each group. The mean heart rate is an important thing to know, of course, but it may not always be the best piece of information with respect to the effect of caffeine on zebrafish heart rate. What happens if there are outliers (heart rates that are very high or very low than most of the others)? These outliers could skew the average much smaller or larger
than that of a typical embryo in each group. For an extreme example of how an outlier can affect the mean, think of a typical college classroom with 18 students in it. We would guess that the average net worth of a student in that class is probably pretty close to $0 because most students have not begun to amass stocks, bonds, homes, furniture, etc. But what happens if Bill Gates suddenly enters that classroom? Then there are 18 students with no money and one man with billions. If we add up the net worth of all the people in the classroom, and divide by 19 to get the average net worth, we would find that the average person in the classroom is a multimillionaire. But the typical student in the classroom has much less money than that.
So, obviously there needs to be other ways to find out what a "typical" heart rate value might be. One common method is to rank
the data (order them from smallest to largest), and then take the measurement that falls in the middle. This value is the median
. So, for our classroom example above, we would find the net worth of the 10
th
poorest person. We would probably see that the median net worth of someone in our classroom is pretty close to $0, even with Bill Gates in the mix. In this situation, the median is a much better measure of the typical net worth than the mean.
On the other hand, when the data doesn't have any outliers in it, the mean is a good measure of what is "typical" in that group, and the mean and median values will usually be pretty similar. When
there are outliers, though, the mean is influenced by them, and it isn't a very good measure of what's "typical". In other words, the median is more robust
to outliers than the mean. Let’s make a barplot using the median value:
ggplot(caffeine, aes(x=Caffeine_Concentration,y=BPM, fill = Caffeine_Concentration))+
Your preview ends here
Eager to read complete document? Join bartleby learn and gain access to the full version
- Access to all documents
- Unlimited textbook solutions
- 24/7 expert homework help
geom_bar(position = "dodge", stat = "summary", fun = "median")
What changed from the command we used to make a barplot with the mean? (Type your answer below):
Again, we can improve our plot by adding more specific axis labels:
ggplot(caffeine, aes(x=Caffeine_Concentration,y=BPM, fill = Caffeine_Concentration)) +
geom_bar(position = "dodge", stat = "summary", fun = "median") +
xlab("Caffeine Concentration (mM)") + ylab("Median `Heart Rate (bpm)")
Save your plots and script with meaningful names (hint: include the word “median” or “mean” in the
file name) and download them to your computer. You will upload your finished figures and your final R script to Canvas as part of your post-lab assignment. In a future lab, we will discuss figure titles and figure captions/legends, and work on activities to help you understand how to write captions for your figures. For now, you do not need to add captions to your figures.
*Portions used or adapted from Biol 3004 Learn Metagenomics! website, metagenomics3004.appspot.com
References
Bakkers J. 2011. Zebrafish as a model to study cardiac development and human cardiac disease. Cardiovascular research 91(2): 279-288.
Battig K., Buzzi R., Martin J.R., Feierabend J.M. 1984. The effects of caffeine on physiological functions and mental performance. Experientia 40(11): 1218-1223.
Bichler A., Swenson A., Harris M.A. 2006. A combination of caffeine and taurine has no effect on short term memory but iduces changes in heart rate and mean arterial blood pressure. Amino Acids 31(4): 471-476.
Department of Biology Teaching + Learning, University of Minnesota. 2015. Biol 3004: Learn Metagenomics! Accessed September 5, 2015. <metagenomics3004.appspot.com>
Ferreira A. P. 2005. Caffeine as an environmental indicator for assessing urban aquatic ecosystems. Cad. Saúde Pública 21(6): 1884-1892.
Your preview ends here
Eager to read complete document? Join bartleby learn and gain access to the full version
- Access to all documents
- Unlimited textbook solutions
- 24/7 expert homework help
Green P.J., Suls J. 1996. The effects of caffeine on ambulatory blood pressure, heart rate, and mood in coffee drinkers. J. Behav. Med. 19: 111-128.
Howe K., Clark M.D., Torroja C.F. et al. 2013. The zebrafish reference genome sequence and its relationship to the human genome. Nature 496 (7446): 498-503.
McClaran S.R., Wetter T.J. 2007. Low doses of caffeine reduce heart rate during submaximal cycle ergometry. J. Int. Soc. Sports Nutr 4:11.
Rana N., Moond M., Marthi A, Bapatla S., Sarvepalli T., Chatti K., Challa A.K. 2010. Caffeine-
induced effects on heart rate in zebrafish embryos and possible mechanisms of action: an effective system for experiments in chemical biology. Zebrafish 7(1): 69-81.
Post-lab Assignment
Remember to go to Canvas and do the post-lab assignment. You will turn in the plots you made, your R script, and your answers to the post-lab questions.
Your preview ends here
Eager to read complete document? Join bartleby learn and gain access to the full version
- Access to all documents
- Unlimited textbook solutions
- 24/7 expert homework help
Related Documents
Recommended textbooks for you
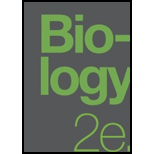
Biology 2e
Biology
ISBN:9781947172517
Author:Matthew Douglas, Jung Choi, Mary Ann Clark
Publisher:OpenStax
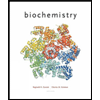
Biochemistry
Biochemistry
ISBN:9781305577206
Author:Reginald H. Garrett, Charles M. Grisham
Publisher:Cengage Learning
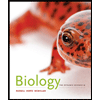
Biology: The Dynamic Science (MindTap Course List)
Biology
ISBN:9781305389892
Author:Peter J. Russell, Paul E. Hertz, Beverly McMillan
Publisher:Cengage Learning
Recommended textbooks for you
- Biology 2eBiologyISBN:9781947172517Author:Matthew Douglas, Jung Choi, Mary Ann ClarkPublisher:OpenStaxBiochemistryBiochemistryISBN:9781305577206Author:Reginald H. Garrett, Charles M. GrishamPublisher:Cengage Learning
- Biology: The Dynamic Science (MindTap Course List)BiologyISBN:9781305389892Author:Peter J. Russell, Paul E. Hertz, Beverly McMillanPublisher:Cengage Learning
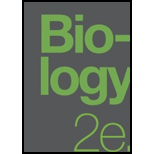
Biology 2e
Biology
ISBN:9781947172517
Author:Matthew Douglas, Jung Choi, Mary Ann Clark
Publisher:OpenStax
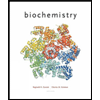
Biochemistry
Biochemistry
ISBN:9781305577206
Author:Reginald H. Garrett, Charles M. Grisham
Publisher:Cengage Learning
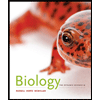
Biology: The Dynamic Science (MindTap Course List)
Biology
ISBN:9781305389892
Author:Peter J. Russell, Paul E. Hertz, Beverly McMillan
Publisher:Cengage Learning