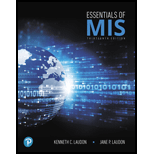
Essentials of MIS (13th Edition)
13th Edition
ISBN: 9780134802756
Author: Kenneth C. Laudon, Jane Laudon
Publisher: PEARSON
expand_more
expand_more
format_list_bulleted
Concept explainers
Expert Solution & Answer
Chapter 6, Problem 1IQ
Explanation of Solution
Answer to interviewer question:
The job seeker is well known about substation equipment. The substation equipment is used in the fields of electrical generation, transmission, and distribution system. This equipment has assembled with electrical components like transformers, auxiliaries, switchgear, etc. This equipment is used many things they are:
- Protecting the system of transmission
- Controlling the exchange of energy
- Ensuring the steady state and transient stability.
SAP for utilities:
“Yes”, the job seeker was worked with SAP for utilities. SAP stands for Systems, Applications and Products in data processing. The SAP utilities is used to manage and bill residential, commercial, and prospective customers. The job seeker also managed the data which is fixed for long period of time in SAP.
Want to see more full solutions like this?
Subscribe now to access step-by-step solutions to millions of textbook problems written by subject matter experts!
Students have asked these similar questions
What are the two overarching methods the author considers for resolving energy problems? Please provide some examples.
What is SDLC and what is it used for?
Outline the concept of a hot zone, its functioning, and any possible downsides.
Chapter 6 Solutions
Essentials of MIS (13th Edition)
Ch. 6.3 - Identify the problem in this case study. To what...Ch. 6.3 - Prob. 2CQ1Ch. 6.3 - Prob. 4CQ1Ch. 6.5 - Prob. 1CQ2Ch. 6.5 - What people, organization, and technology issues...Ch. 6.5 - Prob. 3CQ2Ch. 6.5 - Prob. 4CQ2Ch. 6 - Prob. 1IQCh. 6 - Prob. 2IQCh. 6 - Prob. 3IQ
Ch. 6 - Prob. 4IQCh. 6 - Prob. 1RQCh. 6 - Prob. 2RQCh. 6 - Prob. 3RQCh. 6 - Prob. 4RQCh. 6 - Prob. 5DQCh. 6 - Prob. 6DQCh. 6 - Prob. 7DQCh. 6 - Prob. 8HMPCh. 6 - Prob. 9HMPCh. 6 - Prob. 11HMPCh. 6 - Prob. 13CSQCh. 6 - Prob. 14CSQCh. 6 - Prob. 15CSQCh. 6 - Prob. 16CSQCh. 6 - Prob. 17MLMCh. 6 - Prob. 18MLM
Knowledge Booster
Learn more about
Need a deep-dive on the concept behind this application? Look no further. Learn more about this topic, computer-science and related others by exploring similar questions and additional content below.Similar questions
- Many of our computer related products are produced in other countries, where working conditions are not the same as in the United States. What do you think about your technology products being produced in other countries? Would you be willing to pay more for a computer related product produced entirely in the United States? Is there an ethical issue here? Big picture...would your purchasing decision be impacted if you had knowledge about the working conditions of those who make the product? Why or Why not?arrow_forwardExplain the term "hot zone," explain how it operates, and discuss any potential limitations that it may have.arrow_forwardlegal aspects of cybersecurity Taissa, Sophie, Natalie, Misty, and Jackie all share a house in downtown Dayton. Duringthe great blizzard of 2023, all five co-habitants were snowed in and quickly ran out offood. Jackie tried to leave the house to get food, but quickly fell in the snow. Jackie’sroommates then murdered Jackie, and ate some of her remains to survive. Now the snowhas melted, and the girls are worried about the police finding out what they did. Taissa wants to keep some evidence to blackmail her friends just in case things turnsour in the future. Whenever the girls discuss Jackie’s murder, Taissa surreptitiouslyrecords the discussions on her iPhone without anyone else knowing. Which of thefollowing best describes Taissa’s actions?A. Taissa committed an unconstitutional act.B. Taissa violated the Wiretap ActC. Taissa violated the Electronic Communications Privacy ActD. Taissa’s actions recording her friends are legal in most states.arrow_forward
- legal aspects of cybersecurity Taissa, Sophie, Natalie, Misty, and Jackie all share a house in downtown Dayton. Duringthe great blizzard of 2023, all five co-habitants were snowed in and quickly ran out offood. Jackie tried to leave the house to get food, but quickly fell in the snow. Jackie’sroommates then murdered Jackie, and ate some of her remains to survive. Now the snowhas melted, and the girls are worried about the police finding out what they did. Natalie has had enough. She takes Jackie’s remains, throws them in a black, thick,hefty bag, and tosses them into the Rumpke dumpster she wheels from the side of thehouse, past the fence surrounding her house, and onto the curb in front of her house thenight before trash collection. Police have been waiting in a car on the street andimmediately search the hefty bag as soon as Natalie goes inside the house. Police findthe remains, obtain a warrant for the home, and arrest the girls. Did the police commitany Fourth Amendment…arrow_forwardA prescription drug firm has asked for your assistance in repairing a network issue that affects a large number of PC Appliances used to handle the company's supply chain. You have been given very little information, with the exception of the knowledge that the Computers do not routinely connect with one another. Using whichever method of problem solving you are most comfortable with, explain how you would go about figuring out what the problem is, how you would evaluate the scenario, and how you would come up with a solution.arrow_forward
arrow_back_ios
arrow_forward_ios
Recommended textbooks for you
- Principles of Information Security (MindTap Cours...Computer ScienceISBN:9781337102063Author:Michael E. Whitman, Herbert J. MattordPublisher:Cengage LearningPrinciples of Information Systems (MindTap Course...Computer ScienceISBN:9781285867168Author:Ralph Stair, George ReynoldsPublisher:Cengage Learning
- Enhanced Discovering Computers 2017 (Shelly Cashm...Computer ScienceISBN:9781305657458Author:Misty E. Vermaat, Susan L. Sebok, Steven M. Freund, Mark Frydenberg, Jennifer T. CampbellPublisher:Cengage LearningFundamentals of Information SystemsComputer ScienceISBN:9781305082168Author:Ralph Stair, George ReynoldsPublisher:Cengage LearningSystems ArchitectureComputer ScienceISBN:9781305080195Author:Stephen D. BurdPublisher:Cengage Learning
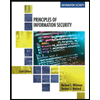
Principles of Information Security (MindTap Cours...
Computer Science
ISBN:9781337102063
Author:Michael E. Whitman, Herbert J. Mattord
Publisher:Cengage Learning
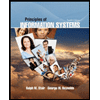
Principles of Information Systems (MindTap Course...
Computer Science
ISBN:9781285867168
Author:Ralph Stair, George Reynolds
Publisher:Cengage Learning
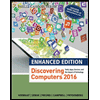
Enhanced Discovering Computers 2017 (Shelly Cashm...
Computer Science
ISBN:9781305657458
Author:Misty E. Vermaat, Susan L. Sebok, Steven M. Freund, Mark Frydenberg, Jennifer T. Campbell
Publisher:Cengage Learning
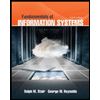
Fundamentals of Information Systems
Computer Science
ISBN:9781305082168
Author:Ralph Stair, George Reynolds
Publisher:Cengage Learning
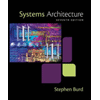
Systems Architecture
Computer Science
ISBN:9781305080195
Author:Stephen D. Burd
Publisher:Cengage Learning