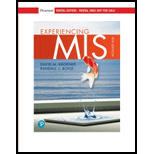
Experiencing MIS
8th Edition
ISBN: 9780134792736
Author: KROENKE
Publisher: PEARSON
expand_more
expand_more
format_list_bulleted
Expert Solution & Answer
Chapter 4.2, Problem 6SW
Explanation of Solution
Security or privacy concerns associated with smart devices
- The Internet of Things (IoT) is rapidly applying connectivity to everyday appliances and home features.
- Many people use personal digital assistants for analysing the past commands for trying to anticipate all the needs.
- These can be linked to accounts for purchasing goods and services and making changes in homes such as turning off alarms, turning on the lights, or adjusting the temperature...
Expert Solution & Answer

Want to see the full answer?
Check out a sample textbook solution
Students have asked these similar questions
Capsim Team PowerPoint Presentations - Slide Title: Key LearningsWhat were the key learnings that you discovered as a team through your Capsim simulation?
Write the SQL code that permits to implement the tables: Student and Transcript. NB: Add the constraints on the attributes – keys and other.
Draw an ERD that will involve the entity types: Professor, Student, Department and Course. Be sure to add relationship types, key attributes, attributes and multiplicity on the ERD.
Chapter 4 Solutions
Experiencing MIS
Ch. 4.2 - Prob. 1SWCh. 4.2 - Prob. 2SWCh. 4.2 - Prob. 3SWCh. 4.2 - Prob. 4SWCh. 4.2 - Prob. 5SWCh. 4.2 - Prob. 6SWCh. 4 - Prob. 1EGDQCh. 4 - Prob. 2EGDQCh. 4 - Prob. 3EGDQCh. 4 - Prob. 4EGDQ
Ch. 4 - Prob. 1ARQCh. 4 - Prob. 2ARQCh. 4 - Prob. 3ARQCh. 4 - Prob. 4ARQCh. 4 - Prob. 1UYKCh. 4 - Prob. 2UYKCh. 4 - Prob. 3UYKCh. 4 - Prob. 4CECh. 4 - Prob. 5CECh. 4 - Prob. 6CECh. 4 - Prob. 7CECh. 4 - Prob. 8CECh. 4 - Prob. 9CSCh. 4 - Prob. 10CSCh. 4 - Prob. 11CSCh. 4 - Prob. 12CSCh. 4 - Prob. 13CSCh. 4 - Prob. 14MLMCh. 4 - Prob. 15MLM
Knowledge Booster
Similar questions
- Draw an ERD that represents a book in a library system. Be sure to add relationship types, key attributes, attributes and multiplicity on the ERD.arrow_forward2:21 m Ο 21% AlmaNet WE ARE HIRING Experienced Freshers Salesforce Platform Developer APPLY NOW SEND YOUR CV: Email: hr.almanet@gmail.com Contact: +91 6264643660 Visit: www.almanet.in Locations: India, USA, UK, Vietnam (Remote & Hybrid Options Available)arrow_forwardProvide a detailed explanation of the architecture on the diagramarrow_forward
- hello please explain the architecture in the diagram below. thanks youarrow_forwardComplete the JavaScript function addPixels () to calculate the sum of pixelAmount and the given element's cssProperty value, and return the new "px" value. Ex: If helloElem's width is 150px, then calling addPixels (hello Elem, "width", 50) should return 150px + 50px = "200px". SHOW EXPECTED HTML JavaScript 1 function addPixels (element, cssProperty, pixelAmount) { 2 3 /* Your solution goes here *1 4 } 5 6 const helloElem = document.querySelector("# helloMessage"); 7 const newVal = addPixels (helloElem, "width", 50); 8 helloElem.style.setProperty("width", newVal); [arrow_forwardSolve in MATLABarrow_forward
- Hello please look at the attached picture. I need an detailed explanation of the architecturearrow_forwardInformation Security Risk and Vulnerability Assessment 1- Which TCP/IP protocol is used to convert the IP address to the Mac address? Explain 2-What popular switch feature allows you to create communication boundaries between systems connected to the switch3- what types of vulnerability directly related to the programmer of the software?4- Who ensures the entity implements appropriate security controls to protect an asset? Please do not use AI and add refrencearrow_forwardFind the voltage V0 across the 4K resistor using the mesh method or nodal analysis. Note: I have already simulated it and the value it should give is -1.714Varrow_forward
arrow_back_ios
SEE MORE QUESTIONS
arrow_forward_ios
Recommended textbooks for you
- Principles of Information Systems (MindTap Course...Computer ScienceISBN:9781285867168Author:Ralph Stair, George ReynoldsPublisher:Cengage LearningEnhanced Discovering Computers 2017 (Shelly Cashm...Computer ScienceISBN:9781305657458Author:Misty E. Vermaat, Susan L. Sebok, Steven M. Freund, Mark Frydenberg, Jennifer T. CampbellPublisher:Cengage Learning
- Fundamentals of Information SystemsComputer ScienceISBN:9781337097536Author:Ralph Stair, George ReynoldsPublisher:Cengage LearningFundamentals of Information SystemsComputer ScienceISBN:9781305082168Author:Ralph Stair, George ReynoldsPublisher:Cengage LearningPrinciples of Information Security (MindTap Cours...Computer ScienceISBN:9781337102063Author:Michael E. Whitman, Herbert J. MattordPublisher:Cengage Learning
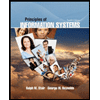
Principles of Information Systems (MindTap Course...
Computer Science
ISBN:9781285867168
Author:Ralph Stair, George Reynolds
Publisher:Cengage Learning
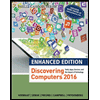
Enhanced Discovering Computers 2017 (Shelly Cashm...
Computer Science
ISBN:9781305657458
Author:Misty E. Vermaat, Susan L. Sebok, Steven M. Freund, Mark Frydenberg, Jennifer T. Campbell
Publisher:Cengage Learning
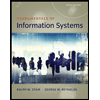
Fundamentals of Information Systems
Computer Science
ISBN:9781337097536
Author:Ralph Stair, George Reynolds
Publisher:Cengage Learning
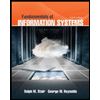
Fundamentals of Information Systems
Computer Science
ISBN:9781305082168
Author:Ralph Stair, George Reynolds
Publisher:Cengage Learning
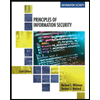
Principles of Information Security (MindTap Cours...
Computer Science
ISBN:9781337102063
Author:Michael E. Whitman, Herbert J. Mattord
Publisher:Cengage Learning