The following estimated regression equation was developed for a model involving two independent variables. ŷ = 40.7 + 8.63x, + 2.71x, After x, was dropped from the model, the least squares method was used to obtain an estimated regression equation involving only x, as an independent variable. ý = 42.0 + 9.01x, (a) Give an interpretation of the coefficient of x, in both models. In the two independent variable case, the coefficient of x, represents the expected change in y corresponding to a -Select--- v unit ---Select--- v in x, when x, is held constant. In the single independent variable case, the coefficient of x, represents the expected change in y corresponding to a --Select--- v unit increase in x,. (b) Could multicollinearity explain why the coefficient of x, differs in the two models? If so, how? |---Select--- v. If x, and x, are correlated, one would expect a change in x, to be --Select--- v a change in X,.
Correlation
Correlation defines a relationship between two independent variables. It tells the degree to which variables move in relation to each other. When two sets of data are related to each other, there is a correlation between them.
Linear Correlation
A correlation is used to determine the relationships between numerical and categorical variables. In other words, it is an indicator of how things are connected to one another. The correlation analysis is the study of how variables are related.
Regression Analysis
Regression analysis is a statistical method in which it estimates the relationship between a dependent variable and one or more independent variable. In simple terms dependent variable is called as outcome variable and independent variable is called as predictors. Regression analysis is one of the methods to find the trends in data. The independent variable used in Regression analysis is named Predictor variable. It offers data of an associated dependent variable regarding a particular outcome.
![**Regression Analysis: Understanding Coefficients**
The following estimated regression equation was developed for a model involving two independent variables:
\[
\hat{y} = 40.7 + 8.63x_1 + 2.71x_2
\]
After \(x_2\) was dropped from the model, the least squares method was used to obtain an estimated regression equation involving only \(x_1\) as an independent variable:
\[
\hat{y} = 42.0 + 9.01x_1
\]
**(a) Interpretation of the Coefficient of \(x_1\) in Both Models**
In the two independent variable model, the coefficient of \(x_1\) represents the expected change in \(y\) corresponding to a change in \(x_1\) when \(x_2\) is held constant. In the single independent variable model, the coefficient of \(x_1\) represents the expected change in \(y\) corresponding to a change in \(x_1\).
**(b) Impact of Multicollinearity on the Coefficient of \(x_1\)**
Could multicollinearity explain why the coefficient of \(x_1\) differs in the two models? If so, how?
When \(x_1\) and \(x_2\) are correlated, one would expect a change in \(x_1\) to be linked with a change in \(x_2\).](/v2/_next/image?url=https%3A%2F%2Fcontent.bartleby.com%2Fqna-images%2Fquestion%2Fe3199557-9ae5-4736-b6b1-84cac0662acc%2F8ab09111-ad32-4541-bca3-0d133417b401%2F68bwjq_processed.png&w=3840&q=75)

Trending now
This is a popular solution!
Step by step
Solved in 5 steps with 4 images

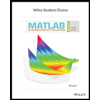
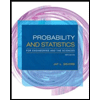
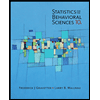
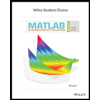
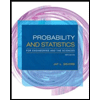
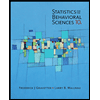
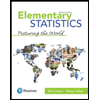
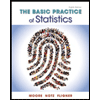
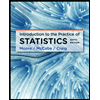