Problem 8: Sample Complexity of Learning with Neural Tangent Kernel (NTK) Statement: Using the Neural Tangent Kernel framework, derive the sample complexity required for a neural network to generalize well on a given learning task. Prove how the sample complexity depends on factors such as the network's depth, width, and the properties of the target function. Key Points for the Proof: • Introduce the Neural Tangent Kernel and its relevance to neural network training dynamics. • Link the NTK to generalization performance and sample complexity. Analyze how architectural parameters influence the NTK and, consequently, the sample complexity. . Provide bounds that relate network properties to the number of samples needed for accurate learning.
Problem 8: Sample Complexity of Learning with Neural Tangent Kernel (NTK) Statement: Using the Neural Tangent Kernel framework, derive the sample complexity required for a neural network to generalize well on a given learning task. Prove how the sample complexity depends on factors such as the network's depth, width, and the properties of the target function. Key Points for the Proof: • Introduce the Neural Tangent Kernel and its relevance to neural network training dynamics. • Link the NTK to generalization performance and sample complexity. Analyze how architectural parameters influence the NTK and, consequently, the sample complexity. . Provide bounds that relate network properties to the number of samples needed for accurate learning.
Elementary Linear Algebra (MindTap Course List)
8th Edition
ISBN:9781305658004
Author:Ron Larson
Publisher:Ron Larson
Chapter5: Inner Product Spaces
Section5.CR: Review Exercises
Problem 62CR
Related questions
Question

Transcribed Image Text:Problem 8: Sample Complexity of Learning with Neural Tangent Kernel
(NTK)
Statement: Using the Neural Tangent Kernel framework, derive the sample complexity required for a
neural network to generalize well on a given learning task. Prove how the sample complexity
depends on factors such as the network's depth, width, and the properties of the target function.
Key Points for the Proof:
•
Introduce the Neural Tangent Kernel and its relevance to neural network training dynamics.
•
Link the NTK to generalization performance and sample complexity.
Analyze how architectural parameters influence the NTK and, consequently, the sample
complexity.
. Provide bounds that relate network properties to the number of samples needed for accurate
learning.
Expert Solution

This question has been solved!
Explore an expertly crafted, step-by-step solution for a thorough understanding of key concepts.
Step by step
Solved in 2 steps with 3 images

Recommended textbooks for you
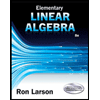
Elementary Linear Algebra (MindTap Course List)
Algebra
ISBN:
9781305658004
Author:
Ron Larson
Publisher:
Cengage Learning
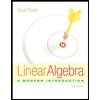
Linear Algebra: A Modern Introduction
Algebra
ISBN:
9781285463247
Author:
David Poole
Publisher:
Cengage Learning
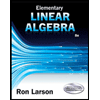
Elementary Linear Algebra (MindTap Course List)
Algebra
ISBN:
9781305658004
Author:
Ron Larson
Publisher:
Cengage Learning
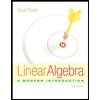
Linear Algebra: A Modern Introduction
Algebra
ISBN:
9781285463247
Author:
David Poole
Publisher:
Cengage Learning