Problem 2: Convergence of Gradient Descent in Over-Parameterized Neural Networks Statement: Consider an over-parameterized neural network (i.e., a network with more parameters than necessary to fit the training data) trained using gradient descent on a squared loss function. Prove that, under appropriate initialization and with a sufficiently small learning rate, gradient descent converges to a global minimum of the loss function. Key Points for the Proof: • • • Define the over-parameterization regime and its implications for the loss landscape. Analyze the dynamics of gradient descent in the high-dimensional parameter space. Use tools from optimization theory to show that all local minima are global minima in this setting. Ensure that the initialization is within the basin of attraction for convergence to a global minimum.
Problem 2: Convergence of Gradient Descent in Over-Parameterized Neural Networks Statement: Consider an over-parameterized neural network (i.e., a network with more parameters than necessary to fit the training data) trained using gradient descent on a squared loss function. Prove that, under appropriate initialization and with a sufficiently small learning rate, gradient descent converges to a global minimum of the loss function. Key Points for the Proof: • • • Define the over-parameterization regime and its implications for the loss landscape. Analyze the dynamics of gradient descent in the high-dimensional parameter space. Use tools from optimization theory to show that all local minima are global minima in this setting. Ensure that the initialization is within the basin of attraction for convergence to a global minimum.
Elementary Linear Algebra (MindTap Course List)
8th Edition
ISBN:9781305658004
Author:Ron Larson
Publisher:Ron Larson
Chapter5: Inner Product Spaces
Section5.CR: Review Exercises
Problem 62CR
Related questions
Question

Transcribed Image Text:Problem 2: Convergence of Gradient Descent in Over-Parameterized Neural
Networks
Statement: Consider an over-parameterized neural network (i.e., a network with more parameters
than necessary to fit the training data) trained using gradient descent on a squared loss function.
Prove that, under appropriate initialization and with a sufficiently small learning rate, gradient
descent converges to a global minimum of the loss function.
Key Points for the Proof:
•
•
•
Define the over-parameterization regime and its implications for the loss landscape.
Analyze the dynamics of gradient descent in the high-dimensional parameter space.
Use tools from optimization theory to show that all local minima are global minima in this
setting.
Ensure that the initialization is within the basin of attraction for convergence to a global
minimum.
Expert Solution

This question has been solved!
Explore an expertly crafted, step-by-step solution for a thorough understanding of key concepts.
Step by step
Solved in 2 steps

Recommended textbooks for you
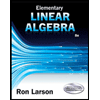
Elementary Linear Algebra (MindTap Course List)
Algebra
ISBN:
9781305658004
Author:
Ron Larson
Publisher:
Cengage Learning
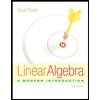
Linear Algebra: A Modern Introduction
Algebra
ISBN:
9781285463247
Author:
David Poole
Publisher:
Cengage Learning
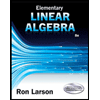
Elementary Linear Algebra (MindTap Course List)
Algebra
ISBN:
9781305658004
Author:
Ron Larson
Publisher:
Cengage Learning
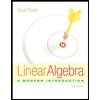
Linear Algebra: A Modern Introduction
Algebra
ISBN:
9781285463247
Author:
David Poole
Publisher:
Cengage Learning