At the beginning of each day, a patient in the hospital is classifed into one of the three conditions: good, fair or critical. At the beginning of the next day, the patient will either still be in the hospital and be in good, fair or critical condition or will be discharged in one of three conditions: improved, unimproved, or dead. The transistion probabilities for this situation are Good Fair Critical Good 0.65 0.20 0.05 Fair 0.50 0.30 0.12 Critical 0.51 0.25 0.20 Improved Unimproved Dead Good 0.06 0.03 0.01 Fair 0.03 0.02 0.03 Critical 0.01 0.01 0.02 For example a patient who begins the day in fair condition has a 12% chance of being in critical condition the next day and a 3% chance of being discharged the next day in improved condition. This moring there were 500 patients in good condition, 300 in fair and 200 in critical. Tomorrow morning the follow admissions will be made: 50 good condtion, 40 fair condition and 30 critical. Predict tomorrow morning's census.
At the beginning of each day, a patient in the hospital is classifed into one of the three conditions: good, fair or critical. At the beginning of the next day, the patient will either still be in the hospital and be in good, fair or critical condition or will be discharged in one of three conditions: improved, unimproved, or dead. The transistion probabilities for this situation are
Good Fair Critical
Good 0.65 0.20 0.05
Fair 0.50 0.30 0.12
Critical 0.51 0.25 0.20
Improved Unimproved Dead
Good 0.06 0.03 0.01
Fair 0.03 0.02 0.03
Critical 0.01 0.01 0.02
For example a patient who begins the day in fair condition has a 12% chance of being in critical condition the next day and a 3% chance of being discharged the next day in improved condition.
This moring there were 500 patients in good condition, 300 in fair and 200 in critical. Tomorrow morning the follow admissions will be made: 50 good condtion, 40 fair condition and 30 critical. Predict tomorrow morning's census.

Trending now
This is a popular solution!
Step by step
Solved in 2 steps with 2 images

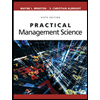
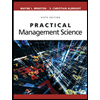