A real estate developer studying the business problem of estimating the consumption of heating oil (gallons per month) has decided to examine the effect of atmospheric temperature (Fahrenheit degree) and the amount of attic insulation (inches) on the oil consumption. Data are collected from a random sample of 15 houses for the moth of January. The regression results for a quadratic regression model, Y = Bo + B1X1 + B2X2 + B3X3 + e, is as follows, at the a= = 0.05 level of significance. Where X₁ is the temperature variable and X2 is the insulation amount variable. The translated squared nsulation amount predictor is X3 = X2. Source Regression Error Total df Sum of Squares Mean Squares F stat 229643.1645 6492.0649 3 ? 11 14 236135.2293 ? ? F crit 3.5874
A real estate developer studying the business problem of estimating the consumption of heating oil (gallons per month) has decided to examine the effect of atmospheric temperature (Fahrenheit degree) and the amount of attic insulation (inches) on the oil consumption. Data are collected from a random sample of 15 houses for the moth of January. The regression results for a quadratic regression model, Y = Bo + B1X1 + B2X2 + B3X3 + e, is as follows, at the a= = 0.05 level of significance. Where X₁ is the temperature variable and X2 is the insulation amount variable. The translated squared nsulation amount predictor is X3 = X2. Source Regression Error Total df Sum of Squares Mean Squares F stat 229643.1645 6492.0649 3 ? 11 14 236135.2293 ? ? F crit 3.5874
MATLAB: An Introduction with Applications
6th Edition
ISBN:9781119256830
Author:Amos Gilat
Publisher:Amos Gilat
Chapter1: Starting With Matlab
Section: Chapter Questions
Problem 1P
Related questions
Question

Transcribed Image Text:A real estate developer studying the business problem of estimating the
consumption of heating oil (gallons per month) has decided to examine the
effect of atmospheric temperature (Fahrenheit degree) and the amount of
attic insulation (inches) on the oil consumption. Data are collected from a
random sample of 15 houses for the moth of January. The regression results
for a quadratic regression model, Y = Bo + B1X1 + B₂X2 + B3X3 + €, is as
follows, at the a = 0.05 level of significance. Where X₁ is the temperature
variable and X2 is the insulation amount variable. The translated squared
insulation amount predictor is X3 = X2/1.
Source. df Sum of Squares Mean Squares F stat F crit
3.5874
3
?
Regression
Error
11
Total
14
Variable
Intercept
Temperature
Insulation
Insulation²
229643.1645
6492.0649
236135.2293
?
?
Coefficient St. Error Lower 95%
624.5864 42.4352
-5.3626
0.3171
-44.5868
14.9547
1.8667
1.1238
531.1872
?
?
?
Upper 95%
717.9856
?
?
?
R-Square= 0.9725, Adjusted R-Square= 0.9650, Standard Error of
Prediction = 24.2938, Number of Observations = 15
a) Conduct the F-Test for this regression model at the 5% level of sig-
nificance and decide if this model is a good fit for this data set and explain
why.

Transcribed Image Text:b) Construct the 95% confidence interval estimator for the partial slope,
for all of the predictors here. Investigate and explain which ones of these
predictors are needed and which ones of these predictors are not needed.
c) Explain the predictive power (R-Square) of this regression equation
for the oil consumption prediction.
d) Explain the standard error of prediction for this regression equation
of the oil consumption prediction.
Expert Solution

This question has been solved!
Explore an expertly crafted, step-by-step solution for a thorough understanding of key concepts.
This is a popular solution!
Trending now
This is a popular solution!
Step by step
Solved in 3 steps with 1 images

Recommended textbooks for you
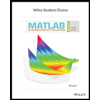
MATLAB: An Introduction with Applications
Statistics
ISBN:
9781119256830
Author:
Amos Gilat
Publisher:
John Wiley & Sons Inc
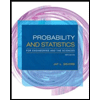
Probability and Statistics for Engineering and th…
Statistics
ISBN:
9781305251809
Author:
Jay L. Devore
Publisher:
Cengage Learning
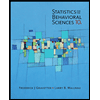
Statistics for The Behavioral Sciences (MindTap C…
Statistics
ISBN:
9781305504912
Author:
Frederick J Gravetter, Larry B. Wallnau
Publisher:
Cengage Learning
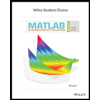
MATLAB: An Introduction with Applications
Statistics
ISBN:
9781119256830
Author:
Amos Gilat
Publisher:
John Wiley & Sons Inc
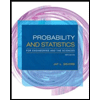
Probability and Statistics for Engineering and th…
Statistics
ISBN:
9781305251809
Author:
Jay L. Devore
Publisher:
Cengage Learning
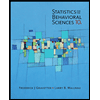
Statistics for The Behavioral Sciences (MindTap C…
Statistics
ISBN:
9781305504912
Author:
Frederick J Gravetter, Larry B. Wallnau
Publisher:
Cengage Learning
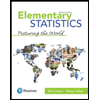
Elementary Statistics: Picturing the World (7th E…
Statistics
ISBN:
9780134683416
Author:
Ron Larson, Betsy Farber
Publisher:
PEARSON
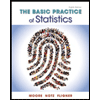
The Basic Practice of Statistics
Statistics
ISBN:
9781319042578
Author:
David S. Moore, William I. Notz, Michael A. Fligner
Publisher:
W. H. Freeman
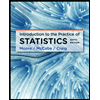
Introduction to the Practice of Statistics
Statistics
ISBN:
9781319013387
Author:
David S. Moore, George P. McCabe, Bruce A. Craig
Publisher:
W. H. Freeman