*7. In this problem, you'll find MLES for the Gaussian simple linear regression model. Let N (Bo + B1xi, o²) for i = 1,..., n, where x; are fixed 'covariates'. Since the Y;'s indep. Y; are independent and the density of Y; is 1 exp { 1 f (yi; Bo, B1, o²) = %3D V2no2 202 the likelihood function is { 1 L (Bo, B1, 0²; y:) II f (yi; ßo, P1, o²) = (27o²)¯ , { (di – Bo – B1æ:)² exp 202 i=1 i=1 (a) Write the log-likelihood € (Bo, ß1,0²; y;) = log L (Bo, B1,0²;y;). (b) Find e (Bo, B1,0²; y;). (c) Find e (Bo, B1,0²; y;). (d) Find e (Bo, B1,0²; y;). aß1 (e) Solve the system of equations aBo e (Bo, B1, o²; y:) = 0 e (Во, 30, B1, 0²; y;) = 0 e (Bo, B1, o²; y;) = 0 for Bo, B1,0² to obtain Bo = j – B1ữ E=1 TiYi – nyā B, = 1 E(yi – Bo – B1x:)² i=1 Comment: to verify that these solutions jointly specify a maximum, one must show that the matrix of second-order partial derivatives (known as the Hessian) is negative definite at the solutions. This is the multivariate analogue of the second derivative test. You are not expected to know how to do this.
*7. In this problem, you'll find MLES for the Gaussian simple linear regression model. Let N (Bo + B1xi, o²) for i = 1,..., n, where x; are fixed 'covariates'. Since the Y;'s indep. Y; are independent and the density of Y; is 1 exp { 1 f (yi; Bo, B1, o²) = %3D V2no2 202 the likelihood function is { 1 L (Bo, B1, 0²; y:) II f (yi; ßo, P1, o²) = (27o²)¯ , { (di – Bo – B1æ:)² exp 202 i=1 i=1 (a) Write the log-likelihood € (Bo, ß1,0²; y;) = log L (Bo, B1,0²;y;). (b) Find e (Bo, B1,0²; y;). (c) Find e (Bo, B1,0²; y;). (d) Find e (Bo, B1,0²; y;). aß1 (e) Solve the system of equations aBo e (Bo, B1, o²; y:) = 0 e (Во, 30, B1, 0²; y;) = 0 e (Bo, B1, o²; y;) = 0 for Bo, B1,0² to obtain Bo = j – B1ữ E=1 TiYi – nyā B, = 1 E(yi – Bo – B1x:)² i=1 Comment: to verify that these solutions jointly specify a maximum, one must show that the matrix of second-order partial derivatives (known as the Hessian) is negative definite at the solutions. This is the multivariate analogue of the second derivative test. You are not expected to know how to do this.
MATLAB: An Introduction with Applications
6th Edition
ISBN:9781119256830
Author:Amos Gilat
Publisher:Amos Gilat
Chapter1: Starting With Matlab
Section: Chapter Questions
Problem 1P
Related questions
Question
![**Title: Maximum Likelihood Estimation for Gaussian Simple Linear Regression**
In this problem, you'll find the Maximum Likelihood Estimates (MLEs) for the Gaussian simple linear regression model. Let \( Y_i \sim \text{indep. } N (\beta_0 + \beta_1 x_i, \sigma^2) \) for \( i = 1, \ldots, n \), where \( x_i \) are fixed 'covariates'. Since the \( Y_i \)'s are independent, the density of \( Y_i \) is
\[
f(y_i; \beta_0, \beta_1, \sigma^2) = \frac{1}{\sqrt{2\pi \sigma^2}} \exp \left\{ -\frac{1}{2\sigma^2} (y_i - \beta_0 - \beta_1 x_i)^2 \right\}
\]
The likelihood function is
\[
\mathcal{L} (\beta_0, \beta_1, \sigma^2; y_i) = \prod_{i=1}^{n} f(y_i; \beta_0, \beta_1, \sigma^2) = (2\pi \sigma^2)^{-\frac{n}{2}} \exp \left\{ -\frac{1}{2\sigma^2} \sum_{i=1}^{n} (y_i - \beta_0 - \beta_1 x_i)^2 \right\}
\]
(a) **Log-Likelihood Function:**
Write the log-likelihood \( \ell (\beta_0, \beta_1, \sigma^2; y_i) = \log \mathcal{L} (\beta_0, \beta_1, \sigma^2; y_i) \).
(b) **Partial Derivative with respect to \( \beta_0 \):**
Find \( \frac{\partial}{\partial \beta_0} \ell (\beta_0, \beta_1, \sigma^2; y_i) \).
(c) **Partial Derivative with respect to \( \beta_1 \):**
Find \( \frac{\partial}{\partial \beta_1} \ell (\beta_0, \beta_1, \sigma^2; y_i) \).
(d) **Partial](/v2/_next/image?url=https%3A%2F%2Fcontent.bartleby.com%2Fqna-images%2Fquestion%2F544a9f38-3e68-4113-87a7-993914a696cb%2F818af393-629c-4279-a7e2-78f27df5345c%2Fx3f8u4r_processed.png&w=3840&q=75)
Transcribed Image Text:**Title: Maximum Likelihood Estimation for Gaussian Simple Linear Regression**
In this problem, you'll find the Maximum Likelihood Estimates (MLEs) for the Gaussian simple linear regression model. Let \( Y_i \sim \text{indep. } N (\beta_0 + \beta_1 x_i, \sigma^2) \) for \( i = 1, \ldots, n \), where \( x_i \) are fixed 'covariates'. Since the \( Y_i \)'s are independent, the density of \( Y_i \) is
\[
f(y_i; \beta_0, \beta_1, \sigma^2) = \frac{1}{\sqrt{2\pi \sigma^2}} \exp \left\{ -\frac{1}{2\sigma^2} (y_i - \beta_0 - \beta_1 x_i)^2 \right\}
\]
The likelihood function is
\[
\mathcal{L} (\beta_0, \beta_1, \sigma^2; y_i) = \prod_{i=1}^{n} f(y_i; \beta_0, \beta_1, \sigma^2) = (2\pi \sigma^2)^{-\frac{n}{2}} \exp \left\{ -\frac{1}{2\sigma^2} \sum_{i=1}^{n} (y_i - \beta_0 - \beta_1 x_i)^2 \right\}
\]
(a) **Log-Likelihood Function:**
Write the log-likelihood \( \ell (\beta_0, \beta_1, \sigma^2; y_i) = \log \mathcal{L} (\beta_0, \beta_1, \sigma^2; y_i) \).
(b) **Partial Derivative with respect to \( \beta_0 \):**
Find \( \frac{\partial}{\partial \beta_0} \ell (\beta_0, \beta_1, \sigma^2; y_i) \).
(c) **Partial Derivative with respect to \( \beta_1 \):**
Find \( \frac{\partial}{\partial \beta_1} \ell (\beta_0, \beta_1, \sigma^2; y_i) \).
(d) **Partial
Expert Solution

This question has been solved!
Explore an expertly crafted, step-by-step solution for a thorough understanding of key concepts.
Step by step
Solved in 3 steps with 3 images

Recommended textbooks for you
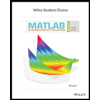
MATLAB: An Introduction with Applications
Statistics
ISBN:
9781119256830
Author:
Amos Gilat
Publisher:
John Wiley & Sons Inc
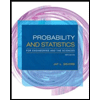
Probability and Statistics for Engineering and th…
Statistics
ISBN:
9781305251809
Author:
Jay L. Devore
Publisher:
Cengage Learning
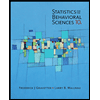
Statistics for The Behavioral Sciences (MindTap C…
Statistics
ISBN:
9781305504912
Author:
Frederick J Gravetter, Larry B. Wallnau
Publisher:
Cengage Learning
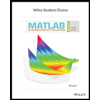
MATLAB: An Introduction with Applications
Statistics
ISBN:
9781119256830
Author:
Amos Gilat
Publisher:
John Wiley & Sons Inc
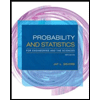
Probability and Statistics for Engineering and th…
Statistics
ISBN:
9781305251809
Author:
Jay L. Devore
Publisher:
Cengage Learning
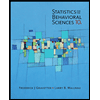
Statistics for The Behavioral Sciences (MindTap C…
Statistics
ISBN:
9781305504912
Author:
Frederick J Gravetter, Larry B. Wallnau
Publisher:
Cengage Learning
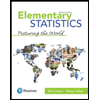
Elementary Statistics: Picturing the World (7th E…
Statistics
ISBN:
9780134683416
Author:
Ron Larson, Betsy Farber
Publisher:
PEARSON
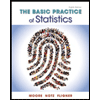
The Basic Practice of Statistics
Statistics
ISBN:
9781319042578
Author:
David S. Moore, William I. Notz, Michael A. Fligner
Publisher:
W. H. Freeman
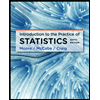
Introduction to the Practice of Statistics
Statistics
ISBN:
9781319013387
Author:
David S. Moore, George P. McCabe, Bruce A. Craig
Publisher:
W. H. Freeman