1234567 Super Bike Company (SBC) is a whole sale distributor of bicycles. Its distribution center in the town serves many retail stores within a 200-mile radius of the town. The data of bicycles ordered by the stores each month (i.e., monthly bicycle demand) during the last 5 years (year 1 year 5) is shown in Table 1 below. The analyst, Harry thought there may be a pattern of seasonal variation within the year, and decided to use the multiplicative seasonal variation method to forecast monthly demands of bicycles for next two years, year 6 and year 7. Harry started with calculating the aggregate demands, the annual demands for each of the past 5 years in order to forecast the annual demands for BOTH year 6 and year 7. Several approaches were considered for this purpose: (a) Naive approach (b) 3-year moving average 10 (c) Trend projection (d) Exponetial smoothing with trend, where Year 1's forecast = 12,000 units, Year 1's trend = 0, smoothing constant for forecast average = 0.6, and smoothing constant for trend = 0.3 12 (e) Associate method (i.e. simple linear regression) by relating bicycle annual demands to ad expense at the regional retail stores each year (see Tables 2.1 and 2.1) 13 14 Answer the following questions, Q1 ~ Q3. 15 16 16 17 18 Table 1 Q1: Select the applicable approach(es) from (a)~(e) that are able to meet Harry's purpose. Present the forecasted annual demads of year 6 and year 7 using the approach(es) you select. Table 2.1 19 Bicycles demands Ad expense (in $000) 20 21 Month 1 1244 Year 1 49.3 Month 2 878 Year 2 32.6 Month 3 1053 Year 3 41.6 Month 4 1080 Year 4 50.1 24 Year 1 Month 5 1541 Year 5 44.0 Month 6 871 Month 1001 Month 8 1265 Table 2.2 28 Month 9 879 Ad expense expected during 29 Month 10 1147 the year (in $000). 30 Month 11 988 Year 6 31 Month 12 1355 Year 7 45.0 48.1 32 Month 1 1200 33 Month 2 1046 1046 34 Month. 1025 1025 971 Month 4 8/1 26 Year 2 Month 5 Month 5 11/3 Month 6 1260 Month 6 1269 Month 7 909 Month 7 808 Month 8 Month 8 1396 1396 Month 9 Month 9 1030 1030 Month 10 962 963 42 Month 11 899 12 Month 12 1034 44 Month 1 1555 1339 45 Month 2 1164 Month 3 991 Month 4 1009 Year 3 Month 5 1148 Month 1037 Month 986 Month 8 1453 Month 9 1093 Month 10 1003 Month 11 Month 11 922 Month 12 Month 12 1406 Month Month 1 1541 1941 Month 2 1058 Month 3 1074 Month 4 959 Year 4 Month 5 1526 Month 6 1052 Month 7 903 Month 1127 Month 9 1057 Month 10 1086 Month 11 999 Month 12 1243 Month 1 1342 Month 2 1225 1225 Month 3 1015 1015 Month 4 1007 1007 Year 5 Month Month S 5 1562 1562 Month 6 1109 1105 Month Month 884 Month 1111 Month 9 1081 Month 10 907 78 Month 11 1067 79 Month 12 1143 80 81 Q2: For each approach you selected in Q1, calculate MAD to show its overall forecast error. Q3: Apparently, you will use the forecasts in Q1 from the approach with the least overall fortecast error found in Q2, to further calculate the monthly demand forecasts for year 6 and year 7, respectively. Show your calculation and result below.
1234567 Super Bike Company (SBC) is a whole sale distributor of bicycles. Its distribution center in the town serves many retail stores within a 200-mile radius of the town. The data of bicycles ordered by the stores each month (i.e., monthly bicycle demand) during the last 5 years (year 1 year 5) is shown in Table 1 below. The analyst, Harry thought there may be a pattern of seasonal variation within the year, and decided to use the multiplicative seasonal variation method to forecast monthly demands of bicycles for next two years, year 6 and year 7. Harry started with calculating the aggregate demands, the annual demands for each of the past 5 years in order to forecast the annual demands for BOTH year 6 and year 7. Several approaches were considered for this purpose: (a) Naive approach (b) 3-year moving average 10 (c) Trend projection (d) Exponetial smoothing with trend, where Year 1's forecast = 12,000 units, Year 1's trend = 0, smoothing constant for forecast average = 0.6, and smoothing constant for trend = 0.3 12 (e) Associate method (i.e. simple linear regression) by relating bicycle annual demands to ad expense at the regional retail stores each year (see Tables 2.1 and 2.1) 13 14 Answer the following questions, Q1 ~ Q3. 15 16 16 17 18 Table 1 Q1: Select the applicable approach(es) from (a)~(e) that are able to meet Harry's purpose. Present the forecasted annual demads of year 6 and year 7 using the approach(es) you select. Table 2.1 19 Bicycles demands Ad expense (in $000) 20 21 Month 1 1244 Year 1 49.3 Month 2 878 Year 2 32.6 Month 3 1053 Year 3 41.6 Month 4 1080 Year 4 50.1 24 Year 1 Month 5 1541 Year 5 44.0 Month 6 871 Month 1001 Month 8 1265 Table 2.2 28 Month 9 879 Ad expense expected during 29 Month 10 1147 the year (in $000). 30 Month 11 988 Year 6 31 Month 12 1355 Year 7 45.0 48.1 32 Month 1 1200 33 Month 2 1046 1046 34 Month. 1025 1025 971 Month 4 8/1 26 Year 2 Month 5 Month 5 11/3 Month 6 1260 Month 6 1269 Month 7 909 Month 7 808 Month 8 Month 8 1396 1396 Month 9 Month 9 1030 1030 Month 10 962 963 42 Month 11 899 12 Month 12 1034 44 Month 1 1555 1339 45 Month 2 1164 Month 3 991 Month 4 1009 Year 3 Month 5 1148 Month 1037 Month 986 Month 8 1453 Month 9 1093 Month 10 1003 Month 11 Month 11 922 Month 12 Month 12 1406 Month Month 1 1541 1941 Month 2 1058 Month 3 1074 Month 4 959 Year 4 Month 5 1526 Month 6 1052 Month 7 903 Month 1127 Month 9 1057 Month 10 1086 Month 11 999 Month 12 1243 Month 1 1342 Month 2 1225 1225 Month 3 1015 1015 Month 4 1007 1007 Year 5 Month Month S 5 1562 1562 Month 6 1109 1105 Month Month 884 Month 1111 Month 9 1081 Month 10 907 78 Month 11 1067 79 Month 12 1143 80 81 Q2: For each approach you selected in Q1, calculate MAD to show its overall forecast error. Q3: Apparently, you will use the forecasts in Q1 from the approach with the least overall fortecast error found in Q2, to further calculate the monthly demand forecasts for year 6 and year 7, respectively. Show your calculation and result below.
Practical Management Science
6th Edition
ISBN:9781337406659
Author:WINSTON, Wayne L.
Publisher:WINSTON, Wayne L.
Chapter2: Introduction To Spreadsheet Modeling
Section: Chapter Questions
Problem 20P: Julie James is opening a lemonade stand. She believes the fixed cost per week of running the stand...
Related questions
Question
Please walk me step by step - I believe for Q1 the only ones I can do is (c), (d) and (e) but if there's more pls assist with that as well. Thank you so much

Transcribed Image Text:1234567
Super Bike Company (SBC) is a whole sale distributor of bicycles. Its distribution center in the town serves many retail stores within a 200-mile radius of the town. The data of bicycles ordered by the stores each month (i.e.,
monthly bicycle demand) during the last 5 years (year 1 year 5) is shown in Table 1 below. The analyst, Harry thought there may be a pattern of seasonal variation within the year, and decided to use the multiplicative
seasonal variation method to forecast monthly demands of bicycles for next two years, year 6 and year 7.
Harry started with calculating the aggregate demands, the annual demands for each of the past 5 years in order to forecast the annual demands for BOTH year 6 and year 7. Several approaches were considered for this
purpose:
(a) Naive approach
(b) 3-year moving average
10 (c) Trend projection
(d) Exponetial smoothing with trend, where Year 1's forecast = 12,000 units, Year 1's trend = 0, smoothing constant for forecast average = 0.6, and smoothing constant for trend = 0.3
12
(e) Associate method (i.e. simple linear regression) by relating bicycle annual demands to ad expense at the regional retail stores each year (see Tables 2.1 and 2.1)
13
14
Answer the following questions, Q1 ~ Q3.
15
16
16
17
18 Table 1
Q1: Select the applicable approach(es) from (a)~(e) that are able to meet Harry's purpose. Present the forecasted annual demads of year 6
and year 7 using the approach(es) you select.
Table 2.1
19 Bicycles demands
Ad expense (in $000)
20
21
Month 1
1244
Year 1
49.3
Month 2
878
Year 2
32.6
Month 3
1053
Year 3
41.6
Month 4
1080
Year 4
50.1
24
Year 1
Month 5
1541
Year 5
44.0
Month 6
871
Month
1001
Month 8
1265
Table 2.2
28
Month 9
879
Ad expense expected during
29
Month 10
1147
the year (in $000).
30
Month 11
988
Year 6
31
Month 12
1355
Year 7
45.0
48.1
32
Month
1
1200
33
Month 2
1046
1046
34
Month.
1025
1025
971
Month 4
8/1
26
Year 2
Month 5
Month 5
11/3
Month 6
1260
Month 6
1269
Month 7
909
Month 7
808
Month 8
Month 8
1396
1396
Month 9
Month 9
1030
1030
Month 10
962
963
42
Month 11
899
12
Month 12
1034
44
Month 1
1555
1339
45
Month 2
1164
Month 3
991
Month 4
1009
Year 3
Month 5
1148
Month
1037
Month
986
Month 8
1453
Month 9
1093
Month 10
1003
Month 11
Month 11
922
Month 12
Month 12
1406
Month
Month 1
1541
1941
Month 2
1058
Month 3
1074
Month 4
959
Year 4
Month 5
1526
Month 6
1052
Month 7
903
Month
1127
Month 9
1057
Month 10
1086
Month 11
999
Month 12
1243
Month 1
1342
Month 2
1225
1225
Month 3
1015
1015
Month 4
1007
1007
Year 5
Month
Month S
5
1562
1562
Month 6
1109
1105
Month
Month
884
Month
1111
Month 9
1081
Month 10
907
78
Month 11
1067
79
Month 12
1143
80
81
Q2: For each approach you selected in Q1, calculate MAD to show its overall forecast error.
Q3: Apparently, you will use the forecasts in Q1 from the approach with the least overall fortecast error found in Q2, to further calculate the
monthly demand forecasts for year 6 and year 7, respectively. Show your calculation and result below.
Expert Solution

This question has been solved!
Explore an expertly crafted, step-by-step solution for a thorough understanding of key concepts.
Step by step
Solved in 2 steps

Recommended textbooks for you
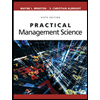
Practical Management Science
Operations Management
ISBN:
9781337406659
Author:
WINSTON, Wayne L.
Publisher:
Cengage,
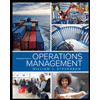
Operations Management
Operations Management
ISBN:
9781259667473
Author:
William J Stevenson
Publisher:
McGraw-Hill Education
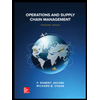
Operations and Supply Chain Management (Mcgraw-hi…
Operations Management
ISBN:
9781259666100
Author:
F. Robert Jacobs, Richard B Chase
Publisher:
McGraw-Hill Education
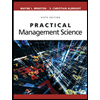
Practical Management Science
Operations Management
ISBN:
9781337406659
Author:
WINSTON, Wayne L.
Publisher:
Cengage,
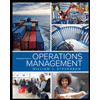
Operations Management
Operations Management
ISBN:
9781259667473
Author:
William J Stevenson
Publisher:
McGraw-Hill Education
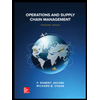
Operations and Supply Chain Management (Mcgraw-hi…
Operations Management
ISBN:
9781259666100
Author:
F. Robert Jacobs, Richard B Chase
Publisher:
McGraw-Hill Education
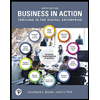
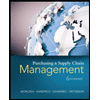
Purchasing and Supply Chain Management
Operations Management
ISBN:
9781285869681
Author:
Robert M. Monczka, Robert B. Handfield, Larry C. Giunipero, James L. Patterson
Publisher:
Cengage Learning
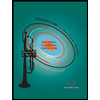
Production and Operations Analysis, Seventh Editi…
Operations Management
ISBN:
9781478623069
Author:
Steven Nahmias, Tava Lennon Olsen
Publisher:
Waveland Press, Inc.