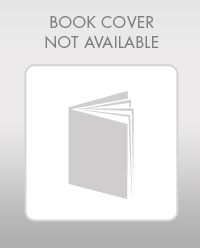
Annualized Rate Occurrence (ARO):
Annualized Rate Occurrence is the estimated frequency at which a given threat is expected to happen.
ARO can be calculated by using the following formula:
Annualized Loss Expectancy (ALE):
Annualized Loss Expectancy is the loss expected from the attack of a specific information asset which has been carried over for a year. It is a product of single loss expectancy and the annualized rate of occurrence.
ALE can be calculated by using the following formula:
Cost-Benefit Analysis (CBA):
- CBA is the study that determines the cost required for protecting an asset.
- It is a process of feasibility which is carried with a formal documentation process. It is also called as economic feasibility study.
- System value is an estimated total cost of the organization in terms of the cost of equipment, and more important, in terms of the cost of information stored in the system.
CBA can be calculated by using the following formula:
Here, the term

Explanation of Solution
Calculate ARO for Programmer mistakes:
Substitute the value of “One year” as “365” and “Frequency of occurrence (One per months)” as “
Hence, the ARO for programmer mistakes is “12 (approximately)”.
Calculate ARO for Loss if intellectual property:
Substitute the value of “One year” as “365” and “Frequency of occurrence (One per 10 years)” as “
Hence, the ARO for Loss if intellectual property is “0.5 (approximately)”.
Calculate ARO for Software Piracy:
Substitute the value of “One year” as “365” and “Frequency of occurrence (One per months)” as “
Hence, the ARO for Software Piracy is “12 (approximately)”.
Calculate ARO for Theft of information (hacker):
Substitute the value of “One year” as “365” and “Frequency of occurrence (One per 6 months)” as “
Hence, the ARO for Theft of information (hacker) is “2 (approximately)”.
Calculate ARO for Theft of information (employee):
Substitute the value of “One year” as “365” and “Frequency of occurrence (One per year)” as “365” in the equation (1).
Hence, the ARO for Theft of Theft of information (employee) is “1 (approximately)”.
Calculate ARO for Web defacement:
Substitute the value of “One year” as “365” and “Frequency of occurrence (One per quarter)” as “
Hence, the ARO for Web defacement is “4 (approximately)”.
Calculate ARO for Theft of equipment:
Substitute the value of “One year” as “365” and “Frequency of occurrence (One per 10 years)” as “
Hence, the ARO for Theft of equipment is “0.5 (approximately)”.
Calculate ARO for Viruses, worms, Trojan Horses:
Substitute the value of “One year” as “365” and “Frequency of occurrence (One per months)” as “
Hence, the ARO for Viruses, worms, Trojan Horses is “12 (approximately)”.
Calculate ARO for Denial-of-service attacks:
Substitute the value of “One year” as “365” and “Frequency of occurrence (One per 6 months)” as “
Hence, the ARO for Denial-of-service attacks is “2 (approximately)”.
Calculate ARO for Earthquake:
Substitute the value of “One year” as “365” and “Frequency of occurrence (One per 20 years)” as “
Hence, the ARO for Earthquake is “0.05 (approximately)”.
Calculate ARO for Food:
Substitute the value of “One year” as “365” and “Frequency of occurrence (One per 10 years)” as “
Hence, the ARO for Food is “0.1 (approximately)”.
Calculate ARO for Fire:
Substitute the value of “One year” as “365” and “Frequency of occurrence (One per 10 years)” as “
Hence, the ARO for Fire is “0.1 (approximately)”.
Calculate ALE for Programmer mistakes:
Substitute the value of “SLE” as “5000” and “ARO” as “12” in the equation (2).
Hence, the ALE for programmer mistakes is “60000”.
Calculate ALE for Loss if intellectual property:
Substitute the value of “SLE” as “75000” and “ARO” as “0.5” in the equation (2).
Hence, the ALE for Loss if intellectual property is “37500”.
Calculate ALE for Software Piracy:
Substitute the value of “SLE” as “500” and “ARO” as “12” in the equation (2).
Hence, the ALE for Software Piracy is “6000”.
Calculate ALE for Theft of information(hacker):
Substitute the value of “SLE” as “2500” and “ARO” as “2” in the equation (2).
Hence, the ALE for Theft of information (hacker)is “5000”.
Calculate ALE for Theft of information (employee)
Substitute the value of “SLE” as “5000” and “ARO” as “1” in the equation (2).
Hence, the ALE for Theft of information (employee) is “5000”.
Calculate ALE for Web defacement:
Substitute the value of “SLE” as “500” and “ARO” as “4” in the equation (2).
Hence, the ALE for Web defacement is “2000”.
Calculate ALE for Theft of equipment:
Substitute the value of “SLE” as “5000” and “ARO” as “0.5” in the equation (2).
Hence, the ALE for Theft of equipment is “2500”.
Calculate ALE for Viruses, worms, Trojan Horses:
Substitute the value of “SLE” as “1500” and “ARO” as “12” in the equation (2).
Hence, the ALE for Viruses, worms, Trojan Horses is “18000”.
Calculate ALE for Denial-of-service attacks:
Substitute the value of “SLE” as “2500” and “ARO” as “2” in the equation (2).
Hence, the ALE for Denial-of-service attacks is “5000”.
Calculate ALE for Earthquake:
Substitute the value of “SLE” as “250000” and “ARO” as “0.05” in the equation (2).
Hence, the ALE for Earthquake is “12500”.
Calculate ALE for Food:
Substitute the value of “SLE” as “50000” and “ARO” as “0.1” in the equation (2).
Hence, the ALE for Food is “5000”.
Calculate ALE for Fire:
Substitute the value of “SLE” as “100000” and “ARO” as “0.1” in the equation (2).
Hence, the ALE for Fire is “10000”.
To calculate CBA for Programmer mistakes:
Substitute the value of “ALE (prior)” as “260000” and “ALE (post)” as “60000” and “ACS” as “20000” in the equation (3).
Hence, the CBA for programmer mistakes is “180000”.
To calculate CBA for Loss if intellectual property:
Substitute the value of “ALE (prior)” as “75000” and “ALE (post)” as “37500” and “ACS” as “15000” in the equation (3).
Hence, the CBA for Loss if intellectual property is “22500”.
To calculate CBA for Software Piracy:
Substitute the value of “ALE (prior)” as “26000” and “ALE (post)” as “6000” and “ACS” as “30000” in the equation (3).
Hence, the CBA for Software Piracy is “-10000”.
To calculate CBA for Theft of information (hacker):
Substitute the value of “ALE (prior)” as “10000” and “ALE (post)” as “5000” and “ACS” as “15000” in the equation (3).
Hence, the CBA for Theft of information (hacker) is “-10000”.
To calculate CBA for Theft of information (employee):
Substitute the value of “ALE (prior)” as “10000” and “ALE (post)” as “5000” and “ACS” as “15000” in the equation (3).
Hence, the CBA for Theft of information (employee) is “-10000”.
To calculate CBA for Web defacement:
Substitute the value of “ALE (prior)” as “6000” and “ALE (post)” as “2000” and “ACS” as “10000” in the equation (3).
Hence, the CBA for Web defacement is “-6000”.
To calculate CBA for Theft of equipment:
Substitute the value of “ALE (prior)” as “5000” and “ALE (post)” as “2500” and “ACS” as “15000” in the equation (3).
Hence, the CBA for Theft of equipment is “-12500”.
To calculate CBA for Viruses, worms, Trojan Horses:
Substitute the value of “ALE (prior)” as “78000” and “ALE (post)” as “18000” and “ACS” as “15000” in the equation (3).
Hence, the CBA for Viruses, worms, Trojan Horses is “45000”.
To calculate CBA for Denial-of-service attacks:
Substitute the value of “ALE (prior)” as “10000” and “ALE (post)” as “5000” and “ACS” as “10000” in the equation (3).
Hence, the CBA for Denial-of-service attacks is “-5000”.
To calculate CBA for Earthquake:
Substitute the value of “ALE (prior)” as “12500” and “ALE (post)” as “12500” and “ACS” as “5000” in the equation (3).
Hence, the CBA for Earthquake is “-5000”.
To calculate CBA for Food:
Substitute the value of “ALE (prior)” as “25000” and “ALE (post)” as “5000” and “ACS” as “10000” in the equation (3).
Hence, the CBA for Food is “10000”.
To calculate CBA for Fire:
Substitute the value of “ALE (prior)” as “50000” and “ALE (post)” as “10000” and “ACS” as “10000” in the equation (3).
Hence, the CBA for Fire is “30000”.
ARO and ALE table for all the threat cost is given below:
ARO and ALE threats | SLE | ARO | ALE | CBA |
Programmer mistakes | 5,000 | 12 | 60,000 | 180,000 |
Loss if intellectual property | 75,000 | 0.5 | 37,500 | 22,500 |
Software Piracy | 500 | 12 | 6,000 | -10,000 |
Theft of information(hacker) | 2,500 | 2 | 5,000 | -10,000 |
Theft of information (employee) | 5,000 | 1 | 5,000 | -10,000 |
Web defacement | 500 | 4 | 2,000 | -6,000 |
Theft of equipment | 5,000 | 0.5 | 2,500 | -12,500 |
Viruses, worms, Trojan Horses | 1,500 | 12 | 18,000 | 45,000 |
Denial-of-service attacks | 2,500 | 2 | 5,000 | -5000 |
Earthquake | 250,000 | 0.05 | 12,500 | -5,000 |
Food | 50,000 | 0.1 | 5,000 | 10,000 |
Fire | 100,000 | 0.1 | 10,000 | 30,000 |
Reason for changes in values:
Some values have been changed because of the implementation controls which had a positive impact on protection of XYZ’s assets. Thus, reducing the frequency of occurrences. However, the controls did not decrease cost for a single incident because the importance of an asset will stay the same and cost XYZ the same amount of time and money to replace. The costs that are listed are worth when the controls are in their place.
Want to see more full solutions like this?
Chapter 5 Solutions
Bundle: Principles of Information Security, Loose-Leaf Version, 6th + MindTap Information Security, 1 term (6 months) Printed Access Card
- 1. Complete the routing table for R2 as per the table shown below when implementing RIP routing Protocol? (14 marks) 195.2.4.0 130.10.0.0 195.2.4.1 m1 130.10.0.2 mo R2 R3 130.10.0.1 195.2.5.1 195.2.5.0 195.2.5.2 195.2.6.1 195.2.6.0 m2 130.11.0.0 130.11.0.2 205.5.5.0 205.5.5.1 R4 130.11.0.1 205.5.6.1 205.5.6.0arrow_forwardAnalyze the charts and introduce each charts by describing each. Identify the patterns in the given data. And determine how are the data points are related. Refer to the raw data (table):arrow_forward3A) Generate a hash table for the following values: 11, 9, 6, 28, 19, 46, 34, 14. Assume the table size is 9 and the primary hash function is h(k) = k % 9. i) Hash table using quadratic probing ii) Hash table with a secondary hash function of h2(k) = 7- (k%7) 3B) Demonstrate with a suitable example, any three possible ways to remove the keys and yet maintaining the properties of a B-Tree. 3C) Differentiate between Greedy and Dynamic Programming.arrow_forward
- What are the charts (with their title name) that could be use to illustrate the data? Please give picture examples.arrow_forwardA design for a synchronous divide-by-six Gray counter isrequired which meets the following specification.The system has 2 inputs, PAUSE and SKIP:• While PAUSE and SKIP are not asserted (logic 0), thecounter continually loops through the Gray coded binarysequence {0002, 0012, 0112, 0102, 1102, 1112}.• If PAUSE is asserted (logic 1) when the counter is onnumber 0102, it stays here until it becomes unasserted (atwhich point it continues counting as before).• While SKIP is asserted (logic 1), the counter misses outodd numbers, i.e. it loops through the sequence {0002,0112, 1102}.The system has 4 outputs, BIT3, BIT2, BIT1, and WAITING:• BIT3, BIT2, and BIT1 are unconditional outputsrepresenting the current number, where BIT3 is the mostsignificant-bit and BIT1 is the least-significant-bit.• An active-high conditional output WAITING should beasserted (logic 1) whenever the counter is paused at 0102.(a) Draw an ASM chart for a synchronous system to providethe functionality described above.(b)…arrow_forwardS A B D FL I C J E G H T K L Figure 1: Search tree 1. Uninformed search algorithms (6 points) Based on the search tree in Figure 1, provide the trace to find a path from the start node S to a goal node T for the following three uninformed search algorithms. When a node has multiple successors, use the left-to-right convention. a. Depth first search (2 points) b. Breadth first search (2 points) c. Iterative deepening search (2 points)arrow_forward
- We want to get an idea of how many tickets we have and what our issues are. Print the ticket ID number, ticket description, ticket priority, ticket status, and, if the information is available, employee first name assigned to it for our records. Include all tickets regardless of whether they have been assigned to an employee or not. Sort it alphabetically by ticket status, and then numerically by ticket ID, with the lower ticket IDs on top.arrow_forwardFigure 1 shows an ASM chart representing the operation of a controller. Stateassignments for each state are indicated in square brackets for [Q1, Q0].Using the ASM design technique:(a) Produce a State Transition Table from the ASM Chart in Figure 1.(b) Extract minimised Boolean expressions from your state transition tablefor Q1, Q0, DISPATCH and REJECT. Show all your working.(c) Implement your design using AND/OR/NOT logic gates and risingedgetriggered D-type Flip Flops. Your answer should include a circuitschematic.arrow_forwardA controller is required for a home security alarm, providing the followingfunctionality. The alarm does nothing while it is disarmed (‘switched off’). It canbe armed (‘switched on’) by entering a PIN on the keypad. Whenever thealarm is armed, it can be disarmed by entering the PIN on the keypad.If motion is detected while the alarm is armed, the siren should sound AND asingle SMS message sent to the police to notify them. Further motion shouldnot result in more messages being sent. If the siren is sounding, it can only bedisarmed by entering the PIN on the keypad. Once the alarm is disarmed, asingle SMS should be sent to the police to notify them.Two (active-high) input signals are provided to the controller:MOTION: Asserted while motion is detected inside the home.PIN: Asserted for a single clock cycle whenever the PIN has beencorrectly entered on the keypad.The controller must provide two (active-high) outputs:SIREN: The siren sounds while this output is asserted.POLICE: One SMS…arrow_forward
- 4G+ Vo) % 1.1. LTE1 : Q B NIS شوز طبي ۱:۱۷ کا A X حاز هذا على إعجاب Mohamed Bashar. MEDICAL SHOE شوز طبي ممول . اقوى عرض بالعراق بلاش سعر القطعة ١٥ الف سعر القطعتين ٢٥ الف سعر 3 قطع ٣٥ الف القياسات : 40-41-42-43-44- افحص وكدر ثم ادفع خدمة التوصيل 5 الف لكافة محافظات العراق ופרסם BNI SH ופרסם DON JU WORLD DON JU MORISO DON JU إرسال رسالة III Messenger التواصل مع شوز طبي تعليق باسم اواب حمیدarrow_forwardA manipulator is identified by the following table of parameters and variables:a. Obtain the transformation matrices between adjacent coordinate frames and calculate the global transformation matrix.arrow_forwardWhich tool takes the 2 provided input datasets and produces the following output dataset? Input 1: Record First Last Output: 1 Enzo Cordova Record 2 Maggie Freelund Input 2: Record Frist Last MI ? First 1 Enzo Last MI Cordova [Null] 2 Maggie Freelund [Null] 3 Jason Wayans T. 4 Ruby Landry [Null] 1 Jason Wayans T. 5 Devonn Unger [Null] 2 Ruby Landry [Null] 6 Bradley Freelund [Null] 3 Devonn Unger [Null] 4 Bradley Freelund [Null] OA. Append Fields O B. Union OC. Join OD. Find Replace Clear selectionarrow_forward
- Principles of Information Security (MindTap Cours...Computer ScienceISBN:9781337102063Author:Michael E. Whitman, Herbert J. MattordPublisher:Cengage LearningManagement Of Information SecurityComputer ScienceISBN:9781337405713Author:WHITMAN, Michael.Publisher:Cengage Learning,Principles of Information Systems (MindTap Course...Computer ScienceISBN:9781285867168Author:Ralph Stair, George ReynoldsPublisher:Cengage Learning
- Information Technology Project ManagementComputer ScienceISBN:9781337101356Author:Kathy SchwalbePublisher:Cengage LearningCOMPREHENSIVE MICROSOFT OFFICE 365 EXCEComputer ScienceISBN:9780357392676Author:FREUND, StevenPublisher:CENGAGE LPrinciples of Information Systems (MindTap Course...Computer ScienceISBN:9781305971776Author:Ralph Stair, George ReynoldsPublisher:Cengage Learning
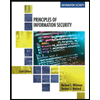
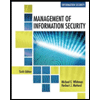
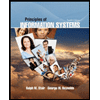
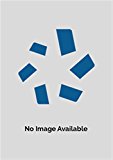
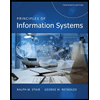