Concept explainers
The monthly sales for Yazici Batteries, Inc., were as follows:
a) Plot the monthly sales data.
b)
i) Naive method.
ii) A 3-month moving average.
iii) A 6-month weighted average using .1, .1, .1, .2, .2, and .3, with the heaviest weights applied to the most recent months.
iv) Exponential smoothing using an α = .3 and a September forecast of 18.
v) A trend projection.
c) With the data given, which method would allow you to forecast next March’s sales?
a)

To determine: Plot and represent the monthly sales data in graphical form.
Introduction: Forecasting is used to predict future changes or demand patterns. It involves different approaches and varies with different time periods. A sequence of data points in successive order is known as a time series. Time series forecasting is the prediction based on past events which are at a uniform time interval.
Answer to Problem 6P
The monthly sales data is plotted and represented.
Explanation of Solution
Given information:
Month | Sales |
January | 20 |
February | 21 |
March | 15 |
April | 14 |
May | 13 |
June | 16 |
July | 17 |
August | 18 |
September | 20 |
October | 20 |
November | 21 |
December | 23 |
Table 1
Graph:
The data to plot the sales is obtained from Table 1. Graph is plotted with the sales for January to December.
Thus, the sales data points are plotted and the graphical representation of sales data is presented.
b) i)

To determine: Forecast January sales using Naïve method.
Answer to Problem 6P
The forecast for January using Naïve method is 23
Explanation of Solution
Given information:
Month | Sales |
January | 20 |
February | 21 |
March | 15 |
April | 14 |
May | 13 |
June | 16 |
July | 17 |
August | 18 |
September | 20 |
October | 20 |
November | 21 |
December | 23 |
Naïve Approach: This method assumes that the demand for a particular period will be the same as the demand in the most recent period.
Month | Sales |
January | 20 |
February | 21 |
March | 15 |
April | 14 |
May | 13 |
June | 16 |
July | 17 |
August | 18 |
September | 20 |
October | 20 |
November | 21 |
December | 23 |
January | 23 |
According to the naïve approach, the demand for January will be the same as the demand in the most recent past month. That is, the demand will be the same as that of December. Therefore, the demand for January will be same as the demand of December; 23.
Hence, the forecast for January using naïve approach is 23
ii)

To determine: Forecast January sales using 3-month moving average.
Answer to Problem 6P
The forecast for January using 3-month moving average is 50.67
Explanation of Solution
Given information:
Month | Sales |
January | 20 |
February | 21 |
March | 15 |
April | 14 |
May | 13 |
June | 16 |
July | 17 |
August | 18 |
September | 20 |
October | 20 |
November | 21 |
December | 23 |
Formula to calculate the demand forecast:
Month | Sales | Moving Average |
January | 20 | |
February | 21 | |
March | 15 | |
April | 14 | 42.67 |
May | 13 | 36.00 |
June | 16 | 32.00 |
July | 17 | 33.67 |
August | 18 | 37.33 |
September | 20 | 40.33 |
October | 20 | 43.67 |
November | 21 | 46.00 |
December | 23 | 47.67 |
January | 50.67 |
Excel worksheet:
Calculation of the demand forecast for January sales:
Substitute the summation of the values 20, 21, and 23and divide it by the nth period; n=3
The January forecast is 50.67
Hence, the forecast of January sales using 3-month moving average is 50.67
iii)

To determine: Forecast January sales using 6-month weighted moving average.
Answer to Problem 6P
The forecast for January using 6-month moving average is 20.60
Explanation of Solution
Given information:
Month | Sales |
January | 20 |
February | 21 |
March | 15 |
April | 14 |
May | 13 |
June | 16 |
July | 17 |
August | 18 |
September | 20 |
October | 20 |
November | 21 |
December | 23 |
Formula to calculate the demand forecast:
Month | Sales | Weighted moving average |
January | 20 | |
February | 21 | |
March | 15 | |
April | 14 | |
May | 13 | |
June | 16 | |
July | 17 | 15.80 |
August | 18 | 15.90 |
September | 20 | 16.20 |
October | 20 | 17.30 |
November | 21 | 18.20 |
December | 23 | 19.40 |
January | 20.60 |
Excel worksheet:
Calculation for the demand forecast of January sales:
To calculate the forecast for January, multiply the weights with the sales of recent year, i.e. multiply weight 0.3 with 23, 0.2 with 21, 0.2 with 20, 0.1 with 20, 0.1 with 18 and 0.1 with 17.
Divide the summation of the multiplied values with the summation of the weights i.e. (0.3+0.2+0.2+0.1+0.1+0.1). The corresponding result is 20.60which is the forecasted value for January. Therefore January forecast is 20.60.
Hence, the forecast of January sales using 6-month weighted moving average is 20.60
iv)

To determine: Forecast January sales using exponential smoothing method.
Answer to Problem 6P
The forecast for January using exponential smoothing method is 20.6298
Explanation of Solution
Given information:
Month | Sales |
January | 20 |
February | 21 |
March | 15 |
April | 14 |
May | 13 |
June | 16 |
July | 17 |
August | 18 |
September | 20 |
October | 20 |
November | 21 |
December | 23 |
Formula to calculate the demand forecast
Where
Sl. No. | Month | Sales | Forecast |
1 | January | 20 | |
2 | February | 21 | |
3 | March | 15 | |
4 | April | 14 | |
5 | May | 13 | |
6 | June | 16 | |
7 | July | 17 | |
8 | August | 18 | |
9 | September | 20 | 18 |
10 | October | 20 | 18.6 |
11 | November | 21 | 19.02 |
12 | December | 23 | 19.614 |
13 | January | 20.6298 |
Excel worksheet:
Calculation of the forecast for October:
To calculate forecast for October, substitute the value of forecast of September, smoothing constant and difference of actual and forecasted demand of September. The result of forecast for October is 18.6.
Calculation of the forecast for November:
To calculate forecast for November, substitute the value of forecast of October, smoothing constant and difference of actual and forecasted demand of October. The result of forecast for November is 19.02.
Calculation of the forecast for December:
To calculate forecast for December, substitute the value of forecast of November, smoothing constant and difference of actual and forecasted demand of November. Therefore, the forecast for December is 19.614.
Calculation of the forecast for January:
To calculate forecast for January, substitute the value of forecast of December, smoothing constant and difference of actual and forecasted demand of December. Therefore, the forecast for January is 20.6298.
Hence, the forecast of January sales using exponential smoothing method is 20.6298
v)

To determine: Forecast January sales using trend projection.
Answer to Problem 6P
The forecast for January using trend projection is 20.754
Explanation of Solution
Given information:
Month | Sales |
January | 20 |
February | 21 |
March | 15 |
April | 14 |
May | 13 |
June | 16 |
July | 17 |
August | 18 |
September | 20 |
October | 20 |
November | 21 |
December | 23 |
Formula to calculate the demand forecast
Where,
Where
Month (x) | Sales (y) | xy | x2 |
1 | 20 | 20 | 1 |
2 | 21 | 42 | 4 |
3 | 15 | 45 | 9 |
4 | 14 | 56 | 16 |
5 | 13 | 65 | 25 |
6 | 16 | 96 | 36 |
7 | 17 | 119 | 49 |
8 | 18 | 144 | 64 |
9 | 20 | 180 | 81 |
10 | 20 | 200 | 100 |
11 | 21 | 231 | 121 |
12 | 23 | 276 | 144 |
∑=78 | ∑=218 | ∑=1474 | ∑=650 |
Substituting the values in the above formula
Calculation of average of x values
Average of x values is obtained by dividing the summation of x values i.e. (1+2+…+12) with the number of period n i.e.12. The value of
Calculation of average of y values
Average of y values is obtained by dividing the summation of sales with the number of period n i.e.12. The value of
Calculation of slope of regression line ‘b’:
Summation of product of sales (y) with x values is ∑xy = 1474, product of number of months (n), average of x values and average of y values is obtained i.e.
Summation of square of x values i.e. 650 is subtracted from the product of number of months i.e. 12 with average of x values i.e. 6.5. The resultant value is 143. The slope of regression line is obtained by dividing 57 with 143. The value of ‘b’ is 0.398.
Calculation of y axis intercept ‘a’:
The y axis intercept is obtained by the difference between average of y values and values obtained by the product of slope of regression line with average of x values. The resultant value of ‘a’ is 15.579.
Calculation of forecast of January:
The January forecast is obtained by summation of the product of slope of regression line and forecasted month, January i.e. 13 with the y-axis intercept. The forecasted value obtained is 20.754.
Hence, the forecast for January sales using trend projection is 20.754
c)

To determine: The best technique among time series methods to forecast March sales.
Explanation of Solution
The calculated results from the data revels that the trend projection (refer to equation (4)) is the best suitable technique to forecast March sales as it is useful in evaluating trends in the data.
Want to see more full solutions like this?
Chapter 4 Solutions
PRIN.OF OPERATIONS MANAGEMENT-MYOMLAB
- The Baker Company wants to develop a budget to predict how overhead costs vary with activity levels. Management is trying to decide whether direct labor hours (DLH) or units produced is the better measure of activity for the firm. Monthly data for the preceding 24 months appear in the file P13_40.xlsx. Use regression analysis to determine which measure, DLH or Units (or both), should be used for the budget. How would the regression equation be used to obtain the budget for the firms overhead costs?arrow_forwardThe file P13_42.xlsx contains monthly data on consumer revolving credit (in millions of dollars) through credit unions. a. Use these data to forecast consumer revolving credit through credit unions for the next 12 months. Do it in two ways. First, fit an exponential trend to the series. Second, use Holts method with optimized smoothing constants. b. Which of these two methods appears to provide the best forecasts? Answer by comparing their MAPE values.arrow_forwardThe owner of a restaurant in Bloomington, Indiana, has recorded sales data for the past 19 years. He has also recorded data on potentially relevant variables. The data are listed in the file P13_17.xlsx. a. Estimate a simple regression equation involving annual sales (the dependent variable) and the size of the population residing within 10 miles of the restaurant (the explanatory variable). Interpret R-square for this regression. b. Add another explanatory variableannual advertising expendituresto the regression equation in part a. Estimate and interpret this expanded equation. How does the R-square value for this multiple regression equation compare to that of the simple regression equation estimated in part a? Explain any difference between the two R-square values. How can you use the adjusted R-squares for a comparison of the two equations? c. Add one more explanatory variable to the multiple regression equation estimated in part b. In particular, estimate and interpret the coefficients of a multiple regression equation that includes the previous years advertising expenditure. How does the inclusion of this third explanatory variable affect the R-square, compared to the corresponding values for the equation of part b? Explain any changes in this value. What does the adjusted R-square for the new equation tell you?arrow_forward
- The file P13_22.xlsx contains total monthly U.S. retail sales data. While holding out the final six months of observations for validation purposes, use the method of moving averages with a carefully chosen span to forecast U.S. retail sales in the next year. Comment on the performance of your model. What makes this time series more challenging to forecast?arrow_forwardThe file P13_26.xlsx contains the monthly number of airline tickets sold by the CareFree Travel Agency. a. Create a time series chart of the data. Based on what you see, which of the exponential smoothing models do you think will provide the best forecasting model? Why? b. Use simple exponential smoothing to forecast these data, using a smoothing constant of 0.1. c. Repeat part b, but search for the smoothing constant that makes RMSE as small as possible. Does it make much of an improvement over the model in part b?arrow_forwardThe file P13_29.xlsx contains monthly time series data for total U.S. retail sales of building materials (which includes retail sales of building materials, hardware and garden supply stores, and mobile home dealers). a. Is seasonality present in these data? If so, characterize the seasonality pattern. b. Use Winters method to forecast this series with smoothing constants = = 0.1 and = 0.3. Does the forecast series seem to track the seasonal pattern well? What are your forecasts for the next 12 months?arrow_forward
- The file P13_02.xlsx contains five years of monthly data on sales (number of units sold) for a particular company. The company suspects that except for random noise, its sales are growing by a constant percentage each month and will continue to do so for at least the near future. a. Explain briefly whether the plot of the series visually supports the companys suspicion. b. By what percentage are sales increasing each month? c. What is the MAPE for the forecast model in part b? In words, what does it measure? Considering its magnitude, does the model seem to be doing a good job? d. In words, how does the model make forecasts for future months? Specifically, given the forecast value for the last month in the data set, what simple arithmetic could you use to obtain forecasts for the next few months?arrow_forwardThe file P13_28.xlsx contains monthly retail sales of U.S. liquor stores. a. Is seasonality present in these data? If so, characterize the seasonality pattern. b. Use Winters method to forecast this series with smoothing constants = = 0.1 and = 0.3. Does the forecast series seem to track the seasonal pattern well? What are your forecasts for the next 12 months?arrow_forwardDo the sales prices of houses in a given community vary systematically with their sizes (as measured in square feet)? Answer this question by estimating a simple regression equation where the sales price of the house is the dependent variable, and the size of the house is the explanatory variable. Use the sample data given in P13_06.xlsx. Interpret your estimated equation, the associated R-square value, and the associated standard error of estimate.arrow_forward
- a) Using the trend-projection (regression) method, develop a forecastfor the sales of Volkswagen Beetles in Nevada through year 6.b) What is the MAD?~c) What is the MSE?arrow_forward4.4 A check-processing center uses exponential smooth- ing to forecast the number of incoming checks each month. The number of checks received in June was 40 million, while the fore- cast was 42 million. A smoothing constant of .2 is used. a) What is the forecast for July? b) If the center received 45 million checks in July, what would be the forecast for August? c) Why might this be an inappropriate forecasting method for this situation? Px .. 4.5 The Carbondale Hospital is considering the purchase of a new ambulance. The decision will rest partly on the antici- pated mileage to be driven next year. The miles driven during the past 5 years are as follows: YEAR 1 2 3 4 5 a) Forecast the mileage for next year (6th year) using a 2-year moving average. b) Find the MAD based on the 2-year moving average. (Hint: You will have only 3 years of matched data.) c) Use a weighted 2-year moving average with weights of 4 and .6 to forecast next year's mileage. (The weight of .6 is for the most…arrow_forwardThe monthly sales for Telco Batteries, Inc. are as follows (see image): Plot the monthly sales data Forecast January sales using each of the following: Naïve method A 3-month moving average A 6-month weighted average using 0.1, 0.1, 0.1, 0.2, 0.2, and 0.3 with the heaviest weights applied to the most recent month Exponential smoothing using an alpha = 0.3 and a September forecast of 18 A trend projection With the data given, which method would allow you to forecast next March’s sales?arrow_forward
- Practical Management ScienceOperations ManagementISBN:9781337406659Author:WINSTON, Wayne L.Publisher:Cengage,Contemporary MarketingMarketingISBN:9780357033777Author:Louis E. Boone, David L. KurtzPublisher:Cengage LearningMarketingMarketingISBN:9780357033791Author:Pride, William MPublisher:South Western Educational Publishing
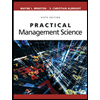
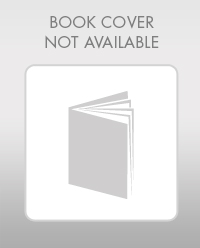