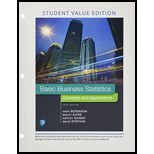
The data stored in McDonalds represent the gross revenues (in billions of current dollars) McDonald’s Corporation from 1975 through 2016:
a. Plot the data.
b. Compute the linear trend forecasting equation.
c. Compute the quadratic trend forecasting equation.
d. Compute the exponential trend forecasting equation.
e. Determine the best-fitting autoregressive model, using
f. Perform a residual analysis for each of the models in (b) through (e).
g. Compute the standard error of the estimate
h. On the basis of your results in (f) and (g), along with a consideration of the principle of parsimony, which model would you select for purpose of forecasting? Discuss.
i. Using the selected model in (h), forecast gross revenues for 2017.

Want to see the full answer?
Check out a sample textbook solution
Chapter 16 Solutions
Basic Business Statistics, Student Value Edition
- Table 2 shows a recent graduate’s credit card balance each month after graduation. a. Use exponential regression to fit a model to these data. b. If spending continues at this rate, what will the graduate’s credit card debt be one year after graduating?arrow_forwardConsider the following estimated trend models. Use them to make a forecast for t= 22. a. Linear Trend: ý = 12.42 + 1.13t (Round intermediate calculations to at least 4 decimal places and final answer to 2 decimal places.) b. Quadratic Trend: û = 18.04 + 1.04t - 0.02t2 (Round intermediate calculations to at least 4 decimal places and final answer to 2 decimal places.) c. Exponential Trend: In(y) = 1.6 + 0.08t, se = 0.01 (Round intermediate calculations to at least 4 decimal places and final answer to 2 decimal places.)arrow_forwardThe following chart shows the actual sales for the last 12 months for a given company. Assume that sales are best fit by a linear trend and you can use single linear regression to set up a forecasting model. Using the sales data answer below questions (justify your answers): A.What would be the typical linear regression equation for the number of sales? B.Make the sales forecast for period 15 of next year. C. Make the sales forecast for period 17 of next year. D. What is the standard error for the data?arrow_forward
- Part c: What percentage of an adult size brain does a child have at age 10? Part d: Determine whether the value from the model is larger or smaller than the value in the table.arrow_forwardplease answer in excel Greenapple Services Inc. calls previous customers to introduce and sell their new products every months.The following data have been collected for the past year showing sales and number of calls on the same month: a) Develop a regression equation to forecast the Sales as a function of the calls. b) Suppose that the company would like to place 32 calls this month. Estimate the Sales in response to this amount of calls.arrow_forwardThe percentage of mothers who smoke cigarettes during pregnancy has declined approximately linearly from13.1% in 1997 to 12.3% in 2004 Predict the percentage in 2015.arrow_forward
- Ozone (O3) is a major component of air pollution in many cities. Atmospheric ozone levels are influenced by many factors, including weather. In one study, the mean percent relative humidity (x) and the mean ozone levels (y) were measured for 120 days in a western city. Mean ozone levels were measured in ppb. The following output (from MINITAB) describes the fit of a linear model to these data. Assume that assumptions 1 through 4 on page 549 hold. The regression equation is Ozone = 88.8 - 0.752 Humidity Predictor Coef SE Coef тР Constant 88.761 7.288 12.18 0.000 Humidity -0.7524 0.13024 -5.78 0.000 S= 11.43R-Sq = 22.0°%R-Sq(adj) = 21.4% %3D Predicted Values for New Observations New Obs FitSE Fit 95.0% CI 95.0% PI 43.62 1.20(41.23 46.00) (20.86, 66.37) Values of Predictors for New Observations New Obs Humidity 60.0 What are the slope and intercept of the least-squares line? Is the linear model useful for predicting ozone levels from relative humidity? Explain. c. Predict the ozone level…arrow_forward1 2 3 4 5 6 7 8 9 10 11 12 13 14 15 16 17 18 19 20 21 22 23 24 25 26 27 28 29 Price/Book Value Ratio Return on Equity 13.032 1.405 8.305 2.113 6.654 1.239 3.262 2.449 5.291 2.398 7.719 0.353 2.569 7.593 5.104 2.012 4.797 2.182 4.129 1.918 1.549 1.951 5.046 2.417 2.159 3.011 1.725 5.582 4.698 Growth% 6.385 11.846 135.669 12.459 0.073 25.092 14.188 8.804 22.766 38.082 18.985 25.696 24.519 19.666 11.624 22.849 49.965 69.649 36.696 3.819 41.139 9.218 29.108 17.772 25.114 29.295 23.764 31.405 9.497 14.759 18.541 12.026 39.016 14.228 39.439 14.097 27.022 14.841 13.237 20.669 17.311 14.887 15.849 5.601 16.775 11.172 8.401 16.161 18.404 23.973 16.673 14.725 46.605 28.839 52.021arrow_forwardIn the area of Russia récords were kept on the relationship between the rain Fall in inches and the yield of wheat (bushels per acre ).the data for a 9 year period is as follow. Rain fall x 13.1,11.4,16.0,15.1,21.4,12.9,9.6,18.2,18.6. yield y : 48.5,44.2,56.8,80.4,47.2,29.9,74.0,74.0,76.8 the equation of the line of least squares is given as ^y= -9.12+4.38x. How many bushels of wheat peen acre can be predicted if it’s is expected that there will be 30 inches of rain?arrow_forward
- The following data of regular growth (linear regression) sales for XYZ company during last eleven years (2005 to 2015): Student Name Student ID cW16 Years Sales 2005 5500 2006 6500 2007 7800 2008 9000 2009 11500 2010 10600 2011 10000 2012 8600 2013 10200 2014 10500 2015 10900 2016 2017 2018 2019 2020 eee Required: 1. Create a new worksheet "Q3", and enter the previous data in it. 2. Use the (FORECAST) functions to predict the expected sales for the following five years (2016 to 2020) "predict expected sales based on actual sales). Actual Sales Expected Salesarrow_forwardAssume that the current date is February 1, 2021. The linear regression model was applied to a monthly time series data based on the last 24 months' sales. (From January 2019 through December 2020). The following partial computer output summarizes the results. Coefficient Estimate t Intercept 4.3 2.07 Slope 1.6 2.98 Determine the predicted sales for February.arrow_forwardAlthough natural gas is currently inexpensive and nuclear power currently (and perhaps deservedly) does not have a good reputation, it is possible that more nuclear power plants will be constructed in the future. Table 4 presents data concerning the construction costs of light water reactor (LWR) nuclear power plants. The dependent variable, C, construction cost, is expressed in millions of dollars, adjusted to a 1976 base. Preliminary analysis of the data and economic theory indicate that variation in cost increases as cost increases. This suggests transforming cost by taking its natural logarithm. S Power plant capacity in MWe N Cumulative number of power plants built by the contractor Build a multiple regression model to predict ln(C) by taking S and N or their natural logarithms as the independent variables. Make sure to check for multicollinearity. Use residual analysis and R2 to check your model. State which variables are important in predicting the cost of constructing an…arrow_forward
- Algebra & Trigonometry with Analytic GeometryAlgebraISBN:9781133382119Author:SwokowskiPublisher:CengageGlencoe Algebra 1, Student Edition, 9780079039897...AlgebraISBN:9780079039897Author:CarterPublisher:McGraw Hill
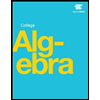
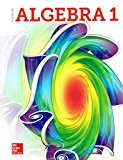