Concept explainers
Utilization of sucrose as a carbon source for the production of chemicals is uneconomical. Beet molasses is a readily available and low-priced substitute. The article “Optimization of the Production of b-Carotene from Molasses by Blakeslea Trispora” β. of Chem. Tech. and Biotech., 2002: 933–943) carried out a multiple
Obs | Linoleic | Kerosene | Antiox | Betacaro |
1 | 30.00 | 30.00 | 10.00 | 0.7000 |
2 | 30.00 | 30.00 | 10.00 | 0.6300 |
3 | 30.00 | 30.00 | 18.41 | 0.0130 |
4 | 40.00 | 40.00 | 5.00 | 0.0490 |
5 | 30.00 | 30.00 | 10.00 | 0.7000 |
6 | 13.18 | 30.00 | 10.00 | 0.1000 |
7 | 20.00 | 40.00 | 5.00 | 0.0400 |
8 | 20.00 | 40.00 | 15.00 | 0.0065 |
9 | 40.00 | 20.00 | 5.00 | 0.2020 |
10 | 30.00 | 30.00 | 10.00 | 0.6300 |
11 | 30.00 | 30.00 | 1.59 | 0.0400 |
12 | 40.00 | 20.00 | 15.00 | 0.1320 |
13 | 40.00 | 40.00 | 15.00 | 0.1500 |
14 | 30.00 | 30.00 | 10.00 | 0.7000 |
15 | 30.00 | 46.82 | 10.00 | 0.3460 |
16 | 30.00 | 30.00 | 10.00 | 0.6300 |
17 | 30.00 | 13.18 | 10.00 | 0.3970 |
18 | 20.00 | 20.00 | 5.00 | 0.2690 |
19 | 20.00 | 20.00 | 15.00 | 0.0054 |
20 | 46.82 | 30.00 | 10.00 | 0.0640 |
a. Fitting the complete second-order model in the three predictors resulted in R2 = .987 and adjusted R2 = .974, whereas fitting the first-order model gave R2 = .016. What would you conclude about the two models?
b. For x1 = x2 = 30, x3 = 10, a statistical software package reported that

Trending nowThis is a popular solution!

Chapter 13 Solutions
PROBABILITY & STATS FOR ENGINEERING &SCI
- Which of the following is(are) TRUE if the coefficient of determination between the response and the predictor variables is 0.81 based on a random sample of size n. O B. The Pearson's sample correlation coefficient is +0.9. O A. This means that 19% of the total variation in the response variable remains unexplained by the simple linear regression model that uses the given predictor variable. O Both A and B Neither A nor Barrow_forwardIn an attempt to develop a model of wine quality as judged by wine experts, data was collected from 50 red wine variants of a certain type of wine. A multiple linear regression model to predict wine quality, measured on a scale from 0 (very bad) to 10 (excellent) was developed, based on alcohol content (%), X1, and the amount of chlorides, X2. Perform a multiple regression analysis and determine the VIF for each independent variable in the model. Is there reason to suspect the existence of collinearity? Determine the VIF for each independent variable in the model, with VIF1 and VIF2 being the VIF for X1 and X2, respectively.arrow_forwardHormone replacement therapy (HRT) is thought to increase the risk of breast cancer. The accompanying data on x = percent of women using HRT and y = breast cancer incidence (cases per 100,000 women) for a region in Germany for 5 years appeared in the paper "Decline in Breast Cancer Incidence after Decrease in Utilization of Hormone Replacement Therapy." The authors of the paper used a simple linear regression model to describe the relationship between HRT use and breast cancer incidence. t HRT Use Breast Cancer Incidence 46.30 40.60 39.50 36.60 30.00 103.30 105.00 100.00 93.80 83.50 (a) What is the equation of the estimated regression line? (Round your numerical values to four decimal places.) ŷ = (b) What is the estimated average change in breast cancer incidence (in cases per 100,000 women) associated with a 1 percentage point increase in HRT use? (Round your answer to four decimal places.) cases per 100,000 women (c) What breast cancer incidence (in cases per 100,000 women) would be…arrow_forward
- Consider the model Ci= B0+B1 Yi+ ui. Suppose you run this regression using OLS and get the following results: b0=-3.13437; SE(b0)=0.959254; b1=1.46693; SE(b1)=0.0697828; R-squared=0.130357; and SER=8.769363. Note that b0 and b1 the OLS estimate of b0 and b1, respectively. The total number of observations is 2950. The number of degrees of freedom for this regression is A. 2950 OB. 2948 OC. 2952 OD. 2arrow_forwardOne is interested in the ceteris paribus relationship between the dependent variable y, and the explanatory variable ₁1. For this, one collects data on two control variables Xi2 and Xiz and runs two LS regressions. Regression 1: yi = B₁x₁1 + Ui Regression 2: y = B₁xil+ B₂x₁2 + B3x13 + Ei Let ₁ denote the LS estimate of ₁ of Regression 1 and let ₁ denote the LS estimate of ₁ in Regression 2. Assume that the true model is given by Regression 2 and that all variables are centered. a) Would you expect a difference between ₁ and ₁ when is highly correlated with 2 and 3 and the partial effects of xi2 and xi3 on yi are also high? b) Would you expect a difference between ₁ and 3₁ when ₁ has almost no correlation with and Xi3 but X2 and 3 are highly correlated? Xi2 c) Which of the two estimators is more efficient if x₁1 is highly correlated with 2 and 13, and Xiz have small partial effects on yi? but i2 d) Which of the two estimators is more efficient if x₁1 is almost uncorrelated with 2 and…arrow_forwardHormone replacement therapy (HRT) is thought to increase the risk of breast cancer. The accompanying data on x = percent of women using HRT and y = breast cancer incidence (cases per 100,000 women) for a region in Germany for 5 years appeared in the paper "Decline in Breast Cancer Incidence after Decrease in Utilization of Hormone Replacement Therapy." The authors of the paper used a simple linear regression model to describe the relationship between HRT use and breast cancer incidence. HRT Use Breast Cancer Incidence 46.30 103.30 40.60 105.00 39.50 100.00 36.60 93.80 30.00 83.50 n USE SALT (a) What is the equation of the estimated regression line? (Round your numerical values to four decimal places.) ý = (b) What is the estimated average change in breast cancer incidence (in cases per 100,000 women) associated with a 1 percentage point increase in HRT use? (Round your answer to four decimal places.) cases per 100,000 women (c) What breast cancer incidence (in cases per 100,000 women)…arrow_forward
- Hormone replacement therapy (HRT) is thought to increase the risk of breast cancer. The accompanying data on x = percent of women using HRT and y = breast cancer incidence (cases per 100,000 women) for a region in Germany for 5 years appeared in the paper "Decline in Breast Cancer Incidence after Decrease in Utilization of Hormone Replacement Therapy." The authors of the paper used a simple linear regression model to describe the relationship between HRT use and breast cancer incidence. HRT Use Breast Cancer Incidence 46.30 103.30 40.60 105.00 39.50 100.00 36.60 93.80 30.00 83.50 n USE SALT (a) What is the equation of the estimated regression line? (Round your numerical values to four decimal places.) ý = 45.5727 + (1.3354 )x (b) What is the estimated average change in breast cancer incidence (in cases per 100,000 women) associated with a 1 percentage point increase in HRT use? (Round your answer to four decimal places.) 1.3354 cases per 100,000 women (c) What breast cancer incidence…arrow_forwardA baseball enthusiast carried out a simple linear regression to investigate whether there is a linear relationship between the number of runs scored by a player and the number of times the player was intentionally walked. Computer output from the regression analysis is shown. Variable DF Estimate SE Intercept 1 16 2.073 Intentional Walks 1 0.50 0.037 R-sq=0.63R-sq=0.63 Let β1β1 represent the slope of the population regression line used to predict the number of runs scored from the number of intentional walks in the population of baseball players. A tt-test for a slope of a regression line was conducted for the following hypotheses. H0:β1=0Ha:β1≠0 below are the options provided thank youarrow_forward3. Wine Participant magazine has collected average price per bottle for the prestigious Chateau Le Thundebird bordeaux for different vintages (years). The data appears in the table below. year of bottling price a) draw the scatter diagram showing how wine price varies by vintage year b) use the most appropriate regression equation to determine the relationship between year of bottling (age) and price. c) what is the explanatory power (RSQ) of that equation d) determine the predicted price of a bottle of this wine for the 2017 vintage. 2009 36 2010 40 2011 51 2012 60 2013 68 2014 72 2015 70 2016 65 2018 51 2019 44 2020 39arrow_forward
- An article described an experiment carried out to assess the impact of the variable x₁ = force (gm), x₂ = power (mW), x3 = temperature (°C), and time (msec) on y ball bond shear strength (gm). X4 = = The estimated regression equation is y = -37.48 +0.2117x₁ +0.4983x2 +0.1297x3 +0.2583x4 Interpret b3 and b4. ● What is the expected value of strength (y) from a force of 35 gm, power of 75 mW, temperature of 200 °C, and time of 20 msec ?arrow_forwardSnowpacks contain a wide spectrum of pollutants thatmay represent environmental hazards. The article“Atmospheric PAH Deposition: Deposition Velocitiesand Washout Ratios” (J. of EnvironmentalEngineering, 2002: 186–195) focused on the depositionof polyaromatic hydrocarbons. The authors proposeda multiple regression model for relating depositionover a specified time period (y, in mg/m2) to tworather complicated predictors x1 (mg-sec/m3) and x2 (mg/m2), defined in terms of PAH air concentrations forvarious species, total time, and total amount of precipitation.Here is data on the species fluoranthene andcorresponding Minitab output:obs x1 x2 flth1 92017 .0026900 278.782 51830 .0030000 124.533 17236 .0000196 22.654 15776 .0000360 28.685 33462 .0004960 32.666 243500 .0038900 604.707 67793 .0011200 27.698 23471 .0006400 14.189 13948 .0004850 20.6410 8824 .0003660 20.6011 7699 .0002290 16.6112 15791 .0014100 15.0813 10239 .0004100 18.0514 43835 .0000960 99.7115 49793 .0000896 58.9716 40656…arrow_forwardAn oil exploration company wants to develop a statistical model to predict the cost of drilling a new well. One of the many variables thought to be an important predictor of the cost is the number of feet in depth that the must be drilled to create the well. Consequently, the company decided to fit the simple linear regression model, where y = cost of drilling the new well (in $thousands) and x = number of feet drilled to create the well. Using data collected for a sample of n=83 wells, the following results were obtained: = 10.5 + 16.20x Give a practical interpretation of the estimate of the slope of the least squares line. An oil exploration company wants to develop a statistical model to predict the cost of drilling a new well. One of the many variables thought to be an important predictor of the cost is the number of feet in depth that the must be drilled to create the well. Consequently, the company decided to fit the simple linear regression model, where y =…arrow_forward
- Big Ideas Math A Bridge To Success Algebra 1: Stu...AlgebraISBN:9781680331141Author:HOUGHTON MIFFLIN HARCOURTPublisher:Houghton Mifflin Harcourt
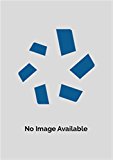