You are a researcher in the automobile industry studying fuel efficiency differences between vintage cars. You are interested in the following research question: “Did fuel efficiency among vintage cars vary depending on vehicle weight?” use the screen shot below to make reference to at least the following statistics in your write-up: the slope coefficient, the test t-statistic and p-value associated with the slope coefficient, the yintercept, and R
You are a researcher in the automobile industry studying fuel efficiency differences between vintage cars. You are interested in the following research question: “Did fuel efficiency among vintage cars vary depending on vehicle weight?” use the screen shot below to make reference to at least the following statistics in your write-up: the slope coefficient, the test t-statistic and p-value associated with the slope coefficient, the yintercept, and R
MATLAB: An Introduction with Applications
6th Edition
ISBN:9781119256830
Author:Amos Gilat
Publisher:Amos Gilat
Chapter1: Starting With Matlab
Section: Chapter Questions
Problem 1P
Related questions
Topic Video
Question
You are a researcher in the automobile industry studying fuel efficiency differences between
vintage cars. You are interested in the following research question: “Did fuel efficiency
among vintage cars vary depending on vehicle weight?”
use the screen shot below to make reference to at least the following statistics in your write-up: the slope
coefficient, the test t-statistic and p-value associated with the slope coefficient, the yintercept, and R

Transcribed Image Text:**Linear Regression Analysis Summary**
**Call:**
`lm(formula = mpg ~ weight, data = auto)`
This analysis involves a linear regression where the dependent variable is miles per gallon (mpg) and the independent variable is weight. The dataset used is named "auto."
**Residuals:**
- Min: -6.9593
- 1Q (First Quartile): -1.9325
- Median: -0.3713
- 3Q (Third Quartile): 0.8885
- Max: 13.8174
The residuals indicate the difference between the observed and predicted values of mpg. They provide an insight into the distribution of errors in the model.
**Coefficients:**
- **Intercept:**
- Estimate: 39.4402835
- Standard Error: 1.6140031
- t value: 24.44
- Pr(>|t|): <2e-16 ***
The intercept is the predicted value of mpg when the weight is zero.
- **Weight:**
- Estimate: -0.0060087
- Standard Error: 0.0005179
- t value: -11.60
- Pr(>|t|): <2e-16 ***
The weight coefficient indicates that for each unit increase in weight, the mpg decreases by approximately 0.006. The significance codes (*** for p-value < 0.001) suggest this variable is highly significant.
**Significance Codes:**
- 0 ‘***’
- 0.001 ‘**’
- 0.01 ‘*’
- 0.05 ‘.’
- 0.1 ‘ ’
- 1
**Model Summary:**
- Residual Standard Error: 3.439 on 72 degrees of freedom
- Multiple R-squared: 0.6515
- Adjusted R-squared: 0.6467
- F-statistic: 134.6 on 1 and 72 DF
- p-value: < 2.2e-16
The R-squared value indicates that approximately 65.15% of the variability in mpg can be explained by the weight. The high F-statistic and corresponding low p-value further confirm the model's overall significance.
This summary provides insights into how well weight predicts mpg and the
Expert Solution

This question has been solved!
Explore an expertly crafted, step-by-step solution for a thorough understanding of key concepts.
This is a popular solution!
Trending now
This is a popular solution!
Step by step
Solved in 2 steps

Knowledge Booster
Learn more about
Need a deep-dive on the concept behind this application? Look no further. Learn more about this topic, statistics and related others by exploring similar questions and additional content below.Recommended textbooks for you
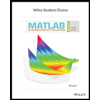
MATLAB: An Introduction with Applications
Statistics
ISBN:
9781119256830
Author:
Amos Gilat
Publisher:
John Wiley & Sons Inc
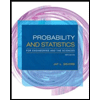
Probability and Statistics for Engineering and th…
Statistics
ISBN:
9781305251809
Author:
Jay L. Devore
Publisher:
Cengage Learning
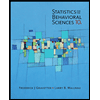
Statistics for The Behavioral Sciences (MindTap C…
Statistics
ISBN:
9781305504912
Author:
Frederick J Gravetter, Larry B. Wallnau
Publisher:
Cengage Learning
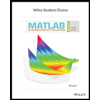
MATLAB: An Introduction with Applications
Statistics
ISBN:
9781119256830
Author:
Amos Gilat
Publisher:
John Wiley & Sons Inc
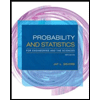
Probability and Statistics for Engineering and th…
Statistics
ISBN:
9781305251809
Author:
Jay L. Devore
Publisher:
Cengage Learning
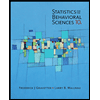
Statistics for The Behavioral Sciences (MindTap C…
Statistics
ISBN:
9781305504912
Author:
Frederick J Gravetter, Larry B. Wallnau
Publisher:
Cengage Learning
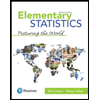
Elementary Statistics: Picturing the World (7th E…
Statistics
ISBN:
9780134683416
Author:
Ron Larson, Betsy Farber
Publisher:
PEARSON
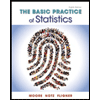
The Basic Practice of Statistics
Statistics
ISBN:
9781319042578
Author:
David S. Moore, William I. Notz, Michael A. Fligner
Publisher:
W. H. Freeman
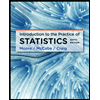
Introduction to the Practice of Statistics
Statistics
ISBN:
9781319013387
Author:
David S. Moore, George P. McCabe, Bruce A. Craig
Publisher:
W. H. Freeman