Write code to define a K-NN regression model with K=5 and the neighbors are weighted by the inverse of their distance.
![Write code to define a K-NN regression model with K=5 and the neighbors are weighted by the inverse
of their distance.
[ ] # Write your code here](/v2/_next/image?url=https%3A%2F%2Fcontent.bartleby.com%2Fqna-images%2Fquestion%2F9b63d5f0-a313-4df7-92fd-2acb696a8a17%2Ff6244dd8-274e-4aa0-b0da-860ddc639c9f%2Frm5mev7_processed.png&w=3840&q=75)

Algorithm: K-NN Regression
Input:
- Training data (X_train, y_train): Features and corresponding target values.
- New data point (X_new): The data point for which we want to make a prediction.
- Number of neighbors (K): The number of nearest neighbors to consider.
- Weighting scheme (weights): A choice of 'distance' to weight neighbors by inverse distance.
Output:
- Predicted target value for the new data point.
Steps:
1. Compute the Euclidean distance between the new data point (X_new) and all data points in X_train.
2. Sort the distances and identify the K nearest neighbors.
3. If using 'distance' weighting, calculate the weight for each neighbor as the inverse of their distance.
4. Compute the weighted average of the target values of the K nearest neighbors.
5. Return the weighted average as the predicted target value for the new data point.
Pseudocode:
1. Initialize an empty list 'distances' to store distances between X_new and each point in X_train.
2. For each data point (X_train_i, y_train_i) in X_train and y_train:
a. Calculate the Euclidean distance dist = ||X_new - X_train_i||.
b. Append dist to the 'distances' list.
3. Sort the 'distances' list and keep track of the indices of the K smallest distances.
4. Initialize a variable 'weighted_sum' to 0 and a variable 'total_weight' to 0.
5. For each index 'i' in the K nearest neighbor indices:
a. If 'weights' is 'distance', calculate the weight as weight = 1 / distances[i].
b. Add y_train[i] * weight to 'weighted_sum'.
c. Add weight to 'total_weight'.
6. Calculate the predicted target value as predicted_value = weighted_sum / total_weight.
7. Return predicted_value as the final prediction.
Note:
- This algorithm assumes a regression task where the target values are continuous.
- You can replace 'X_new' with your specific data point for prediction and 'X_train' and 'y_train' with your training data.
- The choice of the number of neighbors (K) and weighting scheme (weights) can be adjusted based on your problem and preferences.
Step by step
Solved in 3 steps with 2 images

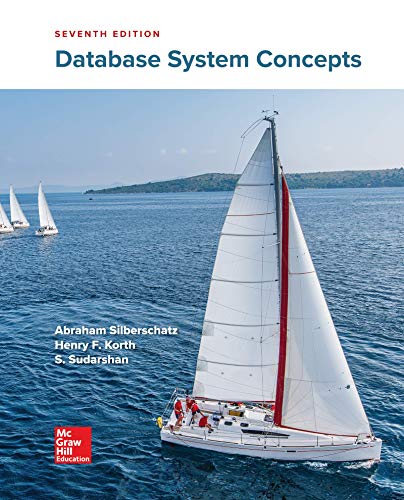
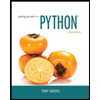
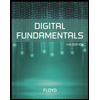
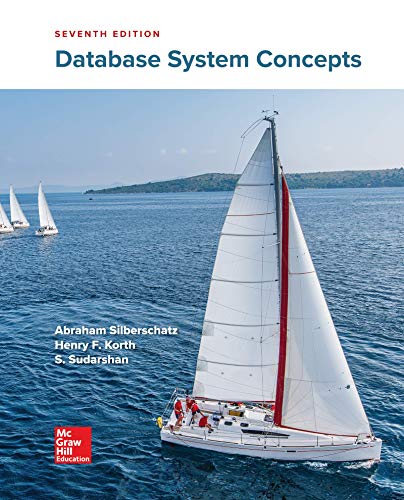
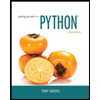
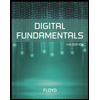
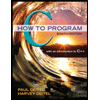
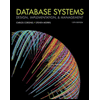
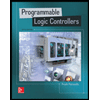