Now that we have fit our model, which means that we have computed the optimal model parameters, we can use our model to plot the regression line for the data. Below, I supply you with x_fit and y_fit that represent the x- and y-data of the regression line, respectively. All we need to do next is ask the model to predict a z_fit value for each x_fit and y_fit pair by invoking the model's predict() method. This should make sense when you consider the ordinary least squares linear regression equation for calculating z_fit: ????=?̂ 0+?̂ 1????+?̂ 2????zfit=θ^0+θ^1xfit+θ^2yfit
Create Second Image
Now that we have fit our model, which means that we have computed the optimal model parameters, we can use our model to plot the regression line for the data. Below, I supply you with x_fit and y_fit that represent the x- and y-data of the regression line, respectively. All we need to do next is ask the model to predict a z_fit value for each x_fit and y_fit pair by invoking the model's predict() method. This should make sense when you consider the ordinary least squares linear regression equation for calculating z_fit:
where ?̂ ?θ^i are the computed model parameters. You must use x_fit and y_fit as features to be passed together as a DataFrame to the model's predict() method, which will return z_fit as determined by the above equation. Once you obtain z_fit, you are ready to plot the regression line by plotting it against x_fit and y_fit.
Any dataset would be great. I just want to understand it.

Trending now
This is a popular solution!
Step by step
Solved in 2 steps with 2 images

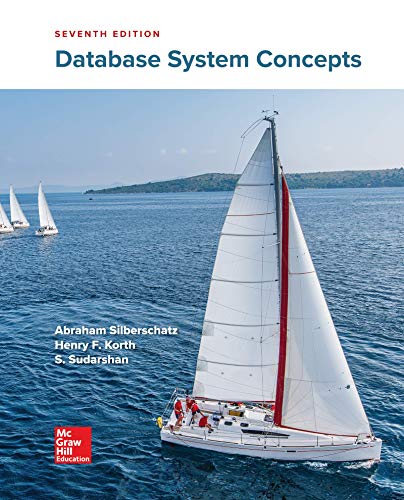
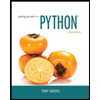
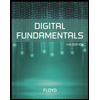
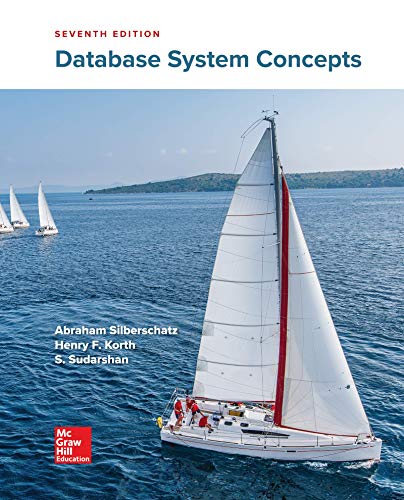
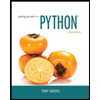
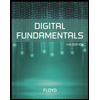
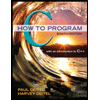
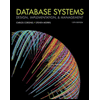
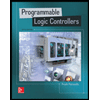