The following table contains output from a lasso fit to a linear model with d = 6 variables and n = 70 observations. Starting from the left, the columns are λ, and B1, ..., B6, i.e. each row has and the transposed column vector ẞ(λ). 0.00000 0.14473 -0.01238 -0.16257 -0.02082 -0.19404 0.08851 0.58488 0.13477 0.00000 -0.14698 -0.00910 1.12126 0.12656 0.00000 -0.13565 0.00000 7.27740 0.03425 0.00000 -0.02504 0.00000 8.69332 0.01007 0.00000 0.00000 0.00000 -0.02773 0.00000 9.29266 0.00000 0.00000 0.00000 0.00000 -0.01778 0.00000 10.52807 0.00000 0.00000 0.00000 0.00000 0.00000 0.00000 -0.18503 0.08213 -0.17573 0.07534 -0.05573 0.00000 For each of the required computations below, briefly report your procedure and the required quantity. a) For each row in the table, compute s, the proportion of shrinkage defined ass = s(λ) = ||B(a)||₁/max ||B(1)||1. b) Consider λ = 8.99299. Note that λ' is the intermediate value between λ = 8.69332 and λ = 9.29266 of the 5th and 6th rows above. Using this value of 1', compute and report the shrunk estimator B(λ'). c) Consider the vector of predictors x =(-0.84507, -1.09859, -0.92958, 1.09859, -0.90141, -0.92958). Using your shrunk estimator B(λ') (as column vector) compute the predicted value y^= x² ß(λ'). d) Repeat b) for the regularization parameter taking value λ" = 11, that is, determine ẞ(λ").
The following table contains output from a lasso fit to a linear model with d = 6 variables and n = 70 observations. Starting from the left, the columns are λ, and B1, ..., B6, i.e. each row has and the transposed column vector ẞ(λ). 0.00000 0.14473 -0.01238 -0.16257 -0.02082 -0.19404 0.08851 0.58488 0.13477 0.00000 -0.14698 -0.00910 1.12126 0.12656 0.00000 -0.13565 0.00000 7.27740 0.03425 0.00000 -0.02504 0.00000 8.69332 0.01007 0.00000 0.00000 0.00000 -0.02773 0.00000 9.29266 0.00000 0.00000 0.00000 0.00000 -0.01778 0.00000 10.52807 0.00000 0.00000 0.00000 0.00000 0.00000 0.00000 -0.18503 0.08213 -0.17573 0.07534 -0.05573 0.00000 For each of the required computations below, briefly report your procedure and the required quantity. a) For each row in the table, compute s, the proportion of shrinkage defined ass = s(λ) = ||B(a)||₁/max ||B(1)||1. b) Consider λ = 8.99299. Note that λ' is the intermediate value between λ = 8.69332 and λ = 9.29266 of the 5th and 6th rows above. Using this value of 1', compute and report the shrunk estimator B(λ'). c) Consider the vector of predictors x =(-0.84507, -1.09859, -0.92958, 1.09859, -0.90141, -0.92958). Using your shrunk estimator B(λ') (as column vector) compute the predicted value y^= x² ß(λ'). d) Repeat b) for the regularization parameter taking value λ" = 11, that is, determine ẞ(λ").
Advanced Engineering Mathematics
10th Edition
ISBN:9780470458365
Author:Erwin Kreyszig
Publisher:Erwin Kreyszig
Chapter2: Second-order Linear Odes
Section: Chapter Questions
Problem 1RQ
Related questions
Question

Transcribed Image Text:The following table contains output from a lasso fit to a linear model with d = 6 variables and n = 70 observations. Starting from
the left, the columns are λ, and B1, ..., B6, i.e. each row has and the transposed column vector ẞ(λ).
0.00000
0.14473
-0.01238
-0.16257
-0.02082
-0.19404 0.08851
0.58488 0.13477 0.00000 -0.14698 -0.00910
1.12126 0.12656 0.00000 -0.13565 0.00000
7.27740 0.03425 0.00000 -0.02504 0.00000
8.69332 0.01007 0.00000 0.00000 0.00000 -0.02773 0.00000
9.29266 0.00000 0.00000 0.00000 0.00000 -0.01778 0.00000
10.52807 0.00000 0.00000 0.00000 0.00000 0.00000 0.00000
-0.18503 0.08213
-0.17573 0.07534
-0.05573 0.00000
For each of the required computations below, briefly report your procedure and the required quantity.
a) For each row in the table, compute s, the proportion of shrinkage defined ass = s(λ) = ||B(a)||₁/max ||B(1)||1.
b) Consider λ = 8.99299. Note that λ' is the intermediate value between λ = 8.69332 and λ = 9.29266 of the 5th and 6th rows
above. Using this value of 1', compute and report the shrunk estimator B(λ').
c) Consider the vector of predictors x =(-0.84507, -1.09859, -0.92958, 1.09859, -0.90141, -0.92958). Using your shrunk estimator
B(λ') (as column vector) compute the predicted value y^= x² ß(λ').
d) Repeat b) for the regularization parameter taking value λ" = 11, that is, determine ẞ(λ").
Expert Solution

This question has been solved!
Explore an expertly crafted, step-by-step solution for a thorough understanding of key concepts.
Step by step
Solved in 2 steps

Recommended textbooks for you
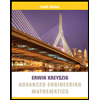
Advanced Engineering Mathematics
Advanced Math
ISBN:
9780470458365
Author:
Erwin Kreyszig
Publisher:
Wiley, John & Sons, Incorporated
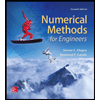
Numerical Methods for Engineers
Advanced Math
ISBN:
9780073397924
Author:
Steven C. Chapra Dr., Raymond P. Canale
Publisher:
McGraw-Hill Education
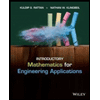
Introductory Mathematics for Engineering Applicat…
Advanced Math
ISBN:
9781118141809
Author:
Nathan Klingbeil
Publisher:
WILEY
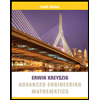
Advanced Engineering Mathematics
Advanced Math
ISBN:
9780470458365
Author:
Erwin Kreyszig
Publisher:
Wiley, John & Sons, Incorporated
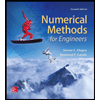
Numerical Methods for Engineers
Advanced Math
ISBN:
9780073397924
Author:
Steven C. Chapra Dr., Raymond P. Canale
Publisher:
McGraw-Hill Education
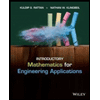
Introductory Mathematics for Engineering Applicat…
Advanced Math
ISBN:
9781118141809
Author:
Nathan Klingbeil
Publisher:
WILEY
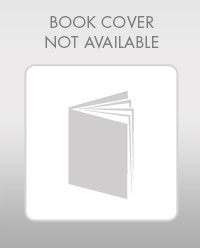
Mathematics For Machine Technology
Advanced Math
ISBN:
9781337798310
Author:
Peterson, John.
Publisher:
Cengage Learning,
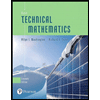
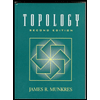