Regression StatisticsMultiple R 0.890579188 Business Administration Program Final Assessment Semester Spring 2020Page 4R Square 0.793131289Adjusted R Square 0.7379663Standard Error 30.28395534Observations 20ANOVA df SS MS FRegression 4 52743.23074 13185.81 14.37743932Residual 15 13756.76926 917.1179509 Total 19 66500 Coefficients Standard Error t Stat P-valueIntercept 73.33291 62.25276 1.17799 0.25715X1 -0.13882 0.05353 -2.59326 0.02037X2 3.73984 0.95568 3.91328 0.00138X3 0.37644 0.16876 2.23061 0.04140X4 17.70752 15.57847 1.13667 0.27351 a. Write down the fitted multiple regression equation
Correlation
Correlation defines a relationship between two independent variables. It tells the degree to which variables move in relation to each other. When two sets of data are related to each other, there is a correlation between them.
Linear Correlation
A correlation is used to determine the relationships between numerical and categorical variables. In other words, it is an indicator of how things are connected to one another. The correlation analysis is the study of how variables are related.
Regression Analysis
Regression analysis is a statistical method in which it estimates the relationship between a dependent variable and one or more independent variable. In simple terms dependent variable is called as outcome variable and independent variable is called as predictors. Regression analysis is one of the methods to find the trends in data. The independent variable used in Regression analysis is named Predictor variable. It offers data of an associated dependent variable regarding a particular outcome.
Regression Statistics
Multiple R
0.89057918
8
Business Administration Program Final Assessment Semester Spring 2020Page 4
R Square
0.79313128
9
Adjusted R Square 0.7379663
Standard Error
30.2839553
4
Observations 20
ANOVA
df SS MS F
Regression 4 52743.23074 13185.81 14.3774393
2
Residual 15 13756.76926 917.117950
9
Total 19 66500
Coefficients Standard Error t Stat P-value
Intercept 73.33291 62.25276 1.17799 0.25715
X1 -0.13882 0.05353 -2.59326 0.02037
X2 3.73984 0.95568 3.91328 0.00138
X3 0.37644 0.16876 2.23061 0.04140
X4 17.70752 15.57847 1.13667 0.27351
a. Write down the fitted multiple regression equation

Step by step
Solved in 2 steps with 2 images

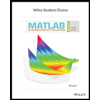
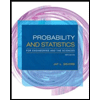
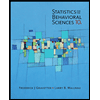
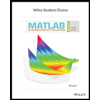
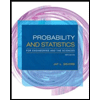
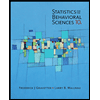
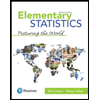
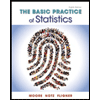
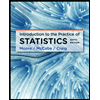