tudy the regression output that follows. How many predictors are there? What is the equation of the regression model? Regression Analysis: y versus x1, x2, x3, x4 Summary Output Regression Statistics R Square 0.802 Adjusted R Square 0.787 Standard Error 9.025 Observations 60 ANOVA df SS MS F p Regression 4 18,088.5 4,522.1 55.52 0.000 Residual 55 4,479.7 81.4 Total 59 22,568.2 Coefficients Standard Error t Stats P-Value Constant −55.93 24.22 −2.31 0.025 x1 0.01049 0.021 0.5 0.619 x2 −0.1072 0.03503 −3.06 0.003 x3 0.57922 0.07633 7.59 0.000 x4 −0.8695 0.1498 −5.81 0.000 (Round all numbers to 2 decimal places, e.g. 1.75.) Question: The number of predictors is: k = ? The regression model is : ŷ = ? + ? x1 + ? x2 + ? x3 + ?
Correlation
Correlation defines a relationship between two independent variables. It tells the degree to which variables move in relation to each other. When two sets of data are related to each other, there is a correlation between them.
Linear Correlation
A correlation is used to determine the relationships between numerical and categorical variables. In other words, it is an indicator of how things are connected to one another. The correlation analysis is the study of how variables are related.
Regression Analysis
Regression analysis is a statistical method in which it estimates the relationship between a dependent variable and one or more independent variable. In simple terms dependent variable is called as outcome variable and independent variable is called as predictors. Regression analysis is one of the methods to find the trends in data. The independent variable used in Regression analysis is named Predictor variable. It offers data of an associated dependent variable regarding a particular outcome.
Study the regression output that follows. How many predictors are there? What is the equation of the regression model?
Summary Output | ||
---|---|---|
Regression Statistics
|
||
R Square
|
0.802 | |
Adjusted R Square
|
0.787 | |
Standard Error
|
9.025 | |
Observations
|
60 |
ANOVA
|
df
|
SS
|
MS
|
F
|
p
|
|||||
---|---|---|---|---|---|---|---|---|---|---|
Regression | 4 | 18,088.5 | 4,522.1 | 55.52 | 0.000 | |||||
Residual | 55 | 4,479.7 | 81.4 | |||||||
Total | 59 | 22,568.2 |
Coefficients
|
Standard
Error |
t Stats
|
P-Value
|
|||||
---|---|---|---|---|---|---|---|---|
Constant
|
−55.93 | 24.22 | −2.31 | 0.025 | ||||
x1 | 0.01049 | 0.021 | 0.5 | 0.619 | ||||
x2 | −0.1072 | 0.03503 | −3.06 | 0.003 | ||||
x3 | 0.57922 | 0.07633 | 7.59 | 0.000 | ||||
x4 | −0.8695 | 0.1498 | −5.81 | 0.000 |
(Round all numbers to 2 decimal places, e.g. 1.75.)
Question:
The number of predictors is:
k = ? |
The regression model is :
ŷ = ? + ? x1 + ? x2 + ? x3 + ? |

Step by step
Solved in 2 steps

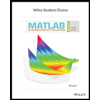
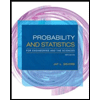
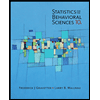
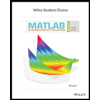
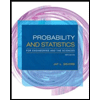
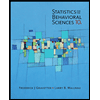
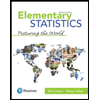
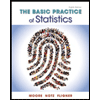
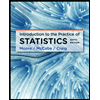