Problem 4: Generalization Bounds for Deep Neural Networks via Rademacher Complexity Statement: Derive generalization bounds for deep neural networks by computing the Rademacher complexity of the hypothesis class defined by networks with bounded weights and specific architectural constraints. Show how these bounds scale with the depth and width of the network. Key Points for the Proof: • • • Define the hypothesis class of neural networks under consideration. Calculate or bound the Rademacher complexity for this class, considering factors like depth, width, and weight constraints. Apply concentration inequalities to relate Rademacher complexity to generalization error. Analyze how the derived bounds behave as the network's depth and width increase.
Problem 4: Generalization Bounds for Deep Neural Networks via Rademacher Complexity Statement: Derive generalization bounds for deep neural networks by computing the Rademacher complexity of the hypothesis class defined by networks with bounded weights and specific architectural constraints. Show how these bounds scale with the depth and width of the network. Key Points for the Proof: • • • Define the hypothesis class of neural networks under consideration. Calculate or bound the Rademacher complexity for this class, considering factors like depth, width, and weight constraints. Apply concentration inequalities to relate Rademacher complexity to generalization error. Analyze how the derived bounds behave as the network's depth and width increase.
College Algebra (MindTap Course List)
12th Edition
ISBN:9781305652231
Author:R. David Gustafson, Jeff Hughes
Publisher:R. David Gustafson, Jeff Hughes
Chapter6: Linear Systems
Section6.8: Linear Programming
Problem 40E
Related questions
Question

Transcribed Image Text:Problem 4: Generalization Bounds for Deep Neural Networks via
Rademacher Complexity
Statement: Derive generalization bounds for deep neural networks by computing the Rademacher
complexity of the hypothesis class defined by networks with bounded weights and specific
architectural constraints. Show how these bounds scale with the depth and width of the network.
Key Points for the Proof:
•
•
•
Define the hypothesis class of neural networks under consideration.
Calculate or bound the Rademacher complexity for this class, considering factors like depth,
width, and weight constraints.
Apply concentration inequalities to relate Rademacher complexity to generalization error.
Analyze how the derived bounds behave as the network's depth and width increase.
Expert Solution

This question has been solved!
Explore an expertly crafted, step-by-step solution for a thorough understanding of key concepts.
Step by step
Solved in 2 steps with 5 images

Recommended textbooks for you
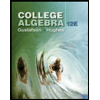
College Algebra (MindTap Course List)
Algebra
ISBN:
9781305652231
Author:
R. David Gustafson, Jeff Hughes
Publisher:
Cengage Learning
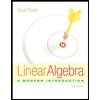
Linear Algebra: A Modern Introduction
Algebra
ISBN:
9781285463247
Author:
David Poole
Publisher:
Cengage Learning
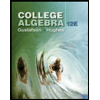
College Algebra (MindTap Course List)
Algebra
ISBN:
9781305652231
Author:
R. David Gustafson, Jeff Hughes
Publisher:
Cengage Learning
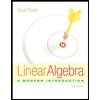
Linear Algebra: A Modern Introduction
Algebra
ISBN:
9781285463247
Author:
David Poole
Publisher:
Cengage Learning