Problem 6: Identifiability of Neural Network Parameters Statement: Investigate the conditions under which the parameters (weights and biases) of a neural network are identifiable, meaning that different parameter settings do not produce the same function. Provide a proof outlining necessary and sufficient conditions for identifiability in multi-layer perceptrons. Key Points for the Proof: • Explore the role of activation functions in parameter identifiability. Examine the impact of network architecture (e.g., number of layers, neurons per layer) on identifiability. • Address permutation symmetries and scaling ambiguities inherent in neural network parameters. Establish conditions that eliminate these ambiguities to achieve identifiability.
Problem 6: Identifiability of Neural Network Parameters Statement: Investigate the conditions under which the parameters (weights and biases) of a neural network are identifiable, meaning that different parameter settings do not produce the same function. Provide a proof outlining necessary and sufficient conditions for identifiability in multi-layer perceptrons. Key Points for the Proof: • Explore the role of activation functions in parameter identifiability. Examine the impact of network architecture (e.g., number of layers, neurons per layer) on identifiability. • Address permutation symmetries and scaling ambiguities inherent in neural network parameters. Establish conditions that eliminate these ambiguities to achieve identifiability.
Advanced Engineering Mathematics
10th Edition
ISBN:9780470458365
Author:Erwin Kreyszig
Publisher:Erwin Kreyszig
Chapter2: Second-order Linear Odes
Section: Chapter Questions
Problem 1RQ
Related questions
Question

Transcribed Image Text:Problem 6: Identifiability of Neural Network Parameters
Statement: Investigate the conditions under which the parameters (weights and biases) of a neural
network are identifiable, meaning that different parameter settings do not produce the same
function. Provide a proof outlining necessary and sufficient conditions for identifiability in multi-layer
perceptrons.
Key Points for the Proof:
•
Explore the role of activation functions in parameter identifiability.
Examine the impact of network architecture (e.g., number of layers, neurons per layer) on
identifiability.
• Address permutation symmetries and scaling ambiguities inherent in neural network
parameters.
Establish conditions that eliminate these ambiguities to achieve identifiability.
Expert Solution

This question has been solved!
Explore an expertly crafted, step-by-step solution for a thorough understanding of key concepts.
Step by step
Solved in 2 steps with 3 images

Recommended textbooks for you
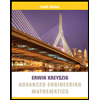
Advanced Engineering Mathematics
Advanced Math
ISBN:
9780470458365
Author:
Erwin Kreyszig
Publisher:
Wiley, John & Sons, Incorporated
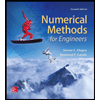
Numerical Methods for Engineers
Advanced Math
ISBN:
9780073397924
Author:
Steven C. Chapra Dr., Raymond P. Canale
Publisher:
McGraw-Hill Education
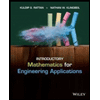
Introductory Mathematics for Engineering Applicat…
Advanced Math
ISBN:
9781118141809
Author:
Nathan Klingbeil
Publisher:
WILEY
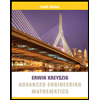
Advanced Engineering Mathematics
Advanced Math
ISBN:
9780470458365
Author:
Erwin Kreyszig
Publisher:
Wiley, John & Sons, Incorporated
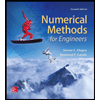
Numerical Methods for Engineers
Advanced Math
ISBN:
9780073397924
Author:
Steven C. Chapra Dr., Raymond P. Canale
Publisher:
McGraw-Hill Education
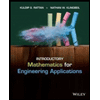
Introductory Mathematics for Engineering Applicat…
Advanced Math
ISBN:
9781118141809
Author:
Nathan Klingbeil
Publisher:
WILEY
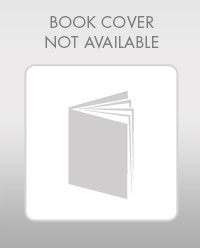
Mathematics For Machine Technology
Advanced Math
ISBN:
9781337798310
Author:
Peterson, John.
Publisher:
Cengage Learning,
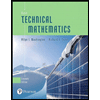
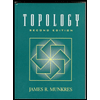