j) Plot the residuals versus ŷ. Interpret this plot. k) Construct a normal probability plot of the residuals and comment on the normality assump- tion.
Correlation
Correlation defines a relationship between two independent variables. It tells the degree to which variables move in relation to each other. When two sets of data are related to each other, there is a correlation between them.
Linear Correlation
A correlation is used to determine the relationships between numerical and categorical variables. In other words, it is an indicator of how things are connected to one another. The correlation analysis is the study of how variables are related.
Regression Analysis
Regression analysis is a statistical method in which it estimates the relationship between a dependent variable and one or more independent variable. In simple terms dependent variable is called as outcome variable and independent variable is called as predictors. Regression analysis is one of the methods to find the trends in data. The independent variable used in Regression analysis is named Predictor variable. It offers data of an associated dependent variable regarding a particular outcome.
Kindly help me with j) k). Thank you!
Previous interpretation as below:
(a) Thus, the multiple linear regression line is,
y = (–102.7132) + 0.6054 x1 + 8.9236 x2 + 1.4375 x3 + 0.0136 x4
(b) y = (–102.7132) + 0.6054 (75) + 8.9236 (24) + 1.4375 (90) + 0.0136 (98)
= (–102.7132) + 45.405 + 214.1664 + 129.375 + 1.3328
= 287.566
(c) The P-value for the overall model is 0.030302769.
It is known that, if the P-value is less than or equal to the level of significance, then reject the null hypothesis. Or else, fail to reject the null hypothesis. Here, the level of significance is 0.05. Hence, the obtained P-value is less than the level of significance. Thus, the null hypothesis is rejected. Therefore, there is enough evidence to claim that the regression model is significant at 5% level of significance.
(d) The mean square error is the estimate value of population variance. From the output, MSE = 242.7156. Thus, estimated value of σ2 is 242.7156.
(e) For x1: From the output it is obtained that the P-value corresponding to the t-test of x1 is 0.1448, which is greater than the level of significance of 0.05. Hence, the null hypothesis is not rejected. Thus, there is not enough evidence to claim that there is significance relationship between x1 and y, when the other predictors are constant at 5% level of significance.
For x2: From the output it is obtained that the P-value corresponding to the t-test of x2 is 0.1361, which is greater than the level of significance of 0.05. Hence, the null hypothesis is not rejected. Thus, there is not enough evidence to claim that there is significance relationship between x2 and y, when the other predictors are constant at 5% level of significance.
For x3: From the output it is obtained that the P-value corresponding to the t-test of x3 is 0.5668, which is greater than the level of significance of 0.05. Hence, the null hypothesis is not rejected. Thus, there is not enough evidence to claim that there is significance relationship between x3 and y, when the other predictors are constant at 5% level of significance.
For x4: From the output it is obtained that the P-value corresponding to the t-test of x4 is 0.9857, which is greater than the level of significance of 0.05. Hence, the null hypothesis is not rejected. Thus, there is not enough evidence to claim that there is significance relationship between x4 and y, when the other predictors are constant at 5% level of significance.
(f) From the output, the 95% confidence interval for β1 is (–0.2669, 1.4777).
From the output, the 95% confidence interval for β2 is (–3.6101, 21.4574).
From the output, the 95% confidence interval for β3 is (–4.2178, 7.0927).
From the output, the 95% confidence interval for β4 is (–1.7216, 1.7488).
(g)
The 95% confidence interval on the mean of Y for the values of regressors from part (b) is (263.7879, 311.3357).
(h) The 95% prediction interval on the power consumption for the values of regressors from part (b) is (243.7175, 331.4062).
(i) The value of R2 is 0.7447 which indicates that fit of the model. This shows that there is 74.47% of the variance in the dependent variable is collectively explained by the independent variables in the model.
The value of adjusted R2 is 0.5989 which is a modified version of R2 which accounts for the predictors that are not significant in the regression model. As the obtained value is more than 50%, that is, 59.89%, it can be said that the model is acceptable.



Trending now
This is a popular solution!
Step by step
Solved in 2 steps with 2 images

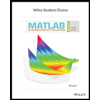
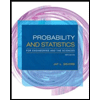
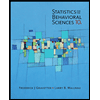
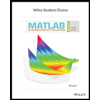
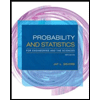
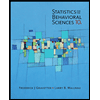
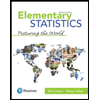
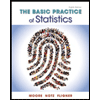
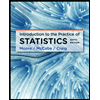