In class, we derived linear regression and various learning algorithms based on gradient descent. In addition to the least square objective, we also learned its probabilistic perspective where each observation is assumed to have a Gaussian noise. (i.e., Noise of each example is an independent and identically distributed sample from a normal distribution) In this problem, you are supposed to deal with the following regression model that includes one feature x1 that relates linearly/quadratically and another linear feature x2. y = 00 + 01x1+02x2+ 03xi+ € where e ~ N(0, o²) You are provided with a training observations D = {(x{", x2", y(?)|1 < i < m}.Derive the conditional log-likelihood that will be later maximized to make D most likely. m 1 m log 1 Ozx – 0zx")? - - - - 202 i=1
In class, we derived linear regression and various learning algorithms based on gradient descent. In addition to the least square objective, we also learned its probabilistic perspective where each observation is assumed to have a Gaussian noise. (i.e., Noise of each example is an independent and identically distributed sample from a normal distribution) In this problem, you are supposed to deal with the following regression model that includes one feature x1 that relates linearly/quadratically and another linear feature x2. y = 00 + 01x1+02x2+ 03xi+ € where e ~ N(0, o²) You are provided with a training observations D = {(x{", x2", y(?)|1 < i < m}.Derive the conditional log-likelihood that will be later maximized to make D most likely. m 1 m log 1 Ozx – 0zx")? - - - - 202 i=1
MATLAB: An Introduction with Applications
6th Edition
ISBN:9781119256830
Author:Amos Gilat
Publisher:Amos Gilat
Chapter1: Starting With Matlab
Section: Chapter Questions
Problem 1P
Related questions
Question

Transcribed Image Text:In class, we derived linear regression and various learning algorithms based on gradient
descent. In addition to the least square objective, we also learned its probabilistic perspective
where each observation is assumed to have a Gaussian noise. (i.e., Noise of each example is an
independent and identically distributed sample from a normal distribution) In this problem, you
are supposed to deal with the following regression model that includes one feature x1 that
relates linearly/quadratically and another linear feature x2.
y = 00 + 01x1+02x2+ 03xi+ € where e ~
N(0, o²)
You are provided with a training observations D = {(x{", x2", y(?)|1 < i < m}.Derive the
conditional log-likelihood that will be later maximized to make D most likely.
m
1
m log-
1
(y® – 0o – O1rf° – 62x2"
-
-
-
202
i=1
(y() – 6o – 0,xfº – 02x – Ozxf1²)²,
m
1
log-
exp(-
202
i=1
m
1
m log 2no
1
(y) – 0o – O,x – 02x
– Ozxf?)²
|
-
202
i=1
Expert Solution

This question has been solved!
Explore an expertly crafted, step-by-step solution for a thorough understanding of key concepts.
Step by step
Solved in 3 steps with 3 images

Recommended textbooks for you
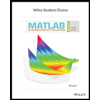
MATLAB: An Introduction with Applications
Statistics
ISBN:
9781119256830
Author:
Amos Gilat
Publisher:
John Wiley & Sons Inc
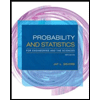
Probability and Statistics for Engineering and th…
Statistics
ISBN:
9781305251809
Author:
Jay L. Devore
Publisher:
Cengage Learning
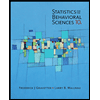
Statistics for The Behavioral Sciences (MindTap C…
Statistics
ISBN:
9781305504912
Author:
Frederick J Gravetter, Larry B. Wallnau
Publisher:
Cengage Learning
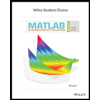
MATLAB: An Introduction with Applications
Statistics
ISBN:
9781119256830
Author:
Amos Gilat
Publisher:
John Wiley & Sons Inc
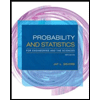
Probability and Statistics for Engineering and th…
Statistics
ISBN:
9781305251809
Author:
Jay L. Devore
Publisher:
Cengage Learning
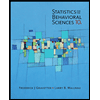
Statistics for The Behavioral Sciences (MindTap C…
Statistics
ISBN:
9781305504912
Author:
Frederick J Gravetter, Larry B. Wallnau
Publisher:
Cengage Learning
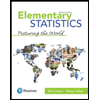
Elementary Statistics: Picturing the World (7th E…
Statistics
ISBN:
9780134683416
Author:
Ron Larson, Betsy Farber
Publisher:
PEARSON
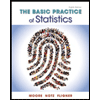
The Basic Practice of Statistics
Statistics
ISBN:
9781319042578
Author:
David S. Moore, William I. Notz, Michael A. Fligner
Publisher:
W. H. Freeman
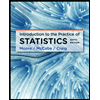
Introduction to the Practice of Statistics
Statistics
ISBN:
9781319013387
Author:
David S. Moore, George P. McCabe, Bruce A. Craig
Publisher:
W. H. Freeman