Please see problem with the solution: after reviewing the information below, assess the appropriateness and accuracy of using a linear regression model. Discuss the meaning of the standard error of the estimate and how it affects the predicted values of Y for that analysis. To predict the air travel industries future, I looked at the 2018 and 2019 travel data from the Bureau of Transportation Statistics (BTA, 2019). With a breakdown in month to month data, I am not sure if this is a good model. If I was to try and predict 2020's numbers through the rest of the year, my model would be way off with the Corona Virus halting most travel. And a month over month analysis, without Corona say, is probably not good enough. I couldn't use March of 2018 to predict November of 2019. Below is an analysis of the two years for review.
Correlation
Correlation defines a relationship between two independent variables. It tells the degree to which variables move in relation to each other. When two sets of data are related to each other, there is a correlation between them.
Linear Correlation
A correlation is used to determine the relationships between numerical and categorical variables. In other words, it is an indicator of how things are connected to one another. The correlation analysis is the study of how variables are related.
Regression Analysis
Regression analysis is a statistical method in which it estimates the relationship between a dependent variable and one or more independent variable. In simple terms dependent variable is called as outcome variable and independent variable is called as predictors. Regression analysis is one of the methods to find the trends in data. The independent variable used in Regression analysis is named Predictor variable. It offers data of an associated dependent variable regarding a particular outcome.
Please see problem with the solution: after reviewing the information below, assess the appropriateness and accuracy of using a linear regression model. Discuss the meaning of the standard error of the estimate and how it affects the predicted values of Y for that analysis.
To predict the air travel industries future, I looked at the 2018 and 2019 travel data from the Bureau of Transportation Statistics (BTA, 2019). With a breakdown in month to month data, I am not sure if this is a good model. If I was to try and predict 2020's numbers through the rest of the year, my model would be way off with the Corona Virus halting most travel. And a month over month analysis, without Corona say, is probably not good enough. I couldn't use March of 2018 to predict November of 2019. Below is an analysis of the two years for review.
Simple linear regression results:
Dependent Variable: 2018
Independent Variable: 2019
2018 = 0.93316444 + 0.9520346 2019
R (
R-sq = 0.9680617
Estimate of error standard deviation: 1.4640167
Parameter estimates:
Parameter | Estimate | Std. Err. | Alternative | DF | T-Stat | P-value |
---|---|---|---|---|---|---|
Intercept | 0.93316444 | 4.8161538 | ≠ 0 | 10 | 0.19375719 | 0.8502 |
Slope | 0.9520346 | 0.054683605 | ≠ 0 | 10 | 17.409873 | <0.0001 |
Analysis of variance table for regression model:
Source | DF | SS | MS | F-stat | P-value |
---|---|---|---|---|---|
Model | 1 | 649.65572 | 649.65572 | 303.10367 | <0.0001 |
Error | 10 | 21.43345 | 2.143345 | ||
Total | 11 | 671.08917 |

Trending now
This is a popular solution!
Step by step
Solved in 2 steps

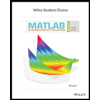
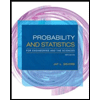
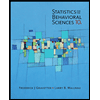
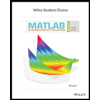
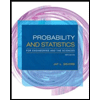
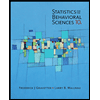
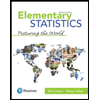
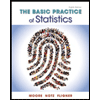
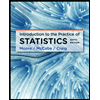