import numpy as npfrom scipy.optimize import minimizeimport matplotlib.pyplot as pltimport pickle #Implement the function regressionObjVal def regressionObjVal(w, X, y): # compute squared error (scalar) with respect # to w (vector) for the given data X and y # # Inputs: # w = d x 1 # X = N x d # y = N x 1 # Output: # error = scalar value (which is the error calcualted using objective function) # IMPLEMENT THIS METHOD - REMOVE THE NEXT LINE error = 0 return error #Implement the function regressionGradient def regressionGradient(w, X, y): # compute gradient of squared error (scalar) with respect # to w (vector) for the given data X and y # Inputs: # w = d x 1 # X = N x d # y = N x 1 # Output: # gradient = d length vector (not a d x 1 matrix) if len(w.shape) == 1: w = w[:,np.newaxis] # IMPLEMENT THIS METHOD - REMOVE THE NEXT LINE error_grad = np.zeros((X.shape[1],)) return error_grad Xtrain,ytrain,Xtest,ytest = pickle.load(open('diabetes.pickle','rb'),encoding='latin1') # add interceptXtrain_i = np.concatenate((np.ones((Xtrain.shape[0],1)), Xtrain), axis=1)Xtest_i = np.concatenate((np.ones((Xtest.shape[0],1)), Xtest), axis=1)args = (Xtrain_i,ytrain)opts = {'maxiter' : 50} w_init = np.zeros((Xtrain_i.shape[1],))soln = minimize(regressionObjVal, w_init, jac=regressionGradient, args=args,method='CG', options=opts)w = np.transpose(np.array(soln.x))w = np.reshape(w,[len(w),1])mse = calRegressionError(w,Xtrain_i,ytrain)print('Gradient Descent Linear Regression MSE on train data - %.2f'%mse)mse = calRegressionError(w,Xtest_i,ytest)print('Gradient Descent Linear Regression MSE on test data - %.2f'%mse)
import numpy as np
from scipy.optimize import minimize
import matplotlib.pyplot as plt
import pickle
#Implement the function regressionObjVal
def regressionObjVal(w, X, y):
# compute squared error (scalar) with respect
# to w (
#
# Inputs:
# w = d x 1
# X = N x d
# y = N x 1
# Output:
# error = scalar value (which is the error calcualted using objective function)
# IMPLEMENT THIS METHOD - REMOVE THE NEXT LINE
error = 0
return error
#Implement the function regressionGradient
def regressionGradient(w, X, y):
# compute gradient of squared error (scalar) with respect
# to w (vector) for the given data X and y
# Inputs:
# w = d x 1
# X = N x d
# y = N x 1
# Output:
# gradient = d length vector (not a d x 1 matrix)
if len(w.shape) == 1:
w = w[:,np.newaxis]
# IMPLEMENT THIS METHOD - REMOVE THE NEXT LINE
error_grad = np.zeros((X.shape[1],))
return error_grad
Xtrain,ytrain,Xtest,ytest = pickle.load(open('diabetes.pickle','rb'),encoding='latin1')
# add intercept
Xtrain_i = np.concatenate((np.ones((Xtrain.shape[0],1)), Xtrain), axis=1)
Xtest_i = np.concatenate((np.ones((Xtest.shape[0],1)), Xtest), axis=1)
args = (Xtrain_i,ytrain)
opts = {'maxiter' : 50}
w_init = np.zeros((Xtrain_i.shape[1],))
soln = minimize(regressionObjVal, w_init, jac=regressionGradient, args=args,method='CG', options=opts)
w = np.transpose(np.array(soln.x))
w = np.reshape(w,[len(w),1])
mse = calRegressionError(w,Xtrain_i,ytrain)
print('Gradient Descent Linear Regression MSE on train data - %.2f'%mse)
mse = calRegressionError(w,Xtest_i,ytest)
print('Gradient Descent Linear Regression MSE on test data - %.2f'%mse)

Step by step
Solved in 2 steps

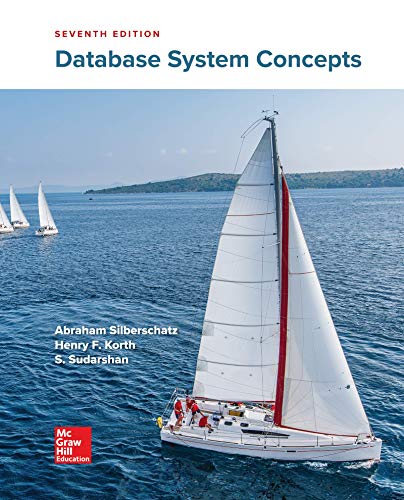
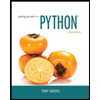
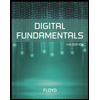
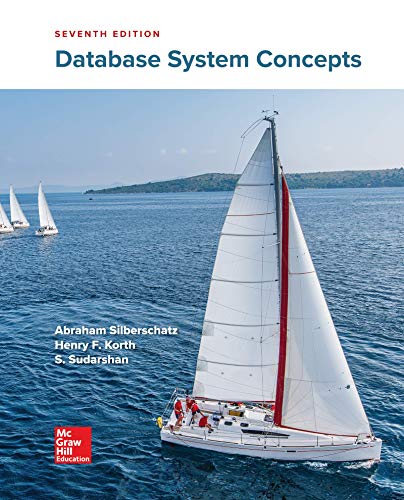
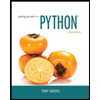
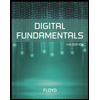
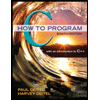
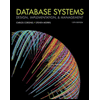
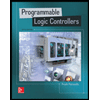