My program works with a CSV file with three columns: names, cities, and heights. I need to create a table that determines the difference in height from the average city size. This allows me to decide which two people have the minimum difference from the average. These difference tables must be in Python lists for each city. They must not use tuples, dictionaries, named functions, or the key or lambda function to sort it. What are some ways I could code for this? import csv data = [] with open("C:\\Users\\lucas\\Downloads\\sample-data.csv") as f: reader = csv.reader(f) for row in csv.reader(f): data.append(list(row)) f.close() data.pop(0) # print(data) city_list = [] city = row[1] for i in range(len(data)): city_list.append(data[i][1]) city_list.sort() print(city_list) unique_cities = [] people_count = [] for city in city_list: # this if statement analy
My program works with a CSV file with three columns: names, cities, and heights. I need to create a table that determines the difference in height from the average city size. This allows me to decide which two people have the minimum difference from the average. These difference tables must be in Python lists for each city. They must not use tuples, dictionaries, named functions, or the key or lambda function to sort it. What are some ways I could code for this?
import csv
data = []
with open("C:\\Users\\lucas\\Downloads\\sample-data.csv") as f:
reader = csv.reader(f)
for row in csv.reader(f):
data.append(list(row))
f.close()
data.pop(0)
# print(data)
city_list = []
city = row[1]
for i in range(len(data)):
city_list.append(data[i][1])
city_list.sort()
print(city_list)
unique_cities = []
people_count = []
for city in city_list:
# this if statement analyzes which cities are in unique_cities. If they are not in the list,
# then they will be appended into the list
if city not in unique_cities:
unique_cities.append(city)
for city in unique_cities:
# because unique cities lists one of each city,
# and because we want to count the frequency of the cities, we use city_list
count = city_list.count(city)
people_count.append(count)
print(unique_cities)
print(people_count)
height_list = []
for d in data:
if city == d[1]:
height_list.append(float(d[2]))
avg = sum(height_list)/len(height_list)

Trending now
This is a popular solution!
Step by step
Solved in 3 steps with 1 images

How could this program work without items() or enumerate?
def Find_heights(data):
heights = []
for d in data:
city = d[1]
height = float(d[2])
index = -1
for i, item in enumerate(heights):
if item[0] == city:
index = i
break
if index == -1:
heights.append([city, [height]])
else:
heights[index][1].append(height)
return heights
height_list = Find_heights(csv_data)
print(height_list)
# Output: [['New York', [5.8, 5.9, 6.0]], ['Los Angeles', [5.5, 5.6, 5.7]], ['Chicago', [5.9, 6.0, 6.1]], ['Houston', [5.6, 5.7, 5.8]]]
#To remove the items from the Find_average function, you can modify it to return a dictionary with city names as keys and average heights as values. Here's an updated version of the function:
def Find_average(heights_list):
averages = {}
for city_data in heights_list:
city = city_data[0]
heights = city_data[1]
average = sum(heights) / len(heights)
averages[city] = average
return averages
How could this code work with...
- lists instead of dictionaries?
- without the items as in...
- def Find_average(heights_dict):
for city, heights in heights_dict.items():
average = sum(heights) / len(heights)
print(f"{city}: {average}")
- def Find_average(heights_dict):
-
import csvcsv_data = []
with open("C:\\Users\\lucas\\Downloads\\sample-data.csv") as f:
reader = csv.reader(f)
for row in csv.reader(f):
csv_data .append(list(row))
f.close()
csv_data.pop(0)difference_list = []
def Sorting_cities(data):
cities = []
for i in range(len(data)):
cities.append(data[i][1])
cities.sort()
return cities
city_list = Sorting_cities(csv_data)
print(city_list)
def Listing_cities(cities):
single_cities = []
for city in cities:
if city not in single_cities:
single_cities.append(city)
return single_cities
unique_cities = Listing_cities(city_list)
print(unique_cities)
def Counting_cities_and_people(single_cities, cities):
people_count = []
for city in single_cities:
count = cities.count(city)
people_count.append(count)
for k in range(len(single_cities)):
print(f'City: {single_cities[k]}, Population: {people_count[k]}')
return people_count
population = Counting_cities_and_people(unique_cities, city_list)
print(population)
def Find_heights(data):
heights = []
for d in data:
city = d[1]
height = float(d[2])
if city not in heights:
heights[city] = [height]
else:
heights[city].append(height)
return heights
height_list = Find_heights(csv_data)
print(height_list)
def Find_averages(heights):
for city, heights in heights:
for averages in heights:
averages = sum(heights)/ len(heights)
return averages
def Find_names(data):
names = []
for city in unique_cities:
for d in data:
if city == d[1]:
names.append(d[0])
return names
name_list = Find_names(csv_data)
print(name_list)
def Find_indexes(unique_city, data_set):
index_list = []
index = 0
for row in data_set:
if row[1] == unique_city:
index_list.append(index)
index += 1
return index_list
indexes = Find_indexes(unique_cities, csv_data)def Find_difference():
for height in height_list:
diff = abs(height - avg)
difference_list.append(diff)index = 0
closest_to_average_height = 1000000000
closest_to_average_name = " "
second_closest_height = 1000000000
second_closest_name = "placeholder"difference_list_two = difference_list.copy()
closest_to_average_diff = 1000000000
second_closest_to_average_diff = closest_to_average_diffdifference_list.sort()
for index in range(len(height_list)):
current_diff = difference_list_two[index]
if current_diff <= closest_to_average_diff:
second_closest_height = (height_list[index])
r = 0
for index in difference_list_two:
if index == difference_list[0]:
closest_to_average_diff = index
closest_to_average_height = height_list[r]
closest_to_average_name = name_list[r]
r += 1
newR = 0
for index in difference_list_two:
if index == difference_list[1] and name_list[newR] != closest_to_average_name:
second_closest_to_average_diff = index
second_closest_name = name_list[newR]
second_closest_height = height_list[newR]
newR += 1
City = "City"
most_average = "Most_Average"
Height = "Height"
next_most = "Second_Most_Average"
Next_height = "Height"print(f"{City:^15} {most_average:^25} {Height:^6} {next_most:^25} {Next_height:^6}")
print(f'{city:^15} {closest_to_average_name:^25} {closest_to_average_height:^6} '
f'{second_closest_name:^25} {second_closest_height:^6}')
print(city, avg, difference_list)
print(height_list)
I am trying to do something similar with the code below. I'm trying to find the average height of each city, but it only prints out one average. Can someone tell me where the problem is?
import csv
csv_data = []
with open("C:\\Users\\lucas\\Downloads\\sample-data.csv") as f:
reader = csv.reader(f)
for row in csv.reader(f):
csv_data .append(list(row))
f.close()
csv_data.pop(0)
difference_list = []
def Sorting_cities(data):
cities = []
for i in range(len(data)):
cities.append(data[i][1])
cities.sort()
return cities
city_list = Sorting_cities(csv_data)
print(city_list)
def Listing_cities(cities):
single_cities = []
for city in cities:
if city not in single_cities:
single_cities.append(city)
return single_cities
unique_cities = Listing_cities(city_list)
print(unique_cities)
def Counting_cities_and_people(single_cities, cities):
people_count = []
for city in single_cities:
count = cities.count(city)
people_count.append(count)
for k in range(len(single_cities)):
print(f'City: {single_cities[k]}, Population: {people_count[k]}')
return people_count
population = Counting_cities_and_people(unique_cities, city_list)
print(population)
def Find_heights(data):
heights = []
for city in unique_cities:
for d in data:
if city == d[1]:
heights.append(float(d[2]))
return heights
height_list = Find_heights(csv_data)
print(height_list)
def Find_average(heights):
local_averages = []
average = sum(heights) / len(heights)
for average in heights:
local_averages.append(average)
return average
avg = Find_average(height_list)
print(avg)
def Find_names(data):
names = []
for city in unique_cities:
for d in data:
if city == d[1]:
names.append(d[0])
return names
name_list = Find_names(csv_data)
print(name_list)
def Find_indexes(unique_city, data_set):
index_list = []
index = 0
for row in data_set:
if row[1] == unique_city:
index_list.append(index)
index += 1
return index_list
indexes = Find_indexes(unique_cities, csv_data)
def Find_difference():
for height in height_list:
diff = abs(height - avg)
difference_list.append(diff)
index = 0
closest_to_average_height = 1000000000
closest_to_average_name = " "
second_closest_height = 1000000000
second_closest_name = "placeholder"
difference_list_two = difference_list.copy()
closest_to_average_diff = 1000000000
second_closest_to_average_diff = closest_to_average_diff
difference_list.sort()
for index in range(len(height_list)):
current_diff = difference_list_two[index]
if current_diff <= closest_to_average_diff:
second_closest_height = (height_list[index])
r = 0
for index in difference_list_two:
if index == difference_list[0]:
closest_to_average_diff = index
closest_to_average_height = height_list[r]
closest_to_average_name = name_list[r]
r += 1
newR = 0
for index in difference_list_two:
if index == difference_list[1] and name_list[newR] != closest_to_average_name:
second_closest_to_average_diff = index
second_closest_name = name_list[newR]
second_closest_height = height_list[newR]
newR += 1
City = "City"
most_average = "Most_Average"
Height = "Height"
next_most = "Second_Most_Average"
Next_height = "Height"
print(f"{City:^15} {most_average:^25} {Height:^6} {next_most:^25} {Next_height:^6}")
print(f'{city:^15} {closest_to_average_name:^25} {closest_to_average_height:^6} '
f'{second_closest_name:^25} {second_closest_height:^6}')
print(city, avg, difference_list)
print(height_list)
How could the
- Lambda
- Complicated for loops
- Idx
- Dictionaries
How could this program be done without the lambda function or without "for loops" as complicated as the following?
for city in city_data:
heights = city_data[city]
avg_height = sum(heights) / len(heights)
diff_list = [abs(height - avg_height) for height in heights]
city_diff[city] = diff_list
Also, the program needs to find two people from each city.
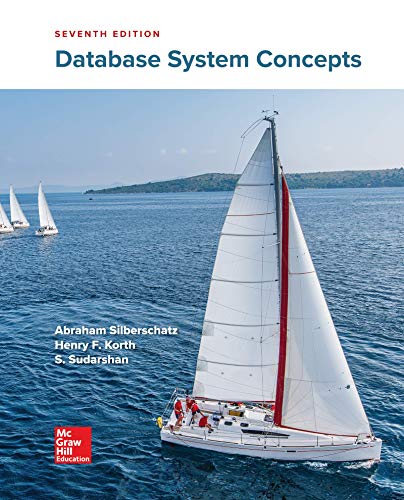
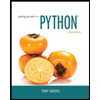
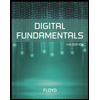
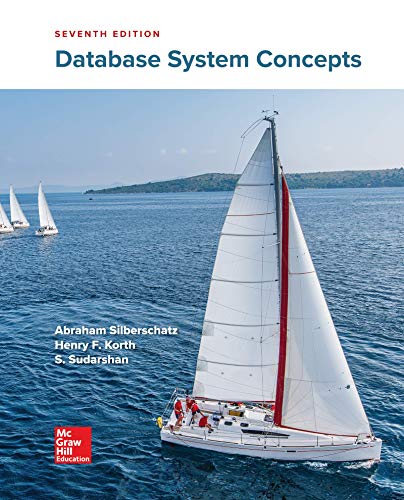
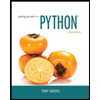
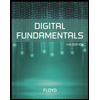
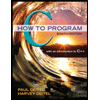
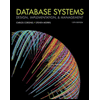
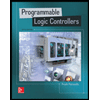