he image as attached is my question, I already have the answer with red text. Also I implement a python code for this question as follow: *Important Note Please write a program or modify my code to output the result like the red text in the images, thank you. # import the libraries import numpy as np import matplotlib.pyplot as plt import pandas as pd import sklearn from sklearn import preprocessing from sklearn.preprocessing import LabelEncoder from sklearn.naive_bayes import GaussianNB #0.2 Assigning features and label variables ID = ['1234', '6754', '2345','2457','3567','7532','1456','1468','8989','9876'] Temp = ['H','H','N','H','N','N','N','H','H','H'] BP = ['H','N','H','H','H','H','N','N','N','N'] Test1 = ['P','N','P','P','P','N','N','N','N','N'] Test2 = ['N','P','P','N','P','N','N','N','P','N'] Health = ['D','D','D','D','D','H','H','H','H','H'] #0.3 Creating labelEncoder and Converting string labels into numbers. le = preprocessing.LabelEncoder() Temp_encoded=le.fit_transform(Temp) print("The encoded temp values are: {}".format(Temp_encoded)) BP_encoded=le.fit_transform(BP) print("The encoded BP values are: {}".format(BP_encoded)) Test1_encoded=le.fit_transform(Test1) print("The encoded Test1 values are: {}".format(Test1_encoded)) Test2_encoded=le.fit_transform(Test2) print("The encoded Test2 values are: {}".format(Test2_encoded)) Health_encoded=le.fit_transform(Health) print("The encoded Health values are: {}".format(Health_encoded)) #0.3.1 Now combine all the features in a single variable (list of tuples). features=zip(Temp_encoded,BP_encoded,Test1_encoded,Test2_encoded) features = list(features) print(features) #0.4 Create a Naive Bayesian Classifier and train the model using the training sets model = GaussianNB() model.fit(features,Health_encoded) #0.5 Predict the output for the new patient: 0: Diseased and 1: Healthy # a) giving value of each term in our NB classifier predicted= model.predict([[0,0,0,1]]) # b) Our Prediction print("Predicted Value:", predicted) #0.6 So our prediction shows the new patient’s health as Healthy (H).
The image as attached is my question, I already have the answer with red text.
Also I implement a python code for this question as follow:
*Important Note
Please write a program or modify my code to output the result like the red text in the images, thank you.
# import the libraries
import numpy as np
import matplotlib.pyplot as plt
import pandas as pd
import sklearn
from sklearn import preprocessing
from sklearn.preprocessing import LabelEncoder
from sklearn.naive_bayes import GaussianNB
#0.2 Assigning features and label variables
ID = ['1234', '6754', '2345','2457','3567','7532','1456','1468','8989','9876']
Temp = ['H','H','N','H','N','N','N','H','H','H']
BP = ['H','N','H','H','H','H','N','N','N','N']
Test1 = ['P','N','P','P','P','N','N','N','N','N']
Test2 = ['N','P','P','N','P','N','N','N','P','N']
Health = ['D','D','D','D','D','H','H','H','H','H']
#0.3 Creating labelEncoder and Converting string labels into numbers.
le = preprocessing.LabelEncoder()
Temp_encoded=le.fit_transform(Temp)
print("The encoded temp values are: {}".format(Temp_encoded))
BP_encoded=le.fit_transform(BP)
print("The encoded BP values are: {}".format(BP_encoded))
Test1_encoded=le.fit_transform(Test1)
print("The encoded Test1 values are: {}".format(Test1_encoded))
Test2_encoded=le.fit_transform(Test2)
print("The encoded Test2 values are: {}".format(Test2_encoded))
Health_encoded=le.fit_transform(Health)
print("The encoded Health values are: {}".format(Health_encoded))
#0.3.1 Now combine all the features in a single variable (list of tuples).
features=zip(Temp_encoded,BP_encoded,Test1_encoded,Test2_encoded)
features = list(features)
print(features)
#0.4 Create a Naive Bayesian Classifier and train the model using the training sets
model = GaussianNB()
model.fit(features,Health_encoded)
#0.5 Predict the output for the new patient: 0: Diseased and 1: Healthy
# a) giving value of each term in our NB classifier
predicted= model.predict([[0,0,0,1]])
# b) Our Prediction
print("Predicted Value:", predicted)
#0.6 So our prediction shows the new patient’s health as Healthy (H).


Step by step
Solved in 2 steps with 2 images

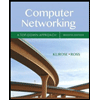
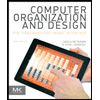
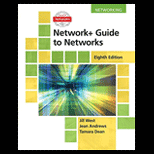
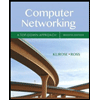
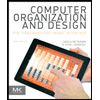
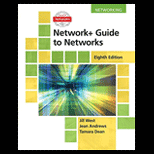
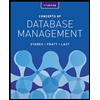
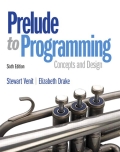
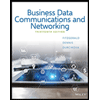