Challenge: Improve Your Spam Classifier [Ungraded] You can improve your classifier in two ways: Feature Extraction: Modify the function extract_features_challenge(). This function takes in a email (list of lines in an email) and a feature dimension B, and should output a feature vector of dimension B. The autograder will pass in both arguments. We provide naive feature extraction from above as an example. Model Training: Modify the function train_spam_filter_challenge(). This function takes in training data xTr, yTr and should output a weight vector w and bias term b for classification. The predictions will be calculated exactly the same way as we have demonstrated in the previous cell. We provide an initial implementation using gradient descent and logistic regression. Your model will be trained on the same training set above (loaded by load_spam_data()), but we will test its accuracy on a testing dataset of emails (hidden from you) feature_dimension = 512 def extract_features_challenge(email, B=feature_dimension): ''' Returns a vector representation for email. The vector is of length B. Input: email: list of lines in an email B: number of dimensions of output vector Output: B-dimensional vector ''' # initialize all-zeros feature vector v = np.zeros(B) email = ' '.join(email) # breaks for non-ascii characters tokens = email.split() for token in tokens: v[hash(token) % B] = 1 # YOUR CODE HERE return v ------- def train_spam_filter_challenge(xTr, yTr): ''' Train a model on training data xTr and labels yTr, and return weight vector and bias term. Input: xTr: data matrix of shape nxd yTr: n-dimensional vector data labels (+1 or -1) Output: w, b w: d-dimension weight vector b: scalar bias term ''' n, d = xTr.shape max_iter = 100 alpha = 1e-5 w, b, losses = logistic_regression_grader(xTr, yTr, max_iter, alpha) # YOUR CODE HERE return w, b ------ def challenge_selftest(): xTr, yTr, cTr = load_spam_data(extract_features_challenge, feature_dimension, train_url) w, b = train_spam_filter_challenge(xTr, yTr) xTe, yTe, cTe = load_spam_data(extract_features_challenge, feature_dimension, test_url) scoresTe = sigmoid_grader(xTe @ w + b) preds = (scoresTe > 0.5).astype(int) preds[preds != 1] = -1 pos_ind = (yTe == 1) neg_ind = (yTe == -1) pos_acc = np.mean(yTe[pos_ind] == preds[pos_ind]) neg_acc = np.mean(yTe[neg_ind] == preds[neg_ind]) test_accuracy = 0.5*pos_acc + 0.5*neg_acc scoresTr = sigmoid_grader(xTr @ w + b) preds_Tr = (scoresTr > 0.5).astype(int) preds_Tr[preds_Tr != 1] = -1 training_accuracy = np.mean(preds_Tr == yTr) return training_accuracy, test_accuracy training_acc, test_acc = challenge_selftest() print("Your features and model achieved training accuracy: {:.2f}% and test accuracy: {:.2f}%".format(training_acc*100, test_acc*100))
Challenge: Improve Your Spam Classifier [Ungraded] You can improve your classifier in two ways: Feature Extraction: Modify the function extract_features_challenge(). This function takes in a email (list of lines in an email) and a feature dimension B, and should output a feature
feature_dimension = 512
def extract_features_challenge(email, B=feature_dimension):
'''
Returns a vector representation for email. The vector is of length B.
Input:
email: list of lines in an email
B: number of dimensions of output vector
Output:
B-dimensional vector
'''
# initialize all-zeros feature vector
v = np.zeros(B)
email = ' '.join(email)
# breaks for non-ascii characters
tokens = email.split()
for token in tokens:
v[hash(token) % B] = 1
# YOUR CODE HERE
return v
-------
def train_spam_filter_challenge(xTr, yTr):
'''
Train a model on training data xTr and labels yTr, and return weight vector and bias term.
Input:
xTr: data matrix of shape nxd
yTr: n-dimensional vector data labels (+1 or -1)
Output:
w, b
w: d-dimension weight vector
b: scalar bias term
'''
n, d = xTr.shape
max_iter = 100
alpha = 1e-5
w, b, losses = logistic_regression_grader(xTr, yTr, max_iter, alpha)
# YOUR CODE HERE
return w, b
------
def challenge_selftest():
xTr, yTr, cTr = load_spam_data(extract_features_challenge, feature_dimension, train_url)
w, b = train_spam_filter_challenge(xTr, yTr)
xTe, yTe, cTe = load_spam_data(extract_features_challenge, feature_dimension, test_url)
scoresTe = sigmoid_grader(xTe @ w + b)
preds = (scoresTe > 0.5).astype(int)
preds[preds != 1] = -1
pos_ind = (yTe == 1)
neg_ind = (yTe == -1)
pos_acc = np.mean(yTe[pos_ind] == preds[pos_ind])
neg_acc = np.mean(yTe[neg_ind] == preds[neg_ind])
test_accuracy = 0.5*pos_acc + 0.5*neg_acc
scoresTr = sigmoid_grader(xTr @ w + b)
preds_Tr = (scoresTr > 0.5).astype(int)
preds_Tr[preds_Tr != 1] = -1
training_accuracy = np.mean(preds_Tr == yTr)
return training_accuracy, test_accuracy
training_acc, test_acc = challenge_selftest()
print("Your features and model achieved training accuracy: {:.2f}% and test accuracy: {:.2f}%".format(training_acc*100, test_acc*100))

Trending now
This is a popular solution!
Step by step
Solved in 3 steps

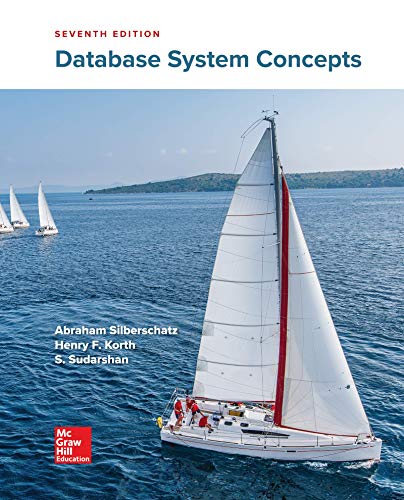
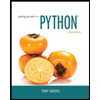
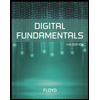
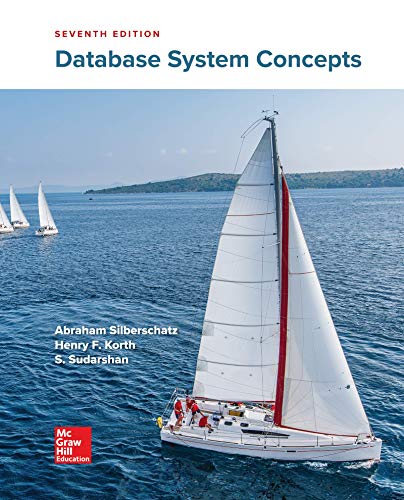
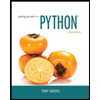
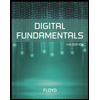
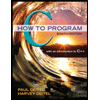
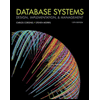
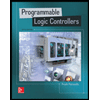