AutoSave off Project Proposal.docx v Design Layout References Mailings Review View Help Search Foxit PDF File Home Insert Draw X Impact (Body) 18 A A Aa AE Paste BIU x² A L A v Clipboard Paragraph Elena Zdravkoska EZ Comments Editing Share 8 Find Normal Strong EVENT HEven Replace Dictate Editor Add-ins Styles Select Editing Editor Add-ins PROJECT PROPOSAL Dataset: https://www.kaggle.com/datasets/mrsimple07/car-prices- prediction-data Project description: I chose this dataset so that I would be able to work on developing a predictive model for car prices estimation. I will be able to achieve developing the model by analyzing the features like make, model, mileage, condition so in the end that same model will predict the car's price. Approach: For my project, I plan to use linear regression and neural networks. I chose these two algorithms because in my opinion they will work the best for the project. Considering that linear regression is simple but effective for prediction of continuous values for example the car prices and it will provide me results that will be easy to understand, I will use it as the baseline model. On the other hand, the neural networks will help me, particularly the deep learning models to handle the complex patterns in the data which will help me with improving prediction accuracy. I will start with preprocessing the data, then I will split the dataset into training and testing sets. Next is training of both models, the linear regression as baseline and the neural networks to capture the complex patterns. After training them I will compare their performance. In the end I will choose the better model and try to improve it by deploying it with new and unseen data. I will be working in Python. Goal: Page 1 of 2 256 words English (United States) Accessibility: Investigate 76°F Search Partly cloudy My goal is to predict effectively car prices. I aim to achieve this by optimizing the algorithms that I chose and fine-tuning the model parameters. Focus ENG 12:52 PM 8/5/2024 50%
Here the code for the project that i have that i have addapted to the proposal that i made. I need to improve the project im the next areas Model Implementation: and Add Hyperparameter Tuning-small change like changimg the parameters. If ypu have any suggestions to make this code be better please do help me. I am a begginer and this is coded in python on kaggle. Pleeeeease help me imporve it, but very simply bc i am an ultimate begginer. Thabks. I am attaching rhe project proposal for you too see whats tbe guide.
!pip install numpy pandas matplotlib scikit-learn tensorflow
import pandas as pd
import numpy as np
import matplotlib.pyplot as plt
from sklearn.model_selection import train_test_split
from sklearn.linear_model import LinearRegression
from sklearn.metrics import mean_squared_error
from sklearn.preprocessing import StandardScaler
from tensorflow.keras.models import Sequential
from tensorflow.keras.layers import Dense
# Load the dataset
url = "https://www.kaggle.com/datasets/mrsimple07/car-prices-prediction-data"
data = pd.read_csv(url)
# Display the first few rows of the dataset
print(data.head())
# Drop rows with missing values (simple approach)
data = data.dropna()
# Convert categorical features to numeric (one-hot encoding)
data = pd.get_dummies(data)
# Separate features and target variable
X = data.drop('price', axis=1)
y = data['price']
# Split the dataset into training and testing sets
X_train, X_test, y_train, y_test = train_test_split(X, y, test_size=0.2, random_state=42)
# Initialize and train the model
lr_model = LinearRegression()
lr_model.fit(X_train, y_train)
# Make predictions
y_pred_lr = lr_model.predict(X_test)
# Evaluate the model
mse_lr = mean_squared_error(y_test, y_pred_lr)
print(f"Linear Regression Mean Squared Error: {mse_lr}")
# Plot actual vs predicted prices
plt.scatter(y_test, y_pred_lr)
plt.xlabel('Actual Prices')
plt.ylabel('Predicted Prices')
plt.title('Linear Regression: Actual vs Predicted Prices')
plt.show()
# Normalize the data
scaler = StandardScaler()
X_train_scaled = scaler.fit_transform(X_train)
X_test_scaled = scaler.transform(X_test)
# Build the neural network model
nn_model = Sequential()
nn_model.add(Dense(64, input_dim=X_train_scaled.shape[1], activation='relu'))
nn_model.add(Dense(32, activation='relu'))
nn_model.add(Dense(1, activation='linear'))
# Compile the model
nn_model.compile(optimizer='adam', loss='mean_squared_error')
# Train the model
history = nn_model.fit(X_train_scaled, y_train, epochs=50, validation_split=0.2, verbose=1)
# Make predictions
y_pred_nn = nn_model.predict(X_test_scaled)
# Evaluate the model
mse_nn = mean_squared_error(y_test, y_pred_nn)
print(f"Neural Network Mean Squared Error: {mse_nn}")
# Plot training loss
plt.plot(history.history['loss'], label='Training Loss')
plt.plot(history.history['val_loss'], label='Validation Loss')
plt.xlabel('Epochs')
plt.ylabel('Loss')
plt.title('Neural Network Training Loss')
plt.legend()
plt.show()
print(f"Linear Regression Mean Squared Error: {mse_lr}")
print(f"Neural Network Mean Squared Error: {mse_nn}")


Step by step
Solved in 2 steps with 4 images

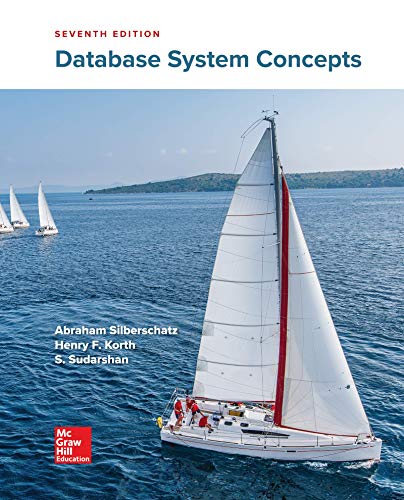
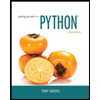
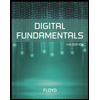
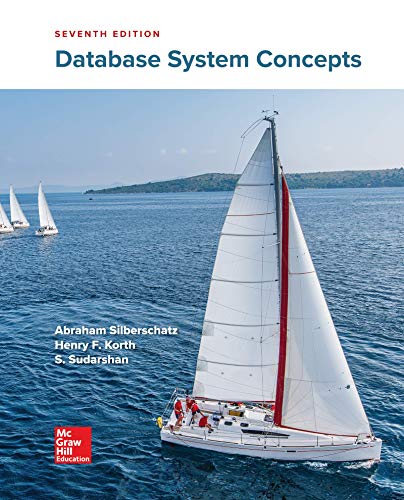
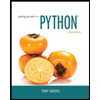
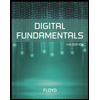
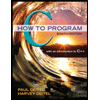
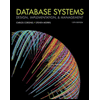
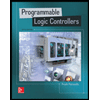