16. Residuals and significance testing with a regression equation Experienced observers use aerial survey methods to estimate the number of snow geese in their summer range area west of Hudson Bay, Canada. A small aircraft flies over the range, and when a flock of geese is spotted, the observer estimates the number of geese in the flock. To investigate the reliability of the estimates, an airplane carrying two goose observers flies over 45 flocks. Each observer makes an independent estimate of the number of geese in each flock. A photograph is taken of each flock and a count made of the number of geese in the photograph. The sample data for the 45 flocks appear in the DataView tool. [Data source: These data were obtained from Lunneborg, C. E. (1994). Modeling experimental and observational data. Pacific Grove, CA: Duxbury Press.] Data SetGeese Sample Variables = 3 Observations = 45 Geese Flock ↓ Photo ↓ A Estimate ↓ B Estimate ↓ 1 62 40 50 2 26 30 20 3 88 75 120 4 56 35 60 5 11 9 10 6 66 55 80 7 42 30 35 8 30 25 30 9 90 40 120 10 119 75 200 11 165 100 200 12 152 150 150 13 205 120 200 14 409 250 300 15 342 500 500 16 200 200 300 17 73 50 40 18 123 75 80 19 150 150 120 20 70 50 60 21 90 60 100 22 110 75 120 23 95 150 150 24 57 40 40 25 43 25 35 26 55 100 110 27 325 200 400 28 114 60 120 29 83 40 40 30 91 35 60 31 56 20 40 32 56 50 40 33 38 25 30 34 25 30 40 35 48 35 45 36 38 25 30 37 22 20 20 38 22 12 20 39 42 34 35 40 34 20 30 41 14 10 12 42 30 25 30 43 9 10 10 44 18 15 18 45 25 20 30 Flock ↓ Photo ↓ A Estimate ↓ B Estimate ↓ 1 62 40 50 2 26 30 20 3 88 75 120 4 56 35 60 5 11 9 10 6 66 55 80 7 42 30 35 8 30 25 30 9 90 40 120 10 119 75 200 11 165 100 200 12 152 150 150 13 205 120 200 14 409 250 300 15 342 500 500 16 200 200 300 17 73 50 40 18 123 75 80 19 150 150 120 20 70 50 60 21 90 60 100 22 110 75 120 23 95 150 150 24 57 40 40 25 43 25 35 26 55 100 110 27 325 200 400 28 114 60 120 29 83 40 40 30 91 35 60 31 56 20 40 32 56 50 40 33 38 25 30 34 25 30 40 35 48 35 45 36 38 25 30 37 22 20 20 38 22 12 20 39 42 34 35 40 34 20 30 41 14 10 12 42 30 25 30 43 9 10 10 44 18 15 18 45 25 20 30 Flock ↓ Photo ↓ A Estimate ↓ B Estimate ↓ 1 62 40 50 2 26 30 20 3 88 75 120 4 56 35 60 5 11 9 10 6 66 55 80 7 42 30 35 8 30 25 30 9 90 40 120 10 119 75 200 11 165 100 200 12 152 150 150 13 205 120 200 14 409 250 300 15 342 500 500 16 200 200 300 17 73 50 40 18 123 75 80 19 150 150 120 20 70 50 60 21 90 60 100 22 110 75 120 23 95 150 150 24 57 40 40 25 43 25 35 26 55 100 110 27 325 200 400 28 114 60 120 29 83 40 40 30 91 35 60 31 56 20 40 32 56 50 40 33 38 25 30 34 25 30 40 35 48 35 45 36 38 25 30 37 22 20 20 38 22 12 20 39 42 34 35 40 34 20 30 41 14 10 12 42 30 25 30 43 9 10 10 44 18 15 18 45 25 20 30 You will work with goose observer B’s estimates in this problem to examine how well observer B’s estimates predict counts from the associated photographs for the same flock. The photographs provide a highly accurate count of geese; optimally, the observer’s estimate would predict the photo-based count for a specific flock. First, use the regression equation to predict Y values based on observer B’s estimates. The regression equation, in the format Ŷ = bX + a, is: Ŷ = 0.77X + 16.16 where X = goose observer B’s estimate, Ŷ = an estimate of the goose count from the photograph In this problem, Y is the actual count of geese in the photograph. Note: The estimated regression equation can also be obtained by going to the Correlation section in the DataView tool, specifying the proper dependent (Y) and independent (X) variables, and clicking on the Linear Regression button. Find the predicted Y values for the flocks 5, 21, and 44. Ŷ for flock 5 is ? . Ŷ for flock 21 is ? . Ŷ for flock 44 is ? . You will need to use the Observations list in the DataView tool to identify goose observer B’s estimate for the appropriate flock. Click on the Observations button in the tool, and scroll to the appropriate flock number. Calculate the residuals for the flocks identified. The residual for flock 5 is ? . The residual for flock 21 is ? . The residual for flock 44 is ? . You want to test the significance of this regression equation. The null hypothesis can be phrased as: The regression equation accounts for a significant portion of the variance in the y scores (counts from the photos). The regression equation does not account for a significant portion of the variance in the y scores (counts from the photos). The slope of the regression equation is greater than zero. The intercept of the regression equation is greater than zero. The Pearson correlation is r = 0.9245, SSXX = 490,692.44, and SSYY = 339,559.64. Calculate the predicted variability, the unpredicted variability, and the percentage of the variance explained by the regression equation mentioned previously. The predicted variability is ? . The unpredicted variability is ? . The percentage of the variance explained is 85.47. To test the null hypothesis, you will first need to find the critical value of F at alpha = 0.05. F is ? . Next, calculate the F-ratio. The F-ratio is ? . Therefore, the null hypothesis is ? . On the basis of these results, you ? conclude that the regression equation accounts for a significant portion of the variance in the y scores (counts from the photos)
16. Residuals and significance testing with a regression equation Experienced observers use aerial survey methods to estimate the number of snow geese in their summer range area west of Hudson Bay, Canada. A small aircraft flies over the range, and when a flock of geese is spotted, the observer estimates the number of geese in the flock. To investigate the reliability of the estimates, an airplane carrying two goose observers flies over 45 flocks. Each observer makes an independent estimate of the number of geese in each flock. A photograph is taken of each flock and a count made of the number of geese in the photograph. The sample data for the 45 flocks appear in the DataView tool. [Data source: These data were obtained from Lunneborg, C. E. (1994). Modeling experimental and observational data. Pacific Grove, CA: Duxbury Press.] Data SetGeese Sample Variables = 3 Observations = 45 Geese Flock ↓ Photo ↓ A Estimate ↓ B Estimate ↓ 1 62 40 50 2 26 30 20 3 88 75 120 4 56 35 60 5 11 9 10 6 66 55 80 7 42 30 35 8 30 25 30 9 90 40 120 10 119 75 200 11 165 100 200 12 152 150 150 13 205 120 200 14 409 250 300 15 342 500 500 16 200 200 300 17 73 50 40 18 123 75 80 19 150 150 120 20 70 50 60 21 90 60 100 22 110 75 120 23 95 150 150 24 57 40 40 25 43 25 35 26 55 100 110 27 325 200 400 28 114 60 120 29 83 40 40 30 91 35 60 31 56 20 40 32 56 50 40 33 38 25 30 34 25 30 40 35 48 35 45 36 38 25 30 37 22 20 20 38 22 12 20 39 42 34 35 40 34 20 30 41 14 10 12 42 30 25 30 43 9 10 10 44 18 15 18 45 25 20 30 Flock ↓ Photo ↓ A Estimate ↓ B Estimate ↓ 1 62 40 50 2 26 30 20 3 88 75 120 4 56 35 60 5 11 9 10 6 66 55 80 7 42 30 35 8 30 25 30 9 90 40 120 10 119 75 200 11 165 100 200 12 152 150 150 13 205 120 200 14 409 250 300 15 342 500 500 16 200 200 300 17 73 50 40 18 123 75 80 19 150 150 120 20 70 50 60 21 90 60 100 22 110 75 120 23 95 150 150 24 57 40 40 25 43 25 35 26 55 100 110 27 325 200 400 28 114 60 120 29 83 40 40 30 91 35 60 31 56 20 40 32 56 50 40 33 38 25 30 34 25 30 40 35 48 35 45 36 38 25 30 37 22 20 20 38 22 12 20 39 42 34 35 40 34 20 30 41 14 10 12 42 30 25 30 43 9 10 10 44 18 15 18 45 25 20 30 Flock ↓ Photo ↓ A Estimate ↓ B Estimate ↓ 1 62 40 50 2 26 30 20 3 88 75 120 4 56 35 60 5 11 9 10 6 66 55 80 7 42 30 35 8 30 25 30 9 90 40 120 10 119 75 200 11 165 100 200 12 152 150 150 13 205 120 200 14 409 250 300 15 342 500 500 16 200 200 300 17 73 50 40 18 123 75 80 19 150 150 120 20 70 50 60 21 90 60 100 22 110 75 120 23 95 150 150 24 57 40 40 25 43 25 35 26 55 100 110 27 325 200 400 28 114 60 120 29 83 40 40 30 91 35 60 31 56 20 40 32 56 50 40 33 38 25 30 34 25 30 40 35 48 35 45 36 38 25 30 37 22 20 20 38 22 12 20 39 42 34 35 40 34 20 30 41 14 10 12 42 30 25 30 43 9 10 10 44 18 15 18 45 25 20 30 You will work with goose observer B’s estimates in this problem to examine how well observer B’s estimates predict counts from the associated photographs for the same flock. The photographs provide a highly accurate count of geese; optimally, the observer’s estimate would predict the photo-based count for a specific flock. First, use the regression equation to predict Y values based on observer B’s estimates. The regression equation, in the format Ŷ = bX + a, is: Ŷ = 0.77X + 16.16 where X = goose observer B’s estimate, Ŷ = an estimate of the goose count from the photograph In this problem, Y is the actual count of geese in the photograph. Note: The estimated regression equation can also be obtained by going to the Correlation section in the DataView tool, specifying the proper dependent (Y) and independent (X) variables, and clicking on the Linear Regression button. Find the predicted Y values for the flocks 5, 21, and 44. Ŷ for flock 5 is ? . Ŷ for flock 21 is ? . Ŷ for flock 44 is ? . You will need to use the Observations list in the DataView tool to identify goose observer B’s estimate for the appropriate flock. Click on the Observations button in the tool, and scroll to the appropriate flock number. Calculate the residuals for the flocks identified. The residual for flock 5 is ? . The residual for flock 21 is ? . The residual for flock 44 is ? . You want to test the significance of this regression equation. The null hypothesis can be phrased as: The regression equation accounts for a significant portion of the variance in the y scores (counts from the photos). The regression equation does not account for a significant portion of the variance in the y scores (counts from the photos). The slope of the regression equation is greater than zero. The intercept of the regression equation is greater than zero. The Pearson correlation is r = 0.9245, SSXX = 490,692.44, and SSYY = 339,559.64. Calculate the predicted variability, the unpredicted variability, and the percentage of the variance explained by the regression equation mentioned previously. The predicted variability is ? . The unpredicted variability is ? . The percentage of the variance explained is 85.47. To test the null hypothesis, you will first need to find the critical value of F at alpha = 0.05. F is ? . Next, calculate the F-ratio. The F-ratio is ? . Therefore, the null hypothesis is ? . On the basis of these results, you ? conclude that the regression equation accounts for a significant portion of the variance in the y scores (counts from the photos)
MATLAB: An Introduction with Applications
6th Edition
ISBN:9781119256830
Author:Amos Gilat
Publisher:Amos Gilat
Chapter1: Starting With Matlab
Section: Chapter Questions
Problem 1P
Related questions
Question
16. Residuals and significance testing with a regression equation
Experienced observers use aerial survey methods to estimate the number of snow geese in their summer range area west of Hudson Bay, Canada. A small aircraft flies over the range, and when a flock of geese is spotted, the observer estimates the number of geese in the flock.
To investigate the reliability of the estimates, an airplane carrying two goose observers flies over 45 flocks. Each observer makes an independent estimate of the number of geese in each flock. A photograph is taken of each flock and a count made of the number of geese in the photograph. The sample data for the 45 flocks appear in the DataView tool. [Data source: These data were obtained from Lunneborg, C. E. (1994). Modeling experimental and observational data. Pacific Grove, CA: Duxbury Press.]
Data SetGeese
Sample
Variables = 3
Observations = 45
Geese
Flock ↓ |
Photo ↓ |
A Estimate ↓ |
B Estimate ↓ |
---|---|---|---|
1 | 62 | 40 | 50 |
2 | 26 | 30 | 20 |
3 | 88 | 75 | 120 |
4 | 56 | 35 | 60 |
5 | 11 | 9 | 10 |
6 | 66 | 55 | 80 |
7 | 42 | 30 | 35 |
8 | 30 | 25 | 30 |
9 | 90 | 40 | 120 |
10 | 119 | 75 | 200 |
11 | 165 | 100 | 200 |
12 | 152 | 150 | 150 |
13 | 205 | 120 | 200 |
14 | 409 | 250 | 300 |
15 | 342 | 500 | 500 |
16 | 200 | 200 | 300 |
17 | 73 | 50 | 40 |
18 | 123 | 75 | 80 |
19 | 150 | 150 | 120 |
20 | 70 | 50 | 60 |
21 | 90 | 60 | 100 |
22 | 110 | 75 | 120 |
23 | 95 | 150 | 150 |
24 | 57 | 40 | 40 |
25 | 43 | 25 | 35 |
26 | 55 | 100 | 110 |
27 | 325 | 200 | 400 |
28 | 114 | 60 | 120 |
29 | 83 | 40 | 40 |
30 | 91 | 35 | 60 |
31 | 56 | 20 | 40 |
32 | 56 | 50 | 40 |
33 | 38 | 25 | 30 |
34 | 25 | 30 | 40 |
35 | 48 | 35 | 45 |
36 | 38 | 25 | 30 |
37 | 22 | 20 | 20 |
38 | 22 | 12 | 20 |
39 | 42 | 34 | 35 |
40 | 34 | 20 | 30 |
41 | 14 | 10 | 12 |
42 | 30 | 25 | 30 |
43 | 9 | 10 | 10 |
44 | 18 | 15 | 18 |
45 | 25 | 20 | 30 |
Flock ↓ |
Photo ↓ |
A Estimate ↓ |
B Estimate ↓ |
---|---|---|---|
1 | 62 | 40 | 50 |
2 | 26 | 30 | 20 |
3 | 88 | 75 | 120 |
4 | 56 | 35 | 60 |
5 | 11 | 9 | 10 |
6 | 66 | 55 | 80 |
7 | 42 | 30 | 35 |
8 | 30 | 25 | 30 |
9 | 90 | 40 | 120 |
10 | 119 | 75 | 200 |
11 | 165 | 100 | 200 |
12 | 152 | 150 | 150 |
13 | 205 | 120 | 200 |
14 | 409 | 250 | 300 |
15 | 342 | 500 | 500 |
16 | 200 | 200 | 300 |
17 | 73 | 50 | 40 |
18 | 123 | 75 | 80 |
19 | 150 | 150 | 120 |
20 | 70 | 50 | 60 |
21 | 90 | 60 | 100 |
22 | 110 | 75 | 120 |
23 | 95 | 150 | 150 |
24 | 57 | 40 | 40 |
25 | 43 | 25 | 35 |
26 | 55 | 100 | 110 |
27 | 325 | 200 | 400 |
28 | 114 | 60 | 120 |
29 | 83 | 40 | 40 |
30 | 91 | 35 | 60 |
31 | 56 | 20 | 40 |
32 | 56 | 50 | 40 |
33 | 38 | 25 | 30 |
34 | 25 | 30 | 40 |
35 | 48 | 35 | 45 |
36 | 38 | 25 | 30 |
37 | 22 | 20 | 20 |
38 | 22 | 12 | 20 |
39 | 42 | 34 | 35 |
40 | 34 | 20 | 30 |
41 | 14 | 10 | 12 |
42 | 30 | 25 | 30 |
43 | 9 | 10 | 10 |
44 | 18 | 15 | 18 |
45 | 25 | 20 | 30 |
Flock ↓ |
Photo ↓ |
A Estimate ↓ |
B Estimate ↓ |
---|---|---|---|
1 | 62 | 40 | 50 |
2 | 26 | 30 | 20 |
3 | 88 | 75 | 120 |
4 | 56 | 35 | 60 |
5 | 11 | 9 | 10 |
6 | 66 | 55 | 80 |
7 | 42 | 30 | 35 |
8 | 30 | 25 | 30 |
9 | 90 | 40 | 120 |
10 | 119 | 75 | 200 |
11 | 165 | 100 | 200 |
12 | 152 | 150 | 150 |
13 | 205 | 120 | 200 |
14 | 409 | 250 | 300 |
15 | 342 | 500 | 500 |
16 | 200 | 200 | 300 |
17 | 73 | 50 | 40 |
18 | 123 | 75 | 80 |
19 | 150 | 150 | 120 |
20 | 70 | 50 | 60 |
21 | 90 | 60 | 100 |
22 | 110 | 75 | 120 |
23 | 95 | 150 | 150 |
24 | 57 | 40 | 40 |
25 | 43 | 25 | 35 |
26 | 55 | 100 | 110 |
27 | 325 | 200 | 400 |
28 | 114 | 60 | 120 |
29 | 83 | 40 | 40 |
30 | 91 | 35 | 60 |
31 | 56 | 20 | 40 |
32 | 56 | 50 | 40 |
33 | 38 | 25 | 30 |
34 | 25 | 30 | 40 |
35 | 48 | 35 | 45 |
36 | 38 | 25 | 30 |
37 | 22 | 20 | 20 |
38 | 22 | 12 | 20 |
39 | 42 | 34 | 35 |
40 | 34 | 20 | 30 |
41 | 14 | 10 | 12 |
42 | 30 | 25 | 30 |
43 | 9 | 10 | 10 |
44 | 18 | 15 | 18 |
45 | 25 | 20 | 30 |
You will work with goose observer B’s estimates in this problem to examine how well observer B’s estimates predict counts from the associated photographs for the same flock. The photographs provide a highly accurate count of geese; optimally, the observer’s estimate would predict the photo-based count for a specific flock.
First, use the regression equation to predict Y values based on observer B’s estimates. The regression equation, in the format Ŷ = bX + a, is:
Ŷ = 0.77X + 16.16
where X = goose observer B’s estimate,
Ŷ = an estimate of the goose count from the photograph
In this problem, Y is the actual count of geese in the photograph.
Note: The estimated regression equation can also be obtained by going to the Correlation section in the DataView tool, specifying the proper dependent (Y) and independent (X) variables, and clicking on the Linear Regression button.
Find the predicted Y values for the flocks 5, 21, and 44. Ŷ for flock 5 is ? . Ŷ for flock 21 is ? . Ŷ for flock 44 is ? . You will need to use the Observations list in the DataView tool to identify goose observer B’s estimate for the appropriate flock. Click on the Observations button in the tool, and scroll to the appropriate flock number.
Calculate the residuals for the flocks identified. The residual for flock 5 is ? . The residual for flock 21 is ? . The residual for flock 44 is ? .
You want to test the significance of this regression equation. The null hypothesis can be phrased as:
The regression equation accounts for a significant portion of the variance in the y scores (counts from the photos).
The regression equation does not account for a significant portion of the variance in the y scores (counts from the photos).
The slope of the regression equation is greater than zero.
The intercept of the regression equation is greater than zero.
The Pearson correlation is r = 0.9245, SSXX = 490,692.44, and SSYY = 339,559.64. Calculate the predicted variability, the unpredicted variability, and the percentage of the variance explained by the regression equation mentioned previously. The predicted variability is ? . The unpredicted variability is ? . The percentage of the variance explained is 85.47.
To test the null hypothesis, you will first need to find the critical value of F at alpha = 0.05. F is ? . Next, calculate the F-ratio. The F-ratio is ? . Therefore, the null hypothesis is ? . On the basis of these results, you ? conclude that the regression equation accounts for a significant portion of the variance in the y scores (counts from the photos).
Expert Solution

This question has been solved!
Explore an expertly crafted, step-by-step solution for a thorough understanding of key concepts.
This is a popular solution!
Trending now
This is a popular solution!
Step by step
Solved in 4 steps

Recommended textbooks for you
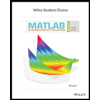
MATLAB: An Introduction with Applications
Statistics
ISBN:
9781119256830
Author:
Amos Gilat
Publisher:
John Wiley & Sons Inc
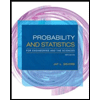
Probability and Statistics for Engineering and th…
Statistics
ISBN:
9781305251809
Author:
Jay L. Devore
Publisher:
Cengage Learning
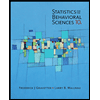
Statistics for The Behavioral Sciences (MindTap C…
Statistics
ISBN:
9781305504912
Author:
Frederick J Gravetter, Larry B. Wallnau
Publisher:
Cengage Learning
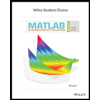
MATLAB: An Introduction with Applications
Statistics
ISBN:
9781119256830
Author:
Amos Gilat
Publisher:
John Wiley & Sons Inc
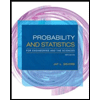
Probability and Statistics for Engineering and th…
Statistics
ISBN:
9781305251809
Author:
Jay L. Devore
Publisher:
Cengage Learning
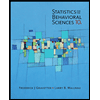
Statistics for The Behavioral Sciences (MindTap C…
Statistics
ISBN:
9781305504912
Author:
Frederick J Gravetter, Larry B. Wallnau
Publisher:
Cengage Learning
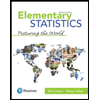
Elementary Statistics: Picturing the World (7th E…
Statistics
ISBN:
9780134683416
Author:
Ron Larson, Betsy Farber
Publisher:
PEARSON
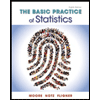
The Basic Practice of Statistics
Statistics
ISBN:
9781319042578
Author:
David S. Moore, William I. Notz, Michael A. Fligner
Publisher:
W. H. Freeman
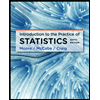
Introduction to the Practice of Statistics
Statistics
ISBN:
9781319013387
Author:
David S. Moore, George P. McCabe, Bruce A. Craig
Publisher:
W. H. Freeman