1. Consider the numerical examples given in Section 8.8 of Chapter 8, involving assess- ment of the relationship of the independent variables HGT, AGE, and (AGE) to the dependent variable WGT. Suppose that HGT is the independent variable of primary concern, so interest lies in evaluating the relationship of HGT to WGT, controlling for the possible confounding effects of AGE and (AGE)². a. Assuming that no interaction of any kind exists, state an appropriate regression model to use as the baseline (i.e., standard) for decisions about confounding. b. Using an appropriate regression coefficient given in part (a) as your measure of association, determine whether confounding exists due to AGE and/or (AGE)². c. Can (AGE) be dropped from your initial model in part (a) because it is not needed to control adequately for confounding? Explain your answer (using a regression coefficient as your measure of association). d. Should (AGE)² be retained in the final model for the sake of precision? Explain. e. In light of both confounding and precision, what should be your final model? Why? f. How would you modify your initial model in part (a) to allow for assessing inter- actions? g. Regarding your answer to part (f), how would you test for interaction?
1. Consider the numerical examples given in Section 8.8 of Chapter 8, involving assess- ment of the relationship of the independent variables HGT, AGE, and (AGE) to the dependent variable WGT. Suppose that HGT is the independent variable of primary concern, so interest lies in evaluating the relationship of HGT to WGT, controlling for the possible confounding effects of AGE and (AGE)². a. Assuming that no interaction of any kind exists, state an appropriate regression model to use as the baseline (i.e., standard) for decisions about confounding. b. Using an appropriate regression coefficient given in part (a) as your measure of association, determine whether confounding exists due to AGE and/or (AGE)². c. Can (AGE) be dropped from your initial model in part (a) because it is not needed to control adequately for confounding? Explain your answer (using a regression coefficient as your measure of association). d. Should (AGE)² be retained in the final model for the sake of precision? Explain. e. In light of both confounding and precision, what should be your final model? Why? f. How would you modify your initial model in part (a) to allow for assessing inter- actions? g. Regarding your answer to part (f), how would you test for interaction?
MATLAB: An Introduction with Applications
6th Edition
ISBN:9781119256830
Author:Amos Gilat
Publisher:Amos Gilat
Chapter1: Starting With Matlab
Section: Chapter Questions
Problem 1P
Related questions
Question

Transcribed Image Text:1. Consider the numerical examples given in Section 8.8 of Chapter 8, involving assessment of the relationship of the independent variables HGT, AGE, and (AGE)² to the dependent variable WGT. Suppose that HGT is the independent variable of primary concern, so interest lies in evaluating the relationship of HGT to WGT, controlling for the possible confounding effects of AGE and (AGE)².
a. Assuming that no interaction of any kind exists, state an appropriate regression model to use as the baseline (i.e., standard) for decisions about confounding.
b. Using an appropriate regression coefficient given in part (a) as your measure of association, determine whether confounding exists due to AGE and/or (AGE)².
c. Can (AGE)² be dropped from your initial model in part (a) because it is not needed to control adequately for confounding? Explain your answer (using a regression coefficient as your measure of association).
d. Should (AGE)² be retained in the final model for the sake of precision? Explain.
e. In light of both confounding and precision, what should be your final model? Why?
f. How would you modify your initial model in part (a) to allow for assessing interactions?
g. Regarding your answer to part (f), how would you test for interaction?

Transcribed Image Text:The image includes multiple regression models of WGT (weight) on various predictors such as HGT (height), AGE, and AGE squared (AGE²). Each model is analyzed using SAS software, and the output is displayed in tables showing statistical results.
### Model Details
**Model 1: WGT = β₀ + β₁HGT + E**
- **Model Summary:**
- Source: Model and Error
- Sum of Squares: Model (989.562312), Error (988.992237), Corrected Total (1978.55455)
- F Statistics: 19.07
- P-value: 0.0003
- **Statistics:**
- R-Square: 0.4989918
- Root MSE: 8.048740
- WGT Mean: 67.75000
- **Parameters:**
- Intercept: Estimate (72.4927036), Standard Error (2.4842008), P-value (0.0001)
- HGT: Estimate (1.0722366), Standard Error (0.2417306), P-value (0.0013)
**Model 2: WGT = β₀ + β₁AGE + E**
- **Model Summary:**
- Source: Model and Error
- Sum of Squares: Model (588.362574), Error (388.387470)
- F Statistics: 14.55
- P-value: 0.0034
- **Statistics:**
- R-Square: 0.526819
- Root MSE: 6.810566
- WGT Mean: 62.75000
- **Parameters:**
- Intercept: Estimate (30.7142657), Standard Error (6.8192306), P-value (0.0015)
- AGE: Estimate (3.6248754), Standard Error (0.9551152), P-value (0.0034)
**Model 3: WGT = β₀ + β₁(AGE)² + E**
- **Model Summary:**
- Source: Model and Error
- Sum of Squares: Model (961.932437), Error (366.372570)
- F Statistics: 14.25
- P-value: 0.003
Expert Solution

This question has been solved!
Explore an expertly crafted, step-by-step solution for a thorough understanding of key concepts.
This is a popular solution!
Trending now
This is a popular solution!
Step by step
Solved in 4 steps with 1 images

Recommended textbooks for you
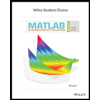
MATLAB: An Introduction with Applications
Statistics
ISBN:
9781119256830
Author:
Amos Gilat
Publisher:
John Wiley & Sons Inc
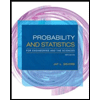
Probability and Statistics for Engineering and th…
Statistics
ISBN:
9781305251809
Author:
Jay L. Devore
Publisher:
Cengage Learning
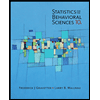
Statistics for The Behavioral Sciences (MindTap C…
Statistics
ISBN:
9781305504912
Author:
Frederick J Gravetter, Larry B. Wallnau
Publisher:
Cengage Learning
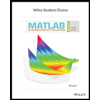
MATLAB: An Introduction with Applications
Statistics
ISBN:
9781119256830
Author:
Amos Gilat
Publisher:
John Wiley & Sons Inc
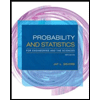
Probability and Statistics for Engineering and th…
Statistics
ISBN:
9781305251809
Author:
Jay L. Devore
Publisher:
Cengage Learning
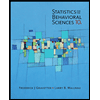
Statistics for The Behavioral Sciences (MindTap C…
Statistics
ISBN:
9781305504912
Author:
Frederick J Gravetter, Larry B. Wallnau
Publisher:
Cengage Learning
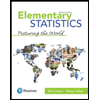
Elementary Statistics: Picturing the World (7th E…
Statistics
ISBN:
9780134683416
Author:
Ron Larson, Betsy Farber
Publisher:
PEARSON
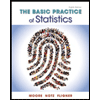
The Basic Practice of Statistics
Statistics
ISBN:
9781319042578
Author:
David S. Moore, William I. Notz, Michael A. Fligner
Publisher:
W. H. Freeman
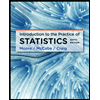
Introduction to the Practice of Statistics
Statistics
ISBN:
9781319013387
Author:
David S. Moore, George P. McCabe, Bruce A. Craig
Publisher:
W. H. Freeman