Suppose the number of seconds required to accelerate to 60 mph depends on both a car’s weight, its horsepower, and an interaction term between the two variables. Describe why an interaction term could be appropriate for the variables in this model and construct three linear relationships between acceleration time and weight, each for a different assumed value of horsepower.
Suppose the number of seconds required to accelerate to 60 mph depends on both a car’s weight, its horsepower, and an interaction term between the two variables. Describe why an interaction term could be appropriate for the variables in this model and construct three linear relationships between acceleration time and weight, each for a different assumed value of horsepower.
MATLAB: An Introduction with Applications
6th Edition
ISBN:9781119256830
Author:Amos Gilat
Publisher:Amos Gilat
Chapter1: Starting With Matlab
Section: Chapter Questions
Problem 1P
Related questions
Question
Suppose the number of seconds required to accelerate to 60 mph depends on both a car’s weight, its horsepower, and an interaction term between the two variables.
Describe why an interaction term could be appropriate for the variables in this model and construct three linear relationships between acceleration time and weight, each for a different assumed value of horsepower.

Transcribed Image Text:### Summary Output
#### Regression Statistics
- **Multiple R:** 0.856730669
- **R Square:** 0.733987439
- **Adjusted R Square:** 0.687044045
- **Standard Error:** 2.967605917
- **Observations:** 21
#### Analysis of Variance (ANOVA)
| | df | SS | MS | F | Significance F |
|----------|----|------------|------------|------------|---------------------|
| Regression | 3 | 413.0930237 | 137.6976746 | 15.63558552 | 3.87427E-05 |
| Residual | 17 | 149.7136429 | 8.806688479 | | |
| Total | 20 | 562.8066667 | | | |
#### Coefficients
| | Coefficients | Standard Error | t Stat | P-value |
|--------------|----------------|----------------|-------------|---------------|
| Intercept | 25.41936066 | 4.338034616 | 5.859649107 | 1.89327E-05 |
| hp | -0.161091493 | 0.035506341 | -4.536978136 | 0.000291759 |
| curbwt | -0.000302296 | 0.002232767 | -0.135390557 | 0.893893295 |
| hp*curbwt | 2.8177E-05 | 9.4596E-06 | 2.978662513 | 0.008429352 |
### Explanation
This output is from a multiple regression analysis, indicating the relationship between a dependent variable and several independent variables.
- **Multiple R** and **R Square** indicate the strength and proportion of variance explained by the model.
- **Adjusted R Square** accounts for the number of predictors in the model.
- **ANOVA** table shows the breakdown of variances, with a significant F-statistic suggesting the model is a good fit.
- **Coefficients** table provides the estimated impact of each predictor, with associated statistical tests. Lower p-values suggest significant contributions to the model.
Expert Solution

This question has been solved!
Explore an expertly crafted, step-by-step solution for a thorough understanding of key concepts.
This is a popular solution!
Trending now
This is a popular solution!
Step by step
Solved in 3 steps

Recommended textbooks for you
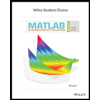
MATLAB: An Introduction with Applications
Statistics
ISBN:
9781119256830
Author:
Amos Gilat
Publisher:
John Wiley & Sons Inc
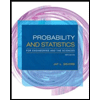
Probability and Statistics for Engineering and th…
Statistics
ISBN:
9781305251809
Author:
Jay L. Devore
Publisher:
Cengage Learning
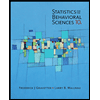
Statistics for The Behavioral Sciences (MindTap C…
Statistics
ISBN:
9781305504912
Author:
Frederick J Gravetter, Larry B. Wallnau
Publisher:
Cengage Learning
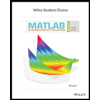
MATLAB: An Introduction with Applications
Statistics
ISBN:
9781119256830
Author:
Amos Gilat
Publisher:
John Wiley & Sons Inc
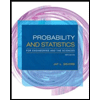
Probability and Statistics for Engineering and th…
Statistics
ISBN:
9781305251809
Author:
Jay L. Devore
Publisher:
Cengage Learning
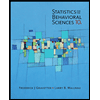
Statistics for The Behavioral Sciences (MindTap C…
Statistics
ISBN:
9781305504912
Author:
Frederick J Gravetter, Larry B. Wallnau
Publisher:
Cengage Learning
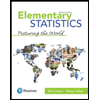
Elementary Statistics: Picturing the World (7th E…
Statistics
ISBN:
9780134683416
Author:
Ron Larson, Betsy Farber
Publisher:
PEARSON
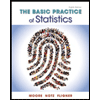
The Basic Practice of Statistics
Statistics
ISBN:
9781319042578
Author:
David S. Moore, William I. Notz, Michael A. Fligner
Publisher:
W. H. Freeman
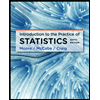
Introduction to the Practice of Statistics
Statistics
ISBN:
9781319013387
Author:
David S. Moore, George P. McCabe, Bruce A. Craig
Publisher:
W. H. Freeman