It contains data on brain mass in different species versus glia-neuron ratio, the latter being a measurement of brain metabolism as the glia provides the metabolic needs of the neurons. The relationship between THE LOGARITHM of the brain mass (in the third column) and Glia-neuron ratio (fourth column) appears linear and it is these two variables that we wish to analyze via linear regression. We would like to know if the human brain fits the trend from the other species. Towards this end we will perform the regression on all species EXCEPT humans
Correlation
Correlation defines a relationship between two independent variables. It tells the degree to which variables move in relation to each other. When two sets of data are related to each other, there is a correlation between them.
Linear Correlation
A correlation is used to determine the relationships between numerical and categorical variables. In other words, it is an indicator of how things are connected to one another. The correlation analysis is the study of how variables are related.
Regression Analysis
Regression analysis is a statistical method in which it estimates the relationship between a dependent variable and one or more independent variable. In simple terms dependent variable is called as outcome variable and independent variable is called as predictors. Regression analysis is one of the methods to find the trends in data. The independent variable used in Regression analysis is named Predictor variable. It offers data of an associated dependent variable regarding a particular outcome.
It contains data on brain mass in different species versus glia-neuron ratio, the latter being a measurement of brain metabolism as the glia provides the metabolic needs of the neurons. The relationship between THE LOGARITHM of the brain mass (in the third column) and Glia-neuron ratio (fourth column) appears linear and it is these two variables that we wish to analyze via linear regression. We would like to know if the human brain fits the trend from the other species. Towards this end we will perform the regression on all species EXCEPT humans (Homo sapiens). Again, throw out the human data from your analysis. You will however need the human numbers for some of the questions. The analysis to be performed is as follows:
1. Calculate the regression line (slope and intercept)
2. Perform an ANOVA test of the null hypothesis for zero slope. From this analysis, obtain SStotal, SSregression and SSresidual as well as the corresponding MS statistics.
3. Perform a t-test of the null hypothesis of zero slope.
4. Compute a 95% confidence interval for the regression slope
5. Calculate the predicted glia-neuron ratio for human brain given the human brain mass. Calculate the two confidence intervals for the predicted glia-neuron ratio corresponding to the mass of the human brain: (i) the confidence interval for the predicted mean and (ii) the confidence interval for a predicted individual.
Once again: You need the human data only for (5) above. Throw it out for the analyses in (1)-(4)
Which 95% confidence interval for the predicted glia-neuron ratio should be used to test if human brains fit the trend observed for other species?
The 95% confidence interval for the mean glia-neuron ratio of all species, which have ln(brain mass) equal to that of humans. |
||
The 95% confidence interval for the glia-neuron ratio of a randomly drawn species with a ln(brain mass) equal to that of humans. |
Which of the following is a potential problem when assessing the fit of the human brain to the glia-neuron ratio versus ln(brain mass) trend observed in other species.
The brain masses are not |
||
ln(brain mass) is not normally distributed. |
||
We are performing extrapolation. |
||
The co-efficient of determination (R2) is too low. |


Trending now
This is a popular solution!
Step by step
Solved in 5 steps with 1 images

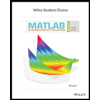
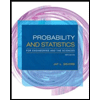
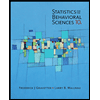
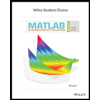
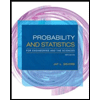
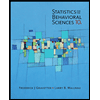
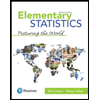
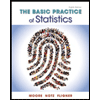
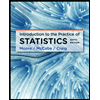