23122-khaliq
docx
keyboard_arrow_up
School
IIS University-Jaipur *
*We aren’t endorsed by this school
Course
323
Subject
Management
Date
Nov 24, 2024
Type
docx
Pages
8
Uploaded by AgentRhinoceros2355
Your preview ends here
Eager to read complete document? Join bartleby learn and gain access to the full version
- Access to all documents
- Unlimited textbook solutions
- 24/7 expert homework help
Your preview ends here
Eager to read complete document? Join bartleby learn and gain access to the full version
- Access to all documents
- Unlimited textbook solutions
- 24/7 expert homework help
Recommended textbooks for you
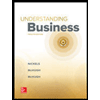
Understanding Business
Management
ISBN:9781259929434
Author:William Nickels
Publisher:McGraw-Hill Education
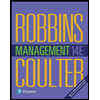
Management (14th Edition)
Management
ISBN:9780134527604
Author:Stephen P. Robbins, Mary A. Coulter
Publisher:PEARSON
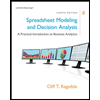
Spreadsheet Modeling & Decision Analysis: A Pract...
Management
ISBN:9781305947412
Author:Cliff Ragsdale
Publisher:Cengage Learning
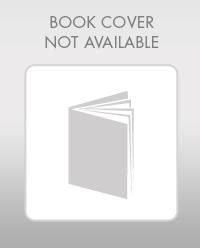
Management Information Systems: Managing The Digi...
Management
ISBN:9780135191798
Author:Kenneth C. Laudon, Jane P. Laudon
Publisher:PEARSON
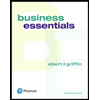
Business Essentials (12th Edition) (What's New in...
Management
ISBN:9780134728391
Author:Ronald J. Ebert, Ricky W. Griffin
Publisher:PEARSON
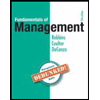
Fundamentals of Management (10th Edition)
Management
ISBN:9780134237473
Author:Stephen P. Robbins, Mary A. Coulter, David A. De Cenzo
Publisher:PEARSON
Recommended textbooks for you
- Understanding BusinessManagementISBN:9781259929434Author:William NickelsPublisher:McGraw-Hill EducationManagement (14th Edition)ManagementISBN:9780134527604Author:Stephen P. Robbins, Mary A. CoulterPublisher:PEARSONSpreadsheet Modeling & Decision Analysis: A Pract...ManagementISBN:9781305947412Author:Cliff RagsdalePublisher:Cengage Learning
- Management Information Systems: Managing The Digi...ManagementISBN:9780135191798Author:Kenneth C. Laudon, Jane P. LaudonPublisher:PEARSONBusiness Essentials (12th Edition) (What's New in...ManagementISBN:9780134728391Author:Ronald J. Ebert, Ricky W. GriffinPublisher:PEARSONFundamentals of Management (10th Edition)ManagementISBN:9780134237473Author:Stephen P. Robbins, Mary A. Coulter, David A. De CenzoPublisher:PEARSON
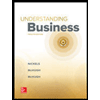
Understanding Business
Management
ISBN:9781259929434
Author:William Nickels
Publisher:McGraw-Hill Education
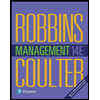
Management (14th Edition)
Management
ISBN:9780134527604
Author:Stephen P. Robbins, Mary A. Coulter
Publisher:PEARSON
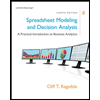
Spreadsheet Modeling & Decision Analysis: A Pract...
Management
ISBN:9781305947412
Author:Cliff Ragsdale
Publisher:Cengage Learning
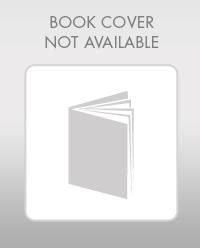
Management Information Systems: Managing The Digi...
Management
ISBN:9780135191798
Author:Kenneth C. Laudon, Jane P. Laudon
Publisher:PEARSON
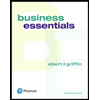
Business Essentials (12th Edition) (What's New in...
Management
ISBN:9780134728391
Author:Ronald J. Ebert, Ricky W. Griffin
Publisher:PEARSON
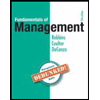
Fundamentals of Management (10th Edition)
Management
ISBN:9780134237473
Author:Stephen P. Robbins, Mary A. Coulter, David A. De Cenzo
Publisher:PEARSON