Assignment 8
docx
keyboard_arrow_up
School
Arizona State University, Tempe *
*We aren’t endorsed by this school
Course
511
Subject
Electrical Engineering
Date
Apr 3, 2024
Type
docx
Pages
7
Uploaded by HighnessWallabyMaster375
Assignment 8
Impurity Calculations using Gini & Entropy
Node Impurity Calculations
Node l
contains 20 data points.
C1= 16, C2= 4
P (C1) = 16/20 = 0.8, P(C1) = 4/20 = 0.2
Gini _ Node1 = 1- P (C1) ^2- P(C2) ^2
=1- P (16/20) ^ 2 – P (4/20) ^2
= 1 - 0.64 - 0.04
= 0.32
Gini of Node 1 = 0.32
Node 2
contains 20 data points.
C1 = 11, C2 = 9
P (C1) = 11/20 = 0.55, P(C1) = 9/20 = 0.45
Gini_Node2 = 1- P (C1) ^2 - P(C2) ^2
= 1- (0.55) ^2- (0.45) ^2
= 1 - 0.3025 - 0.2025
Gini of Node 2 = 0.495
Node 3
contains 20 data points.
C1= 10, C2= 10
P (C1) = 10/20 = 0.5, P(C2) = 10/20 = 0.5
Gini_Node3 = 1- P (C1) ^2- P(C2) ^2
=1 – P (10/20) ^2 – P (10/20) ^2
= 0.5
Gini of Node 3 = 0.5
Node 1 has the lowest Gini. And node 2 has the second lowest Gini value then comes Node 3. Node 1 is the purest because it has low Gini value and node 3 has max impurity as it has high Gini value.
Entropy of the Nodes:
Entropy for Node 1
C1= 16, C2 = 4
P(C1) = 16/20 = 0. 8, P(C2) = 4/20= 0.2
Entropy_Node1 = -(P(C1) log
2
P
(
C
1
)
+ P(C2) log
2
P
(
C
2
)
¿¿
= -(-0.8*(-0.3219) +0.2*(-2.32))
= 0.7219
Entropy for Node 2:
C1= 11, C2 = 9
P(C1) = 11/20 = 0.55, P(C2) = 9/20= 0.45
Entropy_Node2 = -(P(C1) log
2
P
(
C
1
)
+ P(C2) log
2
P
(
C
2
)
¿¿
= -((-0.47) +(-0.51))
= 0.99
Entropy for Node 3:
C1= 10, C2 = 10
P (C1) = 10/20 = 0.5, P(C2) = 10/20 = 0.5
Entropy_Node3 = -(P(C1) log
2
P
(
C
1
)
+ P(C2) log
2
P
(
C
2
)
¿¿
= -(-0.5-0.5)
Entropy of Node3= -(-1) =1
Looking at the above entropy values node 1 is the purest as it has low entropy. Node 2 and Node 3 are highly impure because their entropy is close to 1 i.e. 0.99 and 1 respectively.
Split Impurity Calculations
Split Impurity Calculations:
1. without split Gini Index
We have C1=4, C2=5
=1-P(C1)2- P(C2)2
= 1-P (4/9)2 -P (5/9)2
=1- (0.197136)- (0.308026)
= 0.4944839
2. Split on Attribute A
If A = T We have C1=2, C2=3
= 1 – (2/5) ^2 – (3/5) ^2
= 1-(0.4) ^2-(0.6) ^2
= 0.48
If A=F C1=2, C2=2
= 1- (2/4) ^2 - (2/4) ^2
= 0.5
Gini split = 5/9*(0.48) + 4/9 *(0.5)
= 0.555(0.48) + 0.444(0.5)
= 0.2667 + 0.222
= 0.489
Weighted average = 0.489
3. Split based on attribute B
If B=T C1 =2, C2= 4 = 1-(2/6) ^2-(4/6) ^2
= 1 – 0.111 – 0.4444
= 0.44
If B=F C1=2, C2=1
= 1- (2/3)2- (1/3)2
= 1 – 0.444 – 0.111
= 0.44
Gini split = 6/9*(0.44) + 3/9 *(0.44)
= 0.44
Weighted average = 0.44
4. The weighted average of GINI split B is 0.44 which is lower than 0.489 i.e. Gini split A
Which means Gini split of B attribute is purer. 5. Entropy(t) = −
∑
p
(
i
/
t
)
logp
(
j
/
t
)
Without splitting we get
c1=4, c2=5
P(C1) = 4/9 = 0.444, P(C2) = 5/9 = 0.555
E = -∑P(C1) log
2
P
(
C
1
)
+ P(C2) log
2
P
(
C
2
)
= - ((4/9) log
2
(
0.444
)
+ (5/9) log
2
(
0.555
)
)
= -(4/9(-1.171) - 5/9 (0.848))
= -(-0.529 – 0.442)
= 0.991
Entropy for Attribute A For A=T c1=2, c2=3
P(C1) = 2/5 = 0.4
P(C2) = 3/5 = 0.6
Your preview ends here
Eager to read complete document? Join bartleby learn and gain access to the full version
- Access to all documents
- Unlimited textbook solutions
- 24/7 expert homework help
Entropy(t) = - (2/5) log
2
(
0.4
)
- (3/5) log
2
(
0.6
)
= 0.971
If A=F
C1=2, c2=2
t= -(0.5) log
2
(
0.5
)
+ (0.5) log
2
P
(
0.5
)
t = 1.0
GINI Split = 5/9 * 0.97 + 4/9 * 1
= 0.983
Entropy For attribute B:
B=T c1=2, c2=4
P(C1) = 2/6 = 0.33
P(C2) = 4/6 =0.66
t= -(2/6) log
2
(
0.33
)
+ (4/6) log
2
(
0.66
)
=0.918
B=F c1=2, c2=1
P(C1) = 2/3 = 0.66
P(C2) = 1/3 = 0.333
t= -(2/3)
log
2
(
0.66
)
+ (1/3) log
2
(
0.33
)
=0.918
GINI Split = 6/9 * 0.918 + 3/9 * 0.918
= 0.918
Entropy for Attribute A is 0.983
Entropy for Attribute B is 0.918
Therefore, Entropy for Attribute B is purer.
Building a Decision Tree for a Given Dataset
Total 20 values So, G = 20
Gender spits into 2 values i.e. Male and Female
[Gender = 20] - [Male 10] [Female 10]
In Male 10 – [C0 6] and [C1 4]
So now Gini Male = 1 – p(C0) ^2 – p(C1) ^2
= 1 – [6/10] ^ 2 – [4/10] ^2
= 1 – [0.6] ^2– [ 0.4] ^2
= 0.48
And In Female - [C0 4] and [C1 6]
Gini Female= 1 – p(C0) ^2 – p(C1) ^2
= 1 – [4/10] ^2 - [6/10] ^ 2
= 0.48
SO, for our first attribute split on cap shape we have Gini index for Male and Female
Now weighted avg for the entire splits Gini index
Gini of car shape = x * Gini flat + y * Gini bell
= 10/20 * 0.48 + 10/20 * 0.48
= 0.48
This is our first splits Gini index.
Total 20 values
So, C = 20
Car Type spits into 3 values i.e. Family, Sports and Luxury
[Car Type = 20] - [Family 4] [Sports 8] [Luxury 8]
[In Family 4] – [C0 1] and [C1 3]
So now Gini Family = 1 – p(C0) ^2 – p(C1) ^2
= 1 – [1/4] ^ 2 – [3/4] ^2
= 1 – [0.25] ^2– [ 0.75] ^2
= 0.375
And In sports - [C0 8] and [C1 0]
Gini_Sports = 1 – p(C0) ^2 – p(C1) ^2
= 1 – [8/8] ^2 - 0
= 0
And In Luxury - [C0 1] and [C1 7]
Gini_Luxury = 1 – p(C0) ^2 – p(C1) ^2
= 1 – [1/8] ^ 2 – [7/8] ^2
= 0.21875
Now weighted avg for the entire splits Gini index
Gini of car Type = x * Gini Family + y * Gini Sports + y * Gini Luxury
= 4/20 * 0.375 + 8/20 * 0 + 8/20 * 0.21875
= 0.1625
This is our second split Gini index.
Your preview ends here
Eager to read complete document? Join bartleby learn and gain access to the full version
- Access to all documents
- Unlimited textbook solutions
- 24/7 expert homework help
Related Documents
Related Questions
FAIRCHILD
Discrete POWER & Signal
Technologies
SEMICONDUCTOR ru
1N4001 - 1N4007
Features
• Low torward voltage drop.
10 a14
* High aurge eurrent cepablity.
0.160 4.06)
DO 41
COLOR BAND DGNOTEs CAT-Cos
1.0 Ampere General Purpose Rectifiers
Absolute Maximum Ratings
T-26*Cuness atnerwioe rated
Symbol
Parameter
Value
Units
Average Recttied Current
1.0
375" lead length a TA - 75°C
Tsargei
Peak Forward Surge Current
8.3 ms single halr-sine-wave
Superimposed on rated load JEDEC method)
30
A
Pa
Total Device Dissipetion
2.5
20
Derste above 25°C
Ra
Tag
Thermal Resistence, Junction to Amblent
5D
Storage Temperature Range
55 to +175
-55 to +150
Operating Junetion Temperature
PC
"These rarings are imithg valuee above whien the serviceatity or any semiconductor device may te impaired.
Electrical Characteristics
T-20'Cunieas ofherwise roted
Parameter
Device
Units
4001
4002
4003
4004
4005
4006
4007
Peak Repetitive Reverse Vellage
Maximum RME votage
DC Reverse Voltage
Maximum Reverse Current
@ rated VR…
arrow_forward
Solve it fast fast plz
arrow_forward
I need the correct expert solution with explanation of the steps of the solution and the abbreviations, please.
arrow_forward
Please indicate the temperatures that these p-type semiconductors (Si) are at. (The circles
represent holes.)
[Select]
V [Select]
T
[Select]
[Select]
zero Kelvin
intermediate temperatures
any temperature
room temperature
arrow_forward
2-A) What's the name of the opamp circuit below? Briefly explain the logic.
2-B) Why is there a DC 1 volt offset in Vs sine signal?
arrow_forward
SEE MORE QUESTIONS
Recommended textbooks for you
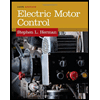
Related Questions
- FAIRCHILD Discrete POWER & Signal Technologies SEMICONDUCTOR ru 1N4001 - 1N4007 Features • Low torward voltage drop. 10 a14 * High aurge eurrent cepablity. 0.160 4.06) DO 41 COLOR BAND DGNOTEs CAT-Cos 1.0 Ampere General Purpose Rectifiers Absolute Maximum Ratings T-26*Cuness atnerwioe rated Symbol Parameter Value Units Average Recttied Current 1.0 375" lead length a TA - 75°C Tsargei Peak Forward Surge Current 8.3 ms single halr-sine-wave Superimposed on rated load JEDEC method) 30 A Pa Total Device Dissipetion 2.5 20 Derste above 25°C Ra Tag Thermal Resistence, Junction to Amblent 5D Storage Temperature Range 55 to +175 -55 to +150 Operating Junetion Temperature PC "These rarings are imithg valuee above whien the serviceatity or any semiconductor device may te impaired. Electrical Characteristics T-20'Cunieas ofherwise roted Parameter Device Units 4001 4002 4003 4004 4005 4006 4007 Peak Repetitive Reverse Vellage Maximum RME votage DC Reverse Voltage Maximum Reverse Current @ rated VR…arrow_forwardSolve it fast fast plzarrow_forwardI need the correct expert solution with explanation of the steps of the solution and the abbreviations, please.arrow_forward
- Please indicate the temperatures that these p-type semiconductors (Si) are at. (The circles represent holes.) [Select] V [Select] T [Select] [Select] zero Kelvin intermediate temperatures any temperature room temperaturearrow_forward2-A) What's the name of the opamp circuit below? Briefly explain the logic. 2-B) Why is there a DC 1 volt offset in Vs sine signal?arrow_forward
arrow_back_ios
arrow_forward_ios
Recommended textbooks for you
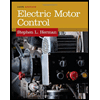