BUSINESS ANALYTICS
docx
keyboard_arrow_up
School
Moi University *
*We aren’t endorsed by this school
Course
5
Subject
Business
Date
Nov 24, 2024
Type
docx
Pages
15
Uploaded by DrMusic8745
BUSINESS ANALYTICS Designing a framework for Business Analytics
Case Study: Ford Motors
Institution Affiliation
Course
Professor’s Name
Date
Table of Contents
PART I: INTRODUCTION
................................................................................................................
3
1.0 Introduction
..............................................................................................................................
3
1.1 Background and Problem
...........................................................................................................
3
1.2 Previous research around the problem
.........................................................................................
4
1.3 Gaps in the knowledge
...............................................................................................................
4
1.4 Aims and Purposes
....................................................................................................................
4
1.5 Research questions
....................................................................................................................
4
1
BUSINESS ANALYTICS PART II: LITERATURE REVIEW/THEORETICAL FRAMEWORK
..................................................
5
2.1 Data, knowledge, information, and insights
..................................................................................
5
2.2 Business analytics
.....................................................................................................................
6
2.2.1 Business Analytics: Origins, Types, and Definitions
...............................................................
6
2.3 An Overview of Business Analytics Frameworks and Models
.......................................................
7
2.3.1 A model for building analytic capability
................................................................................
7
2.3.2 The Framework for Business Analytics
.................................................................................
8
2.3.3 The Business Analytics Framework
......................................................................................
8
PART III: RESEARCH METHODOLOGY
......................................................................................
10
3.1 Research strategy
.....................................................................................................................
10
3.2 Research design
......................................................................................................................
10
3.3 Research method
.....................................................................................................................
10
3.3.1 Interviews
........................................................................................................................
10
3.4 Research quality
......................................................................................................................
10
3.5 The Case- Ford Motors
............................................................................................................
11
3.6 Ford Global Data Management Solution (GDMS)
......................................................................
11
PART IV: EXPECTED CONTRIBUTIONS
......................................................................................
13
4.1 Strengthening the Definition of Business Analytics
.....................................................................
13
4.2 A Structured Framework for Business Analytics
.........................................................................
13
PART V: REFERENCES
..................................................................................................................
14
2
BUSINESS ANALYTICS PART I: INTRODUCTION.
This chapter should outline the Background and problem, Previous research around the problem, Gaps in the knowledge, Importance and significance of the research and Research question(s) or hypothesis
1.0 Introduction
1.1 Background and Problem
The amount of data being collected and stored worldwide has increased dramatically in recent years. There is currently enough data available worldwide to stream a 47,000-year-long series of high-definition TV shows (Acito and Khatri, 2014). According to Eric Schmitt, CEO of Google, in 2010, every two days, the world created as much data as it had from the dawn of civilization until 2003. To wit: (Hoerl et al., 2019). Information is now available to businesses via a variety of channels, including point-of-sale systems, embedded devices, and even social media platforms like Facebook and Twitter (Saxena and Srinivasan, 2018). "Big Data" refers to the massive amounts of data being generated at lightning speeds and in a wide range of formats, and it is revolutionizing the way we work with and perceive information (Saxena and Srinivasan, 2018). Just recently, the notion of "Big Data" has begun to affect nearly every facet of society, from business and government to the arts and sciences (Hoerl et al., 2019). But there are a lot of problems with utilizing these huge data quantities, which leads to failure and unhappiness (Kumar and Bhadani, 2019). The challenge for today's firms is gathering information and making insights of it (Dean, 2019).
3
Your preview ends here
Eager to read complete document? Join bartleby learn and gain access to the full version
- Access to all documents
- Unlimited textbook solutions
- 24/7 expert homework help
BUSINESS ANALYTICS Business performance management has advanced, the trend toward fact-based decision making has occurred, and the integration of analytics approaches into enterprise systems have all contributed to the emergence of business analytics. Business analytics, according to Holsapple (2019), aids an organization in deriving value and meaningful insights from its data. According to Laursen and Thorlund (2010), it involves
"evidence-based issue identification and solutions that occur within the context of business challenges" and "delivering the proper decision support to the appropriate people at the right time" (Holsapple et al., 2019). Despite having a long history, analytics has recently acquired favor among academics and professionals (Saxena and Srinivasan, 2018). Recent research indicates that successful organizations employ analytics more frequently than failed ones (LaValle et al., 2015). In order to provide a consistent, from-the-ground-up framework for Business Analytics, Holsapple et al. (2019) assembled a thorough survey of the literature on the topic. They contend that there hasn't been enough introspective research of Business Analytics as an area
of study. Holsapple et al. (2019) have urged for in-depth case studies to empirically test their conceptual framework in light of this synthesis of the previous corpus of research.
This study is a case study of the research done at Ford Motor Company in Dearborn, Michigan. Ford Motors has just lately begun looking into the potential benefits that may be gained by analyzing logged vehicle data (LVD) collected from its customers' vehicles while they are driven. Ford is now receiving and storing these records in its different data systems. However, there is currently no established plan for transforming this information into actionable insights for the company. Therefore, the purpose of this research is to apply the principles of Business Analytics to examine how Ford Motors might mine the information contained in the vehicles' on-board computers for useful insights and ultimately put those discoveries to use in the form of value-adding strategies.
1.2 Previous research around the problem.
Previous research has highlighted the need for a unified framework for business analytics. Studies have identified the need for an effective data collection and management system, as well as an effective analysis and interpretation process. In addition, research has identified the need for a comprehensive set of tools to facilitate the analysis and interpretation of data. These studies have identified the need for a comprehensive framework that can be used to turn data into actionable insights.
1.3 Gaps in the knowledge
There is a gap in the knowledge regarding how to develop a unified framework for business analytics that is both efficient and reliable. Although there are a number of tools and solutions available for data collection and management, there is a lack of comprehensive solutions that can be used to turn data into actionable insights. Furthermore, there is a lack of research on how to develop a unified framework for business analytics that can be used by businesses of all sizes.
1.4 Aims and Purposes
The primary goal of this study is to provide a business analytics framework for mining data for actionable insights using Ford Motor Company as a case study.
1.5 Research questions
1. How does the application of business analytics help Ford Motors improve its operational efficiency? 2. What strategies does Ford Motors use to leverage data-driven insights to inform their decision-making process?
3. How has the implementation of business analytics enabled Ford Motors to create a competitive edge in the automotive industry?
4
BUSINESS ANALYTICS PART II: LITERATURE REVIEW/THEORETICAL FRAMEWORK
The theoretical framework of the investigation is laid forth in this section. Several different conceptual frameworks will be discussed, and the prior literature on Business Analytics, expertise-based intuition, and the nature of insights is outlined.
2.1 Data, knowledge, information, and insights It was said up front that the creation of new data has accelerated at a dizzying rate in the past several years (Acito and Khatri, 2014). Credit card transactions, Point of Sale records, embedded systems (such as data loggers in trucks and airplanes, and radio frequency identification readers), and social networking sites like Facebook and Twitter all contribute to the massive amounts of data now available to businesses and analysts
(Saxena & Srinivasan, 2018). "Big Data" describes the current era in which data is used due to its unprecedented volume, velocity, and variety (Saxena and Srinivasan, 2018).
Although the definition of "big" data is still up for dispute, Minelli et al. (2018) and Saxena and Srinivasan (2018) both agree that it refers to datasets that go much beyond the norm in terms of volume, diversity, and velocity, all of which make them more difficult to consume, analyze, and display. According to Gandomi and Haider, the "Three V's" (volume, variety, and velocity) have evolved as the paradigm for describing Big
Data (2020). The authors concede, however, that it is not possible to establish universally applicable cutoffs for when a given value of the Three V's is considered "large," given that these boundaries vary by business size, industry, and geographic region.
5
BUSINESS ANALYTICS McKnight (2019) explains that the explosion of data forces businesses to adapt their methods of decision making to incorporate a wider range of facts. We have more data than we know what to do with, but according to Silver (2012), only a small fraction of that data is genuinely helpful; what we really need is knowledge, not data. For the sake of clarity, it is appropriate to highlight precise boundaries between data, information, and knowledge, which might appear as though they are utilized equally. Data is the platform through which information travels; however, data alone is often too specific to be useful for decision making; information is data that has been aggregated to the point where it can be used as a decision support tool; and knowledge is what is gained after the information has been analyzed and interpreted, as stated by Laursen and Thorlund (2015). Knowledge is "justified true belief," as defined by Nonaka (1994, p.15), and it is the result of having had one's mind "activated" by information.
However, the overarching goal of this research is to provide a framework for transforming raw data into useful insights, thus it is appropriate to dig into the meaning of "insights" as well. Insight is defined by Klein
and Jarosz (2016, p.346) as "discontinuous discovery, a nonobvious adjustment to a person's mental model of a dynamic system, resulting in a new set of beliefs that are more accurate, complete, and valuable." As Klein and Jarosz (2016, p.347) elaborate, "either fresh data or a combination of facts or a finding of a contradiction takes the person into a conceptual region that was new to the person in some respects" when a person has an epiphany and acquires new understanding.
People get insight in three ways: (a) via contradiction, when discrepancies in their mental models are noticed; (b) through desperation, when there is a desire to rethink the situation; and (c) through connection, when an implication is discovered. Klein and Jarosz provide this framework (2016). There are three methods to get insight into a situation, and that insight could then lead to a knowledge of how to behave. Figure 1 displays the Klein and Jarosz (2016) framework.
Figure 1 Insight's Anchor model
2.2 Business analytics
2.2.1 Business Analytics: Origins, Types, and Definitions
According to Saxena and Srinivasan (2018), management theory can be traced back to Frederick Taylor, who used analytics methodologies from observation through execution (Saxena & Srinivasan, 2018). Over time, approaches from areas like statistics, quality control, and operations research were developed and utilized, and business analysts were brought in to aid management. IT departments' business intelligence 6
Your preview ends here
Eager to read complete document? Join bartleby learn and gain access to the full version
- Access to all documents
- Unlimited textbook solutions
- 24/7 expert homework help
BUSINESS ANALYTICS and data warehousing teams started giving executives analytics dashboards and reports as a result of these innovations (Saxena and Srinivasan, 2018).
However, according to Acito and Khatri (2014), the notion of Business Analytics as it exists now is rather recent. According to them, various recent developments have converged, leading to the birth of the notion of
Business Analytics, and the massive growth in the availability of data is one of these trends. One further factor at play here is the improved link between company strategy and data that has resulted from the maturation of business performance management. The third factor is the growing awareness that choices at all levels of an organization must be based on hard data. Finally, one may see a fourth development in the widespread adoption of cutting-edge analytics tools in enterprise infrastructure.
2.3 An Overview of Business Analytics Frameworks and Models Numerous conceptual frameworks for Business Analytics have been established by academics in the years after the turn of the millennium. One of the ground-breaking discoveries is the methodology for developing an analytical competence inside an organization presented by Davenport et al. (2001).
2.3.1 A model for building analytic capability There are three main parts to the model proposed by Davenport et al. (2001), and they are the setting, the
changes made, and the results. Figure 2 shows the resulting model.
Figure 2 Analytic Capability Model
It is possible to look at the environmental elements as necessary conditions for developing analytic capacity. There are many other facets to consider, such as strategy, expertise, structure, culture, tools, and information.
In the Transform stage, the information is processed and utilized to guide next actions. The Outcome element contains the final outcomes of the analysis and decision-making; if nothing is done to alter the organization, the Context and Transformation elements are useless (Davenport et al., 2001). Outcomes may be broken down into three categories: behaviors, processes, and monetary gains. The cone shape used to represent this model is meant to emphasize the significance of the background information employed in the analytic transformation, which is the foundation for producing superior results.
7
BUSINESS ANALYTICS 2.3.2 The Framework for Business Analytics
Figure 3 depicts an alternate perspective on business analytics proposed by Laursen and Thorlund (2010). These authors highlight the multi-level and hierarchical nature of analytics, pointing out how information flows from upper-level management in a commercial context to IT professionals in a more technical context. This causes a shift in the flow of data in the opposite direction.
Figure 3 Model for Business Analytical
2.3.3 The Business Analytics Framework
Since it is the most modern, is thought to be the most all-encompassing, and its creators beg for empirical tests, the framework/model of Business Analytics described last in this literature review will serve as the initial base for this study. Holsapple et al. (2019) conducted a thorough literature study and developed a framework that is made up of six groups of viewpoints on Business Analytics. Figure 4 depicts the whole scheme.
Holsapple, and C. (2019) created a framework that includes many viewpoints, the first of which is Business Analytics as a Movement. To do this, one must embrace a philosophy and way of life in which issues are addressed and decisions are made in light of available evidence. The mentality is in place for a company to develop plans and conduct business with an eye on identifying and resolving problems based on hard data. Among the latter group, Business Analytics belongs to the category of Capabilities. A company's problem-
solving prowess is proportional to the depth and breadth of its resources. In this context, "competences" refer to the company's and its employees' abilities in areas like as qualitative and quantitative methods, systematic thinking, the use of descriptive, predictive, and prescriptive models, and the use of evidence. Access to resources isn't enough to ensure a successful business, though; it also requires having the right infrastructure and atmosphere in place.
8
BUSINESS ANALYTICS Figure 4 Framework for the Business Analytical
Using this framework, business analytics is also viewed as a Transforming process, one that takes the evidence gathered and turns it into a decision or an insight. It's safe to assume that the process of transformation makes use of and is influenced by a variety of behaviors, technologies, skill sets, and cultural
factors. From this vantage point, questions like as "what," "why," "when," and "how" are of most importance, as these pertain to the process that drives and organizes the transition. In the context of working with evidence, the terms "practices" and "technologies" relate to the methods used to accomplish goals including deepening comprehension, developing reliable predictions, and creating new forms of knowledge.
As much as 80% of an organization's data or knowledge cannot be expressed quantitatively, evidence-based
operations are not limited to only compiling statistics. Therefore, Business Analytics requires a combination of quantitative and qualitative, more practice-based methodology (Holsapple et al., 2019).
Business Analytics is defined as a process consisting of a series of stages. These steps make up an evidence-
based procedure. Accessing, analyzing, gathering, and evaluating evidence are all included in the definitions
offered by Holsapple et al (2019). These steps, in conjunction with the aforementioned ideas and tools, enable the transformation process to convert the data into useful insights or conclusions. In their final analysis, Holsapple et al. (2019) use a decisional paradigm viewpoint toward Business Analytics. This demonstrates how Business Analytics differs from methods such as experience- and intuition-based naturalistic decision making. Business analytics should be used for decision making at a bare minimum. The decisional paradigm asserts that one must look to logic and facts before making any choices.
9
Your preview ends here
Eager to read complete document? Join bartleby learn and gain access to the full version
- Access to all documents
- Unlimited textbook solutions
- 24/7 expert homework help
BUSINESS ANALYTICS PART III: RESEARCH METHODOLOGY
This chapter provides an overview of the study's methodology, detailing its approach, structure, and methodologies.
3.1 Research strategy
The qualitative method will mostly be used in this investigation. Research that uses inductive methods often
employs qualitative methods, whereas deductive methods favor the use of quantitative tools (Bryman and Bell, 2016). Theory will be used in experimentation and observation, leading to conclusions in deductive approaches. In any case, the two strategies share certain commonalities (Bryman, 2017). This research will not use inductive or deductive strategies; instead, it will take an abductive approach based on the systematic melding of theory, framework, and practice (Dubois and Gadde, 2002). This suggests that there is a theoretical underpinning underneath the empirical data, which requires additional tweaks. Thus, progress will be made in both the theoretical basis and the empirical inquiry at the same time.
3.2 Research design
Case studies, which include the use of many pieces of data to investigate a phenomenon in its natural environment, will be used in this study (Yin, 1994). According to Bryman and Bell (2019), it requires a deep
dive into a single case to fully understand all of its nuances and complexities. In order to address questions like "why," "what," and "how," this study prefers to employ a case study, as stated by Saunders et al. (2009). In fact, Holsapple et al. (2019) propose doing empirical case studies of Business Analytics; the authors of the present study agree that this is a valid approach to examining the efficacy and growth of Business Analytics in real-world settings.
3.3 Research method
One definition of a research method is "any strategy used to collect information methodically" (Bryman and
Bell, 2016). This research will collect data through literature readings, interviews, casual discussions, observations, and analyses of the case company's internal papers and reports.
3.3.1 Interviews
Structured interviews are the most typical type, followed by semi-structured and then unstructured interviews, with the former two being more qualitative than the latter (Bryman and Bell, 2019). Because this
study aims to learn more about the respondents' experiences and perspectives, as well as because doing so would allow us to collect more in-depth data, study will conduct qualitative interviews. Furthermore, semi-
structured interviews will be used because the I already have a concept of how to evaluate the data.
Predetermined questions and discussion points will serve as a framework for semi-structured interviews. Since questions can be added based on the context and flow of the conversation, semi-structured interviews give more in-depth responses to research issues. This method will also be used to the in-person interviews; the guide will serve as a blueprint, but the actual questions asked will develop organically in response to the participants' responses (Saunders et al. 2009). According to Robson (2011), interviews are a flexible approach of data collection and motivational analysis. Semi-structured interviews are difficult to shield against interviewer and interviewee prejudice, as pointed out by Robson (2011).
3.4 Research quality
It is common practice, especially in quantitative research, to evaluate studies based on their internal and external validity, reliability, and objectivity to establish the quality of the research. Nonetheless, there are many who claim that these cannot be used to qualitative research, and therefore the criteria of trustworthiness have evolved as a measure of the quality of qualitative studies (Bryman and Bell, 2016). 10
BUSINESS ANALYTICS Guba (1981) emphasizes that the credibility, transferability, dependability, and confirmability of research all contribute to its overall trustworthiness. According to Bryman and Bell (2016), a measurement of research's internal validity is whether or not it was conducted in accordance with accepted standards of practice. Respondent validation and triangulation are two methods that may be used to improve the reliability of research (Bryman and Bell, 2016). Both methods will be employed here. As previously indicated, interviewees will be provided with a copy of the transcript to double check for accuracy. Since the information gained from the interviews will be summarized, it will be checked by a key stakeholder. Triangulation will also be employed by carefully examining the procedure, the applied practices and technologies, and the operations carried out. Informal discussions with relevant parties are also undertaken in order to dispel any confusion regarding, for instance, interview responses or the operation of certain technologies and databases. Furthermore, internal papers and tools will be evaluated to verify that the writers have a firm grasp on the material and that concerns brought up in interviews can be independently confirmed. 3.5 The Case- Ford Motors
Ford Motors Company is one of the largest automotive companies in the world and has been at the forefront
of technological innovation for decades. As such, the company has been leveraging big data analytics to gain insights into their customer base, their manufacturing processes, and the competitive landscape. By utilizing analytics, Ford has been able to make better decisions, improve customer satisfaction, and stay competitive. Ford has implemented a variety of analytics solutions to help them gain insights from their data. These solutions include predictive analytics, machine learning, and artificial intelligence. Ford has also developed an app called FordPass which will allow customers to collect and analyze data about their driving
habits. This data is then used to suggest driving tips and other personalized content to the customer.
Ford has also implemented a predictive analytics platform which helps them better understand customer preferences and behaviors. This will help the company better target its marketing efforts and create more personalized experiences for its customers. Additionally, Ford has been using analytics to improve its production processes. By leveraging analytics, the company is able to identify bottlenecks and inefficiencies
in its manufacturing processes and take corrective action to improve production.
3.6 Ford Global Data Management Solution (GDMS)
The Ford Global Data Management System is a complete platform that gathers, processes, and analyzes data from many sources using big data analytics, machine learning, and AI. The information obtained from this data is then utilized to understand market trends, customer preferences, and manufacturing efficiency. By acquiring the information required to make educated decisions, Ford's data management system aids the corporation in staying one step ahead of the competition.
With the help of Ford's global data management solution, the corporation now has real-time access to data from a variety of sources, including information on customer satisfaction, dealership sales, and consumer behavior. After that, this data is merged with machine learning and predictive analytics algorithms to provide insights that may be utilized to pinpoint problem areas and spur new business prospects.
Ford is also able to swiftly recognize patterns and create plans to address market changes thanks to the data management technology. To contact potential customers, they might use it, for instance, to discover client preferences and develop customized advertising. Moreover, Ford's Global Data Management System offers the team useful insights that can be used to streamline procedures and boost productivity.
The On-Board Diagnostics (OBD) system of cars' In Use Performance Ratios (IUPR) and the emission levels of the vehicles, particularly of mono-nitrogen oxides, are the two primary forms of data that will be 11
BUSINESS ANALYTICS taken from and examined in the process under investigation (NOx). Ford will be required to inform the authorities of how these metrics performed.
Certain definitions of the OBD system have been granted by the UN and are outlined in Regulation No. 49, Revision 6. (UN, 2013). The following are these definitions: The term "OBD system" refers to an on-board system for a car or engine that is capable of: 1. detecting malfunctions that affect the engine's emission performance;
2. alerting the driver to their occurrence; 3. pinpointing the likely location of the malfunction using data stored in computer memory and transmitting that information off-board. "IUPR (In-Use Performance Ratio) is the ratio of the number of times that driving cycles relevant to that monitor or set of monitors have occurred to the number of situations under which a monitor, or a group of monitors, should have identified a problem." (UN, 2013).
Euro VI refers to the most recent engine rules in Europe. The legal lower limit for IUPR is set at 0.1 in this version. Regulations also posit a limit on the permissible quantity of NOx emissions, which is 0.69 g/kWh. In addition to these guidelines, FORD will establish additional engineering goals for its cars and engines that
place even higher expectations on them.
The analytics method and the analytics framework that will be assessed in the case study will both employ this kind of data. The goal is for FORD MOTORS to learn more about the performance of their cars from this data.
12
Your preview ends here
Eager to read complete document? Join bartleby learn and gain access to the full version
- Access to all documents
- Unlimited textbook solutions
- 24/7 expert homework help
BUSINESS ANALYTICS PART IV: EXPECTED CONTRIBUTIONS
4.1 Strengthening the Definition of Business Analytics
This study makes theoretical contributions to the field of Business Analytics by providing a new definition for the term, which will provide the foundation for the study's other theoretical contributions. The literature review of this report presents several definitions of Business Analytics, including "delivering the right decision support to the right people at the right time"; "evidence-based problem recognition and resolution that occurs within the context of business situations"; and "any data-driven process that provides insights."
Business Analytics is defined as an organizational framework for communicating timely, relevant, and actionable information to the right stakeholders, based on the empirical findings of this case study.
The authors of this study believe that the term "insights" is preferable since it encompasses "decision support" and "problem identification and resolution" from prior definitions. 4.2 A Structured Framework for Business Analytics
The previous literature explored in support of the empirical case study aided the theoretical framework effectively. According to the authors, however, no prior theoretical paradigm could have effectively defined the study endeavor and its findings. This section's objective is to provide an improved conceptual framework for Business Analytics by integrating the most pertinent components of earlier research. The Business Analytics Framework will serve as the theoretical basis for this case study, as its inclusion of a wide range of elements—transformational process, specialized activities, practices and technologies, capability set, movement, and decisional paradigm—enable a comprehensive and all-inclusive view of FORD motors' Business Analytics architecture. Therefore, it may be used to assess an organization's current level of analytical alignment and pinpoint opportunities for improvement. But terms like "movement" and "decisional paradigm" are nebulous at best and perhaps inadequate at worst. Based on Davenport et alconceptualization,.'s "organization & culture" was found to be more transparent than "movement" (2001). The term "decisional paradigm" will not be included in the resulting framework because both the literature and the empirical study stress the role of experience and intuition in decision making.
13
BUSINESS ANALYTICS PART V: REFERENCES
Acito, F. & Khatri, V. (2014) Business analytics: Why now and what next? Business Horizons 57, (5): 565-570 Bergman, B. & Klefsjö, B. (2015) Quality: from customer needs to customer satisfaction. Studentlitteratur, Lund. Bryman, A. (2017) Social research methods. Oxford University Press, Oxford. Bryman, A. & Bell, E. (2016) Business research methods. Oxford University Press, Oxford. Davenport, T.H., Harris, J.G., De Long, D.W. & Jacobson, A.L. (2001) Data to Knowledge to Results: Building an Analytic Capability. California management review, vol. 43, no. 2, pp. 117-138. Davenport, T.H. (2006) Competing on analytics. Harvard Business Review, United States.
Dean, J. (2019) Big data, data mining, and machine learning: value creation for business leaders and practitioners. John Wiley & Sons, Hoboken, N.J. Done, A., Voss, C. & Rytter, N.G. (2016) Best practice interventions: short-term impact and long-
term outcomes. Journal of Operations Management, vol. 29, no. 5, pp. 500-513 Duncan, T. & Ryan, P. (2015) Interviewing reluctant respondents: strikes, henchmen, and Gaelic games. Organizational Research Methods, vol. 13, no. 3, pp. 562-581. Gandomi, A. & Haider, M. (2020) Beyond the hype: Big data concepts, methods, and analytics. International Journal of Information Management, vol. 35, no. 2, pp. 137-144.
Gladwell, M. (2005) Blink: The power of thinking without thinking. New York: Little, Brown. Griffin, A. & Hauser, J.R. (1993) The Voice of the Customer. Marketing Science, vol. 12, no. 1, pp. 1-27.
Guba, E. G. (1981) Criteria for assessing the trustworthiness of naturalistic inquiries. ECTJ, 29(2), 75-91.
Hoerl, R.W., Snee, R.D. & De Veaux, R.D. (2019) Applying statistical thinking to ‘Big Data’ problems. Wiley Interdisciplinary Reviews: Computational Statistics, vol. 6, no. 4, pp. 222- 232. Kahneman, D. (2016) Thinking, fast and slow. Farrar, Straus and Giroux, New York.
Kahneman, D. & Klein, G. (2019) Conditions for Intuitive Expertise: A Failure to Disagree. American Psychologist, vol. 64, no. 6, pp. 515-526. Kahneman, D. & Tversky, A. (1984) Choices, values, and frames. American Psychologist, vol. 39, no. 4, pp. 341-350.
Kiron, D., R. Shockley, N. Kruschwitz, G. Finch, M. Haydock (2016) Analytics: The widening divide. Sloan Management Review, 53(3) 1-22.
Klein, G. & Jarosz, A. (2011) A Naturalistic Study of Insight. Journal of Cognitive Engineering and Decision Making, vol. 5, no. 4, pp. 335-351.
14
BUSINESS ANALYTICS Laursen, G.H.N., Thorlund, J. (2010) Business analytics: taking business intelligence beyond reporting. Wiley, Hoboken, N.J.
McKnight, W. (2019) Information management: strategies for gaining a competitive advantage with data. Morgan Kaufmann, Waltham, MA. Minelli, M., Chambers, M., Dhiraj, A. (2018) Big data, big analytics: emerging business intelligence and analytic trends for today's businesses. John Wiley & Sons, Inc, Hoboken, N.J.
Morabito, V. (2020) Big Data and Analytics: Strategic and Organizational Impacts. Springer, Cham.
Robson, C. (2011) Real world research: a resource for users of social research methods in applied settings. Wiley, Chichester
Salas, E., M.A. Rosen, D. DiazGranados (2015). Expertise-based intuition and decision making in organizations. Journal of Management 36(4) 941-973
Saunders, M., Lewis, P., & Thornhill, A. (2009) Research methods for business students. Pearson Education UK.
Saxena, R.N., Srinivasan, A. (2018) Business analytics: a practitioner's guide. Springer, New York.
Schroeder, R.G., Linderman, K., Liedtke, C. & Choo, A.S. (2008) Six Sigma: definition and underlying theory. Journal of Operations Management, vol. 26, no. 4, pp. 536-554.
15
Your preview ends here
Eager to read complete document? Join bartleby learn and gain access to the full version
- Access to all documents
- Unlimited textbook solutions
- 24/7 expert homework help
Related Documents
Recommended textbooks for you
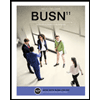
BUSN 11 Introduction to Business Student Edition
Business
ISBN:9781337407137
Author:Kelly
Publisher:Cengage Learning
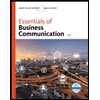
Essentials of Business Communication (MindTap Cou...
Business
ISBN:9781337386494
Author:Mary Ellen Guffey, Dana Loewy
Publisher:Cengage Learning
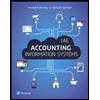
Accounting Information Systems (14th Edition)
Business
ISBN:9780134474021
Author:Marshall B. Romney, Paul J. Steinbart
Publisher:PEARSON
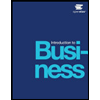
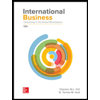
International Business: Competing in the Global M...
Business
ISBN:9781259929441
Author:Charles W. L. Hill Dr, G. Tomas M. Hult
Publisher:McGraw-Hill Education
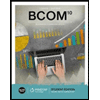
Recommended textbooks for you
- BUSN 11 Introduction to Business Student EditionBusinessISBN:9781337407137Author:KellyPublisher:Cengage LearningEssentials of Business Communication (MindTap Cou...BusinessISBN:9781337386494Author:Mary Ellen Guffey, Dana LoewyPublisher:Cengage LearningAccounting Information Systems (14th Edition)BusinessISBN:9780134474021Author:Marshall B. Romney, Paul J. SteinbartPublisher:PEARSON
- International Business: Competing in the Global M...BusinessISBN:9781259929441Author:Charles W. L. Hill Dr, G. Tomas M. HultPublisher:McGraw-Hill Education
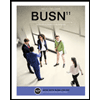
BUSN 11 Introduction to Business Student Edition
Business
ISBN:9781337407137
Author:Kelly
Publisher:Cengage Learning
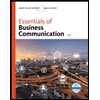
Essentials of Business Communication (MindTap Cou...
Business
ISBN:9781337386494
Author:Mary Ellen Guffey, Dana Loewy
Publisher:Cengage Learning
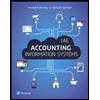
Accounting Information Systems (14th Edition)
Business
ISBN:9780134474021
Author:Marshall B. Romney, Paul J. Steinbart
Publisher:PEARSON
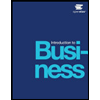
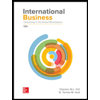
International Business: Competing in the Global M...
Business
ISBN:9781259929441
Author:Charles W. L. Hill Dr, G. Tomas M. Hult
Publisher:McGraw-Hill Education
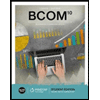