part 2
.docx
keyboard_arrow_up
School
University of Tasmania *
*We aren’t endorsed by this school
Course
743
Subject
Business
Date
Jun 22, 2024
Type
docx
Pages
7
Uploaded by JusticeAntelopePerson683941
1
Table of content
Scatterplot of the data and regression analysis
The answer to
Q. a -----------------------------------------------------------
(3)
Seasonal Patterns
The answer to
Q. b. ----------------------------------------------------------
(4)
Business reporting best practices and steps will help business reports
stand out
The answer to
Q. c -------------------------------------------------------
(4 -
5)
Reference list ------------------------------------------------------------------------- (8)
TTEC605 Data analytics
2
Q.1). Scatterplot of the data and Regression analysis
As there is a clear upward trend in the data, but fluctuations deviate from a straight line, a
linear trend does not seem to fit these data well. The data would not adequately represent
the changes in the data over time if a linear trend were to be fitted to the data.
A linear trend is a straight line that is used to model a pattern in data over time. The slope
and y-intercept of the line are calculated using the least squares method.
Once we have the final plot, we can see that the graph does indeed exhibit a distinct linear
trend. The purchase of airline tickets has undoubtedly increased over the years. When it
comes to airline ticket sales in 2019, the month of November is when they reach their
highest level.
The customer value changes and manifests a behaviour during specific months and during
certain seasons. Total ticket sales have surpassed 3,000, just like in the months of June,
May, and November. While the number of customers rises annually in January, there are
fewer customers overall than in other months. i. e 2619.
TTEC605 Data analytics
3
Q.2)
Seasonal Patterns These sales data show that there is a seasonal pattern. Sales are typically higher in May,
June, July, October, and November and lower in January, February, March, August, and
September. The seasonal component can be used to forecast future values by first
separating it from the trend and residual components using a seasonal decomposition.
This method divides the data into three parts: the trend component, which captures the
long-term changes in the data; the seasonal component, which captures the regular
fluctuations in the data; and the residual component, which captures the random
fluctuations in the data that are not explained by the trend or seasonal components. Once
the seasonal component has been determined, it can be used to predict future values under
the presumption that the pattern will persist.
Q.3
TTEC605 Data analytics
Your preview ends here
Eager to read complete document? Join bartleby learn and gain access to the full version
- Access to all documents
- Unlimited textbook solutions
- 24/7 expert homework help
Related Questions
Question 3
An organization uses a business intelligence system to predict products that tend to
be purchased together. This is an example of.
O A) Regression Analysis
B) Cluster Analysis
C) REM Analysis
D) Market Basket Analysis
arrow_forward
1- Explain the facts regarding the data, including number of variables and datapoints.
#of variables
# of observations
2- Complete the table as follows, List the variables features including, names, variable,
level, etc.
Variable
Variable Type
Variable Level
Age
Numeric
Ratio
Gender
Categorical
Sales_Rep
Business
Years
College
Personality
Certificates
Feedback
Salary
NPS
Part 3 is shown on the photo
arrow_forward
1- Explain the facts regarding the data, including number of variables and datapoints.
#of variables
# of observations
2- Complete the table as follows, List the variables features including, names, variable,
level, etc.
Variable
Variable Type
Variable Level
Age
Numeric
Ratio
Gender
Categorical
Sales_Rep
Business
Years
College
Personality
Certificates
Feedback
Salary
NPS
arrow_forward
11.2
arrow_forward
III. What is the expected value of sample information
IV. What is the efficiency of the survey information
arrow_forward
38
arrow_forward
Divvy Bikes 2021 summer ridership has increased 30% from the ridership levels in 2020. Divvy's management attributes this to 2020 having fewer work places and recreation venues were open than in 2021.
This is an example of which type of data analytics?
Question 6 options:
a)
Descriptive
b)
Diagnostic
c)
Predictive
d)
Prescriptive
arrow_forward
Only question 1
arrow_forward
Which test is best to test the hypothesis that multiple variances (2 or more) are equal?
Group of answer choices
1. t test
2. proportions test
3. Mood's median test
4. Levene's test
5. Analysis of Variance (ANOVA)
arrow_forward
Month
Actual Sales
Naive Forecast-
Absolute Value of
(# of Product X)
Sales
Errors
(# of Product X)
Jan/19
Feb/19
Mar/19
Apr/19
May/19
Jun/19
Jul/19
Aug/19
Sep/19
Oct/19
Nov/19
Dec/19
Jan/20
1,860
2,033
3,556
4,211
6,250
7,990
10,250
9,850
9,980
9,990
7,895
5,353
- Explain the calculation method for the Naive Forecast model.
the faln
arrow_forward
QUESTION 2:The manager of YTL Computers wants to develop next year’s quarterly forecasts of salesrevenue for its brand laptops. The sales are seasonal and the company believes that thefollowing most recent eight quarters of sales should be representative of next year’ssales:
Year
Quarter
Sales (millions of dollars)
1
1
9.2
1
2
5.4
1
3
4.3
1
4
14.1
2
1
10.3
2
2
6.4
2
3
5.4
2
4
16.0
Determine the forecast of next year’s quarterly sales revenue for this line of laptops.Show all your workings.
arrow_forward
Scatter plot
Which are correct?
A) positive / negative/ cluster/ not applicable
B) linear / none linear
C) strong correlation / weak correlation
arrow_forward
V.
Let's Explore / Let's Create
1. Discuss the other types of forecasting methods that financial analysts use to predict future
revenues.
2. Are there any several other factors that may need to be considered that affects the sales forecast?
RUBRIC
Criteria
Poor (3 Points)
Fair (7 Points)
Fair
Good (10 Points)
Good
Deer
arrow_forward
Q5) Monthly sales for a six month period are as follows:
Month SalesJan 18,000Feb 22,000Mar 16,000Apr 18,000May 20,000June 24,000
Compute the sales forecast for July using the following approaches: (1) Four-month moving average; (2) Weighted three-month moving average using .50 for June, .30 for May and .20 for April;(3) Exponential smoothing with α (smoothing constant) equal to .40, assuming a February forecast of 18,000
arrow_forward
Trail Questions
National Scan, Inc., sells radio frequency inventory tags.
Monthly sales for a seven-month period were as follows:
Month
Sales ('000 units)
Feb.
19
Mar.
18
Apr
15
May
20
Jun.
18
Jul.
22
Aug.
20
Forecast September sales volume using each of the following:
(1) A linear trend equation.
(2) A five-month moving average
(3) Exponential smoothing with a smoothing constant equal to
.20, assuming a March forecast of 19('000)
(4) A weighted average using 0.60 for the most recent month,
0.30 for the next most recent, and 0.10 for the next.
arrow_forward
1. Give a specific example and scenario in Hospitality Industry that we can apply the
Data Analysis Cycle.
Identify the
Problem
Identify
Available Data
Sources
Identify if
Additional Data
Sources are
needed
Statistical
Analysis
Implementation
and
Development
Communicate
Results
Maintenance
arrow_forward
06
A
B
xfx 64000
C
D
E
F
G
Roll up the individual product forecasts and compare them with the family data. Use the
family forecasts to revise the individual forecasts (both dollars & units).
PART A
Product Family Forecast
Family $
Rolled-Up $ Rolled-Down $
Family
Forecast
Forecast
Sales Goal
1
50,000
46.000
60,000
2
50,000
64.000
50,000
3
75,000
65,000
90,000
Total
175,000
175,000
200,000
,
Use the FAMILY forecast to revise the individual item forecasts (in both dollars & units)
1
2
ROLL UP
Unit
3
Product Forecast
Dollars/Unit Dollar Forecast (goal)
ROLL DOWN
Dollar
Forecast
Unit Forecast
(goal)
A
10 $ 1,000.00 $ 10,000.00
5
B
15 $ 1,200.00 $
18,000.00
6
C
20 $ 900.00 $
18,000.00
17
TOTAL
$
46,000.00
18
5 $
5,000.00 $
25,000.00
19
E
3 $
7,000.00 $
21,000.00
20
F
2 $
9,000.00 $
18,000.00 xxXX XXXX
21
TOTAL
$
64,000.00
22
G
100 $
250.00 $
25,000.00 xxxxxXXXXXXXXXXXXXXXX
23
H
24
I
180 $
220 $
100.00 $
18,000.00 xx
100.00 $
22,000.00
25
TOTAL
XXXXXXXXXXXX
XXXXXXXXXXXX
XXXX XXXXXXXXXXXX
$…
arrow_forward
NING SYSTEM (ACADEMIC)
Series forecasting for Business ||
The F-test used in testing the significance of a regression model is
O a. Upper tailed test
O b. Two tailed test
O c. Lower tailed test
O d. None
OUS PAGE
arrow_forward
Q1. Brooke is a 32-year-old, single investment banker who lives in an apartment in a large city. After a particularly long and difficult workday, she relaxed in her apartment by reading through one business magazine and two fashion magazines. When questioned by a researcher the next day, she could clearly recall two holiday ads and vaguely remembered one ad for a personal computer from among the nearly 80 ads she had seen in the three magazines. However, she could recount in detail the articles that she had read and even recalled the titles of articles she had not read. How can you explain this?
arrow_forward
Please do not give solution in image format thanku
arrow_forward
Do it accurate clean handwriting dont use chat gpt etc solve accurate
arrow_forward
Here are the errors associated with a particular forecast over the past five months, in chronological order: 2, 5, 0, -5, -10.
Which of the following statements is (are) true?
I. The forecast was too high during the fifth month.
II. The mean error over these five months is -2.
III. The forecast was perfectly accurate during one of the months.
a. I only
Ob. Il only
O. Il only
d. I and III
Oe.I, Il and II
arrow_forward
Part B?
arrow_forward
Q1) Forecasting is both art and science. Describe the situations where we use Qualitative forecasting techniques and quantitative forecasting techniques
arrow_forward
A marketing analyst wants to examine the relationship between sales (in $1,000s) and advertising (in $100s) for firms in the food and beverage Industry and collects monthly data for 25 firms. He estimates the model
Sales-o Advertising. The following ANOVA table shows a portion of the regression results.
Regression
Residual
df
1
23
55
78.53
504.02
MS
78.53
21.91
T
3.58
arrow_forward
A P A Aa A A
2。田、刻 T
Paragraph
Styles
Font
Voice
Editor
Exponential Smoothing Example
9
?
During the past 8 quarters, the Port of Baltimore has unloaded large
quantities of grain. (a = .10). The first quarter forecast was 175.
Quarter
4-5
Actual
1
180
168
Find the
3
159
forecast
for the 9th
quarter.
4
175
5.
190
205
180
182
Using a Trend Line
4-6
The demand for
electrical power
at N.Y.Edison
over the years
1997 – 2003 is
Year
Demand
1997
60
1998
65
1999
89
2000
92
given at the left.
Fin
2001
100
d the overall
2002
122
trend.
2003
110
What is the
demand for 2010
and 2011
2 words
Accessibility. Unavailable
D'Fosus
arrow_forward
Please explain the calculation to get get the answers in the column provided?!
arrow_forward
a. A cell phone Company XYZ conducted an online survey that showed that 75 percent of customers put their number-one requirement for a new phone to be increased battery life. Only 22 percent of customers listed color choices as a large concern. Company XYZ currently offers 6 different color choices for its product and has a below-average battery life compared to competition. What should Company XYZ do with these survey results?
b. Company ABC recently conducted a survey that showed that 30 percent of customers will only shop there once and never use the company’s products again. What are some general ideas that can help increase customer loyalty?
c. Company #alltheshoes would like to find out what, if anything, customers know about its new running shoe, FancyShoe. Design two survey questions to find out the following: 1) Are customers aware of the product? 2) What brand image do customers have of theproduct?
arrow_forward
Q1. Does Livestock Wealth use "inside-out" or "outside-in"?
arrow_forward
SEE MORE QUESTIONS
Recommended textbooks for you
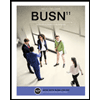
BUSN 11 Introduction to Business Student Edition
Business
ISBN:9781337407137
Author:Kelly
Publisher:Cengage Learning
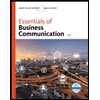
Essentials of Business Communication (MindTap Cou...
Business
ISBN:9781337386494
Author:Mary Ellen Guffey, Dana Loewy
Publisher:Cengage Learning
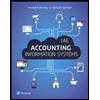
Accounting Information Systems (14th Edition)
Business
ISBN:9780134474021
Author:Marshall B. Romney, Paul J. Steinbart
Publisher:PEARSON
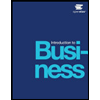
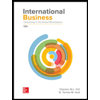
International Business: Competing in the Global M...
Business
ISBN:9781259929441
Author:Charles W. L. Hill Dr, G. Tomas M. Hult
Publisher:McGraw-Hill Education
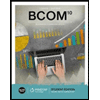
Related Questions
- Question 3 An organization uses a business intelligence system to predict products that tend to be purchased together. This is an example of. O A) Regression Analysis B) Cluster Analysis C) REM Analysis D) Market Basket Analysisarrow_forward1- Explain the facts regarding the data, including number of variables and datapoints. #of variables # of observations 2- Complete the table as follows, List the variables features including, names, variable, level, etc. Variable Variable Type Variable Level Age Numeric Ratio Gender Categorical Sales_Rep Business Years College Personality Certificates Feedback Salary NPS Part 3 is shown on the photoarrow_forward1- Explain the facts regarding the data, including number of variables and datapoints. #of variables # of observations 2- Complete the table as follows, List the variables features including, names, variable, level, etc. Variable Variable Type Variable Level Age Numeric Ratio Gender Categorical Sales_Rep Business Years College Personality Certificates Feedback Salary NPSarrow_forward
- Divvy Bikes 2021 summer ridership has increased 30% from the ridership levels in 2020. Divvy's management attributes this to 2020 having fewer work places and recreation venues were open than in 2021. This is an example of which type of data analytics? Question 6 options: a) Descriptive b) Diagnostic c) Predictive d) Prescriptivearrow_forwardOnly question 1arrow_forwardWhich test is best to test the hypothesis that multiple variances (2 or more) are equal? Group of answer choices 1. t test 2. proportions test 3. Mood's median test 4. Levene's test 5. Analysis of Variance (ANOVA)arrow_forward
- Month Actual Sales Naive Forecast- Absolute Value of (# of Product X) Sales Errors (# of Product X) Jan/19 Feb/19 Mar/19 Apr/19 May/19 Jun/19 Jul/19 Aug/19 Sep/19 Oct/19 Nov/19 Dec/19 Jan/20 1,860 2,033 3,556 4,211 6,250 7,990 10,250 9,850 9,980 9,990 7,895 5,353 - Explain the calculation method for the Naive Forecast model. the falnarrow_forwardQUESTION 2:The manager of YTL Computers wants to develop next year’s quarterly forecasts of salesrevenue for its brand laptops. The sales are seasonal and the company believes that thefollowing most recent eight quarters of sales should be representative of next year’ssales: Year Quarter Sales (millions of dollars) 1 1 9.2 1 2 5.4 1 3 4.3 1 4 14.1 2 1 10.3 2 2 6.4 2 3 5.4 2 4 16.0 Determine the forecast of next year’s quarterly sales revenue for this line of laptops.Show all your workings.arrow_forwardScatter plot Which are correct? A) positive / negative/ cluster/ not applicable B) linear / none linear C) strong correlation / weak correlationarrow_forward
arrow_back_ios
SEE MORE QUESTIONS
arrow_forward_ios
Recommended textbooks for you
- BUSN 11 Introduction to Business Student EditionBusinessISBN:9781337407137Author:KellyPublisher:Cengage LearningEssentials of Business Communication (MindTap Cou...BusinessISBN:9781337386494Author:Mary Ellen Guffey, Dana LoewyPublisher:Cengage LearningAccounting Information Systems (14th Edition)BusinessISBN:9780134474021Author:Marshall B. Romney, Paul J. SteinbartPublisher:PEARSON
- International Business: Competing in the Global M...BusinessISBN:9781259929441Author:Charles W. L. Hill Dr, G. Tomas M. HultPublisher:McGraw-Hill Education
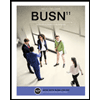
BUSN 11 Introduction to Business Student Edition
Business
ISBN:9781337407137
Author:Kelly
Publisher:Cengage Learning
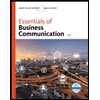
Essentials of Business Communication (MindTap Cou...
Business
ISBN:9781337386494
Author:Mary Ellen Guffey, Dana Loewy
Publisher:Cengage Learning
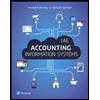
Accounting Information Systems (14th Edition)
Business
ISBN:9780134474021
Author:Marshall B. Romney, Paul J. Steinbart
Publisher:PEARSON
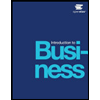
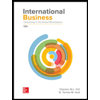
International Business: Competing in the Global M...
Business
ISBN:9781259929441
Author:Charles W. L. Hill Dr, G. Tomas M. Hult
Publisher:McGraw-Hill Education
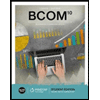