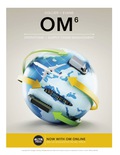
Interpretation: The best smoothing constant needs to be determined by evaluating the MSE .
Concept Introduction: Single Exponential Smoothing is a method which computes the weighted average of previous sales data to forecast the future sales value.

Explanation of Solution
Following formula can be used to calculate the forecasted values:
The Mean Square Error for various values of a is as follows:
At a = 0.1
Period | Observation | Forecast | Error | Squared Error |
1 | 1623 | 1623 | ||
2 | 1533 | 1623 | 90 | 8100 |
3 | 1455 | 1614 | 159 | 25281 |
4 | 1386 | 1598.1 | 212.1 | 44986.41 |
5 | 1209 | 1576.89 | 367.89 | 135343.0521 |
6 | 1348 | 1540.101 | 192.101 | 36902.7942 |
7 | 1581 | 1520.8909 | 60.1091 | 3613.103903 |
8 | 1332 | 1526.90181 | 194.9018 | 37986.71554 |
9 | 1245 | 1507.411629 | 262.4116 | 68859.86303 |
10 | 1521 | 1481.170466 | 39.8295 | 1586.38907 |
11 | 1421 | 1485.153419 | 64.15342 | 4115.661232 |
12 | 1502 | 1478.738078 | 23.2619 | 541.1159916 |
13 | 1656 | 1481.06427 | 174.396 | 30413.96482 |
14 | 1614 | 1498.557843 | 115.442 | 13326.85536 |
15 | 1332 | 1510.102059 | 178.1021 | 31720.34325 |
16 | 1492.291853 | SUM | 442777.2685 | |
MSE | 34059.79 |
At a = 0.2
Period | Observation | Forecast | Error | Squared Error |
1 | 1623 | 1623 | ||
2 | 1533 | 1623 | 90 | 8100 |
3 | 1455 | 1605 | 150 | 22500 |
4 | 1386 | 1575 | 212.1 | 44986.41 |
5 | 1209 | 1537.2 | 367.89 | 135343.0521 |
6 | 1348 | 1471.56 | 123.56 | 15267.0736 |
7 | 1581 | 1446.848 | 134.152 | 17996.7591 |
8 | 1332 | 1473.6784 | 141.6784 | 20072.76903 |
9 | 1245 | 1445.34272 | 200.3427 | 40137.20546 |
10 | 1521 | 1405.274176 | 39.8295 | 1586.38907 |
11 | 1421 | 1428.419341 | 7.419341 | 55.04661791 |
12 | 1502 | 1426.935473 | 23.2619 | 541.1159916 |
13 | 1656 | 1441.948378 | 174.396 | 30413.96482 |
14 | 1614 | 1484.758702 | 115.442 | 13326.85536 |
15 | 1332 | 1510.606962 | 178.607 | 31900.44687 |
16 | 1474.88557 | SUM | 382227.088 | |
MSE | 29402.08 |
At a = 0.3
Period | Observation | Forecast | Error | Squared Error |
1 | 1623 | 1623 | ||
2 | 1533 | 1623 | 90 | 8100 |
3 | 1455 | 1596 | 141 | 19881 |
4 | 1386 | 1553.7 | 212.1 | 44986.41 |
5 | 1209 | 1503.39 | 367.89 | 135343.0521 |
6 | 1348 | 1415.073 | 67.073 | 4498.787329 |
7 | 1581 | 1394.9511 | 186.0489 | 34614.19319 |
8 | 1332 | 1450.76577 | 118.7658 | 14105.30812 |
9 | 1245 | 1415.136039 | 170.136 | 28946.27177 |
10 | 1521 | 1364.095227 | 39.8295 | 1586.38907 |
11 | 1421 | 1411.166659 | 9.83334 | 96.69457556 |
12 | 1502 | 1414.116661 | 23.2619 | 541.1159916 |
13 | 1656 | 1440.481663 | 174.396 | 30413.96482 |
14 | 1614 | 1505.137164 | 115.442 | 13326.85536 |
15 | 1332 | 1537.796015 | 205.796 | 42351.99973 |
16 | 1476.05721 | SUM | 378792.0421 | |
MSE | 29137.84 |
At a = 0.4
Period | Observation | Forecast | Error | Squared Error |
1 | 1623 | 1623 | ||
2 | 1533 | 1623 | 90 | 8100 |
3 | 1455 | 1587 | 132 | 17424 |
4 | 1386 | 1534.2 | 212.1 | 44986.41 |
5 | 1209 | 1474.92 | 367.89 | 135343.0521 |
6 | 1348 | 1368.552 | 20.552 | 422.384704 |
7 | 1581 | 1360.3312 | 220.6688 | 48694.71929 |
8 | 1332 | 1448.59872 | 116.5987 | 13595.26151 |
9 | 1245 | 1401.959232 | 156.9592 | 24636.20051 |
10 | 1521 | 1339.175539 | 39.8295 | 1586.38907 |
11 | 1421 | 1411.905324 | 9.83334 | 96.69457556 |
12 | 1502 | 1415.543194 | 23.2619 | 541.1159916 |
13 | 1656 | 1450.125916 | 174.396 | 30413.96482 |
14 | 1614 | 1532.47555 | 115.442 | 13326.85536 |
15 | 1332 | 1565.08533 | 233.0853 | 54328.77103 |
16 | 1471.851198 | SUM | 393495.819 | |
MSE | 30268.909 |
At a = 0.5
Period | Observation | Forecast | Error | Squared Error |
1 | 1623 | 1623 | ||
2 | 1533 | 1623 | 90 | 8100 |
3 | 1455 | 1578 | 123 | 15129 |
4 | 1386 | 1516.5 | 212.1 | 44986.41 |
5 | 1209 | 1451.25 | 367.89 | 135343.0521 |
6 | 1348 | 1330.125 | 17.875 | 319.515625 |
7 | 1581 | 1339.0625 | 241.9375 | 58533.75391 |
8 | 1332 | 1460.03125 | 128.0313 | 16392.00098 |
9 | 1245 | 1396.015625 | 151.0156 | 22805.71899 |
10 | 1521 | 1320.507813 | 39.8295 | 1586.38907 |
11 | 1421 | 1420.753906 | 9.83334 | 96.69457556 |
12 | 1502 | 1420.876953 | 23.2619 | 541.1159916 |
13 | 1656 | 1461.438477 | 174.396 | 30413.96482 |
14 | 1614 | 1558.719238 | 115.442 | 13326.85536 |
15 | 1332 | 1586.359619 | 254.3596 | 64698.81585 |
16 | 1459.17981 | SUM | 412273.2873 | |
MSE | 31713.329 |
At a = 0.6
Period | Observation | Forecast | Error | Squared Error |
1 | 1623 | 1623 | ||
2 | 1533 | 1623 | 90 | 8100 |
3 | 1455 | 1569 | 114 | 12996 |
4 | 1386 | 1500.6 | 212.1 | 44986.41 |
5 | 1209 | 1431.84 | 367.89 | 135343.0521 |
6 | 1348 | 1298.136 | 17.875 | 319.515625 |
7 | 1581 | 1328.0544 | 252.9456 | 63981.47656 |
8 | 1332 | 1479.82176 | 147.8218 | 21851.27273 |
9 | 1245 | 1391.128704 | 146.1287 | 21353.59813 |
10 | 1521 | 1303.451482 | 39.8295 | 1586.38907 |
11 | 1421 | 1433.980593 | 9.83334 | 96.69457556 |
12 | 1502 | 1426.192237 | 23.2619 | 541.1159916 |
13 | 1656 | 1471.676895 | 174.396 | 30413.96482 |
14 | 1614 | 1582.270758 | 115.442 | 13326.85536 |
15 | 1332 | 1601.308303 | 269.3083 | 72526.96216 |
16 | 1439.723321 | SUM | 427423.3071 | |
MSE | 32878.71 |
At a = 0.7
Period | Observation | Forecast | Error | Squared Error |
1 | 1623 | 1623 | ||
2 | 1533 | 1623 | 90 | 8100 |
3 | 1455 | 1560 | 105 | 11025 |
4 | 1386 | 1486.5 | 212.1 | 44986.41 |
5 | 1209 | 1416.15 | 367.89 | 135343.0521 |
6 | 1348 | 1271.145 | 17.875 | 319.515625 |
7 | 1581 | 1324.9435 | 256.0565 | 65564.93119 |
8 | 1332 | 1504.18305 | 172.1831 | 29647.00271 |
9 | 1245 | 1383.654915 | 138.6549 | 19225.18545 |
10 | 1521 | 1286.596475 | 39.8295 | 1586.38907 |
11 | 1421 | 1450.678942 | 9.83334 | 96.69457556 |
12 | 1502 | 1429.903683 | 23.2619 | 541.1159916 |
13 | 1656 | 1480.371105 | 174.396 | 30413.96482 |
14 | 1614 | 1603.311331 | 115.442 | 13326.85536 |
15 | 1332 | 1610.793399 | 278.7934 | 77725.75957 |
16 | 1415.63802 | SUM | 437901.8765 | |
MSE | 33684.75 |
At a = 0.8
Period | Observation | Forecast | Error | Squared Error |
1 | 1623 | 1623 | ||
2 | 1533 | 1623 | 90 | 8100 |
3 | 1455 | 1551 | 96 | 9216 |
4 | 1386 | 1474.2 | 212.1 | 44986.41 |
5 | 1209 | 1403.64 | 367.89 | 135343.0521 |
6 | 1348 | 1247.928 | 17.875 | 319.515625 |
7 | 1581 | 1327.9856 | 253.0144 | 64016.28661 |
8 | 1332 | 1530.39712 | 198.3971 | 39361.41722 |
9 | 1245 | 1371.679424 | 126.6794 | 16047.67646 |
10 | 1521 | 1270.335885 | 39.8295 | 1586.38907 |
11 | 1421 | 1470.867177 | 9.83334 | 96.69457556 |
12 | 1502 | 1430.973435 | 23.2619 | 541.1159916 |
13 | 1656 | 1487.794687 | 174.396 | 30413.96482 |
14 | 1614 | 1622.358937 | 115.442 | 13326.85536 |
15 | 1332 | 1615.671787 | 283.6718 | 80469.68301 |
16 | 1388.734357 | SUM | 443825.0609 | |
MSE | 34140.38 |
At a = 0.9
Period | Observation | Forecast | Error | Squared Error |
1 | 1623 | 1623 | ||
2 | 1533 | 1623 | 90 | 8100 |
3 | 1455 | 1542 | 87 | 7569 |
4 | 1386 | 1463.7 | 212.1 | 44986.41 |
5 | 1209 | 1393.77 | 367.89 | 135343.0521 |
6 | 1348 | 1227.477 | 17.875 | 319.515625 |
7 | 1581 | 1335.9477 | 245.0523 | 60050.62974 |
8 | 1332 | 1556.49477 | 224.4948 | 50397.90176 |
9 | 1245 | 1354.449477 | 109.4495 | 11979.18802 |
10 | 1521 | 1255.944948 | 39.8295 | 1586.38907 |
11 | 1421 | 1494.494495 | 9.83334 | 96.69457556 |
12 | 1502 | 1428.349449 | 23.2619 | 541.1159916 |
13 | 1656 | 1494.634945 | 174.396 | 30413.96482 |
14 | 1614 | 1639.863494 | 115.442 | 13326.85536 |
15 | 1332 | 1616.586349 | 284.5863 | 80989.39029 |
16 | 1360.458635 | SUM | 445700.1073 | |
MSE | 34284.623 |
After evaluating the MSE of all
After comparing the MSEs of Exponential smoothing method performed in this question and moving average method in previous problem, The moving average method seems better option based on the given data.
Want to see more full solutions like this?
- The results of your four plans will provide an indicative EOQ value. State this value and discuss in a precise manner, why it is not the exact, true value. Additional calculations in the form of plans E, F etc. may also assist your explanation of the EOQ and can be includedarrow_forwardi). Complete the table assuming a Level production plan. ii) Comment on your results and explain whether at this stage, you consider a Level plan is a suitable approach for this particular business. Your comment should include reference to a calculated ‘fill rate’.arrow_forwardIn the following sawtooth inventory profile diagram, two inventory plans with different order quantities (Q) and different frequencies of delivery are shown; order quantity for Plan A = 200 units and Plan B = 50 units. i). Total demand (D) is 350 units, the holding cost per unit (Ch) is equal to (£0.8) and the ordering cost per order (Co) is (£12.5). Calculate the total costs for each plan and state which one is more preferable along with the reason why. ii). There is a stark difference in the composition of the total costs of Plans A and B. Explain this difference and why it occurs. Use the breakdown of costs for each plan to help illustrate your answer.arrow_forward
- i). Complete the table for a Chase production plan. ii). Explain whether a Level or Chase plan is more suitable for the demand pattern experienced by this particular business, which incidentally relies on highly skilled workers in the production process. Assume a starting workforce of 7 and that fractional workers are permissible. You should support your answer with numerical data derived from Table 3. In comparing the costs, state any other assumptions made.arrow_forwardi). Complete for a Chase production plan. ii). Explain whether a Level or Chase plan is more suitable for the demand pattern experienced by this particular business, which incidentally relies on highly skilled workers in the production process. Assume a starting workforce of 7 and that fractional workers are permissible.arrow_forwardComplete the table for a Chase production plan.arrow_forward
- How much can the garden centre expect to sell during each quarter of next year (Year 3) accounting for seasonality? Your forecast must make use of seasonal indices. All workings must be shown in full. (NOTE: Please round your calculations to three decimal places).arrow_forwardPS.53 Brother I.D. Ricks is a faculty member at BYU-Idaho whose grandchildren live in Oklahoma and California. He and his wife would like to visit their grandchildren at least once a year in these states. They currently have one vehicle with well over 100,000 miles on it, so they want to buy a newer vehicle with fewer miles and that gets better gas mileage. They are considering two options: (1) a new subcompact car that would cost $18,750 to purchase or (2) a used sedan that would cost $12,750.They anticipate that the new subcompact would get 37 miles per gallon (combined highway and around town driving) while the sedan would get 26 miles per gallon. Based on their road tripping history they expect to drive 13,000 miles per year. For the purposes of their analysis they are assuming that gas will cost $2.93 per gallon.Question: How many miles would the Ricks need to drive before the cost of these two options would be the same? (Display your answer to the nearest whole number.) (Hint:…arrow_forwardTisjTzktxyduduarrow_forward
- Not use ai pleasearrow_forwardNot use ai pleasearrow_forwardWhat is Bitcoin? How important is it to businesses and individuals? How does it cause environmental damage and is that damage worth continued uses of Bitcoin? Read the following articles to help answer these questions: United Nations University. (2023, October 24). UN study reveals the hidden environmental impacts of bitcoin: Carbon is not the only harmful by-product. https://unu.edu/press-release/un-study-reveals-hidden-environmental-impacts-bitcoin-carbon-not-only-harmful-product#:~:text=Bitcoin%2C%20the%20most%20popular%20cryptocurrency,the%20worldwide%20Bitcoin%20mining%20network. Salam, E. (2023, April 26). Bitcoin is terrible for the environment – can it ever go green? The Guardian. https://www.theguardian.com/technology/2023/apr/26/bitcoin-mining-climate-crisis-environmental-impactarrow_forward
- MarketingMarketingISBN:9780357033791Author:Pride, William MPublisher:South Western Educational PublishingPractical Management ScienceOperations ManagementISBN:9781337406659Author:WINSTON, Wayne L.Publisher:Cengage,Contemporary MarketingMarketingISBN:9780357033777Author:Louis E. Boone, David L. KurtzPublisher:Cengage Learning
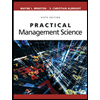
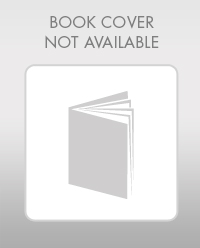