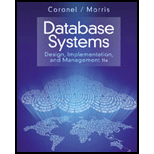
Concept explainers
“COUNT()” function:
In SQL, the “COUNT ()” function will return the total number of rows in the table with satisfying the criteria mentioned in the “WHERE” clause.
DISTINCTROW Keyword:
The “DISTINCTROW” keyword omits data based entire duplicate records, not like just duplicate fields. The syntax for “DISTINCT” keyword is as follows:
Syntax:
SELECT DISTINCTROWcol_Name FROM table_Name1 INNER JOIN table_Name2 ON table_Name1.col_Name = table_Name2.col_Name ORDER BY table_Name1;
SUM function:
The “SUM” function is used to add the value and then return the sum of the expression in a “SELECT” statement.
Syntax:
SELECT SUM (exp) FROM table_Name [WHERE condition];
GROUP BY Clause:
The GROUPBY clause is used to group the result of a SELECT statement done on a table where the tuple values are similar for more than one column
Syntax:
SELECT expression1, expression2, expression_n, aggregate_function(expression)FROMtable_name WHERE conditions GROUP BY expression1, expression2, expression_n;
INNER JOIN keyword:
“INNER JOIN” keyword is used to select all the matching records of both the table.
Syntax:
SELECT col_Name FROM table_Name1 INNER JOIN table_Name2 ON table_Name1.col_Name = table_Name2.col_Name;

Trending nowThis is a popular solution!

Chapter 7 Solutions
Database Systems: Design, Implementation, & Management
- Need help with coding in this in python!arrow_forwardIn the diagram, there is a green arrow pointing from Input C (complete data) to Transformer Encoder S_B, which I don’t understand. The teacher model is trained on full data, but S_B should instead receive missing data—this arrow should not point there. Please verify and recreate the diagram to fix this issue. Additionally, the newly created diagram should meet the same clarity standards as the second diagram (Proposed MSCATN). Finally provide the output image of the diagram in image format .arrow_forwardPlease provide me with the output image of both of them . below are the diagrams code make sure to update the code and mentionned clearly each section also the digram should be clearly describe like in the attached image. please do not provide the same answer like in other question . I repost this question because it does not satisfy the requirment I need in terms of clarifty the output of both code are not very well details I have two diagram : first diagram code graph LR subgraph Teacher Model (Pretrained) Input_Teacher[Input C (Complete Data)] --> Teacher_Encoder[Transformer Encoder T] Teacher_Encoder --> Teacher_Prediction[Teacher Prediction y_T] Teacher_Encoder --> Teacher_Features[Internal Features F_T] end subgraph Student_A_Model[Student Model A (Handles Missing Values)] Input_Student_A[Input M (Data with Missing Values)] --> Student_A_Encoder[Transformer Encoder E_A] Student_A_Encoder --> Student_A_Prediction[Student A Prediction y_A] Student_A_Encoder…arrow_forward
- Why I need ?arrow_forwardHere are two diagrams. Make them very explicit, similar to Example Diagram 3 (the Architecture of MSCTNN). graph LR subgraph Teacher_Model_B [Teacher Model (Pretrained)] Input_Teacher_B[Input C (Complete Data)] --> Teacher_Encoder_B[Transformer Encoder T] Teacher_Encoder_B --> Teacher_Prediction_B[Teacher Prediction y_T] Teacher_Encoder_B --> Teacher_Features_B[Internal Features F_T] end subgraph Student_B_Model [Student Model B (Handles Missing Labels)] Input_Student_B[Input C (Complete Data)] --> Student_B_Encoder[Transformer Encoder E_B] Student_B_Encoder --> Student_B_Prediction[Student B Prediction y_B] end subgraph Knowledge_Distillation_B [Knowledge Distillation (Student B)] Teacher_Prediction_B -- Logits Distillation Loss (L_logits_B) --> Total_Loss_B Teacher_Features_B -- Feature Alignment Loss (L_feature_B) --> Total_Loss_B Partial_Labels_B[Partial Labels y_p] -- Prediction Loss (L_pred_B) --> Total_Loss_B Total_Loss_B -- Backpropagation -->…arrow_forwardPlease provide me with the output image of both of them . below are the diagrams code I have two diagram : first diagram code graph LR subgraph Teacher Model (Pretrained) Input_Teacher[Input C (Complete Data)] --> Teacher_Encoder[Transformer Encoder T] Teacher_Encoder --> Teacher_Prediction[Teacher Prediction y_T] Teacher_Encoder --> Teacher_Features[Internal Features F_T] end subgraph Student_A_Model[Student Model A (Handles Missing Values)] Input_Student_A[Input M (Data with Missing Values)] --> Student_A_Encoder[Transformer Encoder E_A] Student_A_Encoder --> Student_A_Prediction[Student A Prediction y_A] Student_A_Encoder --> Student_A_Features[Student A Features F_A] end subgraph Knowledge_Distillation_A [Knowledge Distillation (Student A)] Teacher_Prediction -- Logits Distillation Loss (L_logits_A) --> Total_Loss_A Teacher_Features -- Feature Alignment Loss (L_feature_A) --> Total_Loss_A Ground_Truth_A[Ground Truth y_gt] -- Prediction Loss (L_pred_A)…arrow_forward
- Database Systems: Design, Implementation, & Manag...Computer ScienceISBN:9781305627482Author:Carlos Coronel, Steven MorrisPublisher:Cengage LearningProgramming with Microsoft Visual Basic 2017Computer ScienceISBN:9781337102124Author:Diane ZakPublisher:Cengage LearningDatabase Systems: Design, Implementation, & Manag...Computer ScienceISBN:9781285196145Author:Steven, Steven Morris, Carlos Coronel, Carlos, Coronel, Carlos; Morris, Carlos Coronel and Steven Morris, Carlos Coronel; Steven Morris, Steven Morris; Carlos CoronelPublisher:Cengage Learning
- A Guide to SQLComputer ScienceISBN:9781111527273Author:Philip J. PrattPublisher:Course Technology Ptr
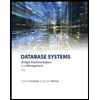
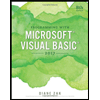
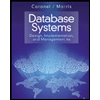
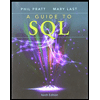
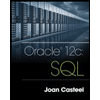