Concept explainers
(a)
To calculate; the
(b)
To calculate; the least-squares regression line for predicting average credit score (y) from average number of late payments (x)
(c)
To construct; a residual plot for the given data
(d)
To calculate; the least-squares regression line using only those points with
(e)
To calculate; the least-squares regression line using only those points with
(f)
To calculate; the coefficient of determination using only those points with
(g)
To calculate; the coefficient of determination using only those points with
(h)
To calculate; the situation in late payments and credit score are more strongly related i.e.

Want to see the full answer?
Check out a sample textbook solution
Chapter 4 Solutions
ELEMENTARY STATISTICS W/CONNECT >C<
- Olympic Pole Vault The graph in Figure 7 indicates that in recent years the winning Olympic men’s pole vault height has fallen below the value predicted by the regression line in Example 2. This might have occurred because when the pole vault was a new event there was much room for improvement in vaulters’ performances, whereas now even the best training can produce only incremental advances. Let’s see whether concentrating on more recent results gives a better predictor of future records. (a) Use the data in Table 2 (page 176) to complete the table of winning pole vault heights shown in the margin. (Note that we are using x=0 to correspond to the year 1972, where this restricted data set begins.) (b) Find the regression line for the data in part ‚(a). (c) Plot the data and the regression line on the same axes. Does the regression line seem to provide a good model for the data? (d) What does the regression line predict as the winning pole vault height for the 2012 Olympics? Compare this predicted value to the actual 2012 winning height of 5.97 m, as described on page 177. Has this new regression line provided a better prediction than the line in Example 2?arrow_forwardUse the Financial database from “Excel Databases.xls” on Blackboard. Use Total Revenues, Total Assets, Return on Equity, Earnings Per Share, Average Yield, and Dividends Per Share to predict the average P/E ratio for a company. Use Excel to develop the multiple linear regression model. Assume a 5% level of significance. Which independent variable is the strongest predictor of the average P/E ratio of a company? A. Total Revenues B. Average Yield C. Earnings Per Share D.Return on Equity E. Total Assets F.Dividends Per Share Company Type Total Revenues Total Assets Return on Equity Earnings per Share Average Yield Dividends per Share Average P/E Ratio AFLAC 6 7251 29454 17.1 2.08 0.9 0.22 11.5 Albertson's 4 14690 5219 21.4 2.08 1.6 0.63 19 Allstate 6 20106 80918 20.1 3.56 1 0.36 10.6 Amerada Hess 7 8340 7935 0.2 0.08 1.1 0.6 698.3 American General 6 3362 80620 7.1 2.19 3 1.4 21.2 American Stores 4 19139 8536 12.2 1.01 1.4 0.34 23.5 Amoco 7 36287…arrow_forwardExplain why the predictor variable is useless as a predictor of the response variable if the slope of the population regression line is 0.arrow_forward
- What is the relationship between diamond price and carat size? 307 diamonds were sampled and a straight-line relationship was hypothesized between y = diamond price (in dollars) and x = size of the diamond (in carats). The simple linear regression for the analysis is shown below: Least Squares Linear Regression of PRICE Interpret the standard deviation of the regression model. a) We expect most of the sampled diamond prices to fall within $1117.56 of their least squares predicted values. b) We can explain 89.25% of the variation in the sampled diamond prices around their mean using the size of the diamond in a linear model. c) For every 1-carat increase in the size of a diamond, we estimate that the price of the diamond will increase by $1117.56. d) We expect most of the sampled diamond prices to fall within $2235.12 of their least squares predicted values.arrow_forwardOnline clothes II For the online clothing retailer dis-cussed in the previous problem, the scatterplot of Total Yearly Purchases by Income showsThe correlation between Total Yearly Purchases and Incomeis 0.722. Summary statistics for the two variables are: a) What is the linear regression equation for predictingTotal Yearly Purchase from Income? b) Do the assumptions and conditions for regression ap-pear to be met? c) What is the predicted average Total Yearly Purchasefor someone with a yearly Income of $20,000? Forsomeone with an annual Income of $80,000?d) What percent of the variability in Total YearlyPurchases is accounted for by this model?e) Do you think the regression might be a useful one forthe company? Comment.arrow_forwardWe have data from 209 publicly traded companies (circa 2010) indicating sales and compensation information at the firm-level. We are interested in predicting a company's sales based on the CEO's salary. The variable sales; represents firm i's annual sales in millions of dollars. The variable salary; represents the salary of a firm i's CEO in thousands of dollars. We use least-squares to estimate the linear regression sales; = a + ßsalary; + ei and get the following regression results: regress sales salary . Source Model Residual Total sales salary _cons SS 337920405 2.3180e+10 2.3518e+10 df 1 207 208 Coef. Std. Err. .9287785 .5346574 5733.917 1002.477 MS 337920405 111980203 113066454 t Number of obs F(1, 207) Prob> F R-squared Adj R-squared Root MSE P>|t| 1.74 0.084 5.72 0.000 209 3.02 -.1252934 3757.543 0.0838 0.0144 0.0096 10582 [95% Conf. Interval] 1.98285 7710.291 This output tells us the regression line equation is sales = 5,733.917 +0.9287785 salary. Suppose a CEO of a company…arrow_forward
- We have data from 209 publicly traded companies (circa 2010) indicating sales and compensation information at the firm-level. We are interested in predicting a company's sales based on the CEO's salary. The variable sales; represents firm i's annual sales in millions of dollars. The variable salary; represents the salary of a firm i's CEO in thousands of dollars. We use least-squares to estimate the linear regression sales; = a + ßsalary; + ei and get the following regression results: . regress sales salary Source Model Residual Total sales salary cons SS 337920405 2.3180e+10 2.3518e+10 df 1 207 208 Coef. Std. Err. .9287785 .5346574 5733.917 1002.477 MS 337920405 111980203 113066454 Number of obs F (1, 207) Prob > F R-squared t P>|t| = Adj R-squared = Root MSE 1.74 0.084 5.72 0.000 = = -.1252934 3757.543 = 209 3.02 0.0838 0.0144 0.0096 10582 [95% Conf. Interval] 1.98285 7710.291 This output tells us the regression line equation is sales = 5,733.917 +0.9287785 salary. Interpret the…arrow_forwardUse the Financial database from “Excel Databases.xls” on Blackboard. Use Total Revenues, Total Assets, Return on Equity, Earnings Per Share, Average Yield, and Dividends Per Share to predict the average P/E ratio for a company. Use Excel to perform a forward selection regression analysis. Assume a 5% level of significance. Which independent variables are in the final model? Select all that apply. Company Type Total Revenues Total Assets Return on Equity Earnings per Share Average Yield Dividends per Share Average P/E Ratio AFLAC 6 7251 29454 17.1 2.08 0.9 0.22 11.5 Albertson's 4 14690 5219 21.4 2.08 1.6 0.63 19 Allstate 6 20106 80918 20.1 3.56 1 0.36 10.6 Amerada Hess 7 8340 7935 0.2 0.08 1.1 0.6 698.3 American General 6 3362 80620 7.1 2.19 3 1.4 21.2 American Stores 4 19139 8536 12.2 1.01 1.4 0.34 23.5 Amoco 7 36287 32489 16.7 2.76 3.1 1.4 16.1 Arco Chemical 2 3995 4116 6.2 1.14 6.1 2.8 40.4 Ashland 7 14319 7777 9.5 3.8 2.3 1.1 12.4 Atlantic Richfield 7…arrow_forwardSales of a particular product for the years 2017 through 2020 have been 45,332, 61,076, 59,547 and 77,795 respectively. If you are going to find the sales forecast by using simple regression (least squares) method, what would be the value of (a) for the regression equation?arrow_forward
- A researcher interested in explaining the level of foreign reserves for the country of Barbados estimated the following multiple regression model using yearly data spanning the period 2001 to 2016: ??=?+????+????+???? Where FR = yearly foreign reserves ($000’s), OIL = annual oil prices, EXP = yearly total exports ($000’s) and FDI = annual foreign direct investment ($000’s). The sample of data was processed using MINITAB and the following is an extract of the output obtained: Predictor Coef StDev t-ratio p-value Constant 5491.38 2508.81 2.1888 0.0491 OIL 85.39 18.46 4.626 0.0006 EXP -377.08 112.19 * 0.0057 FDI -396.99 160.66 -2.471 ** S = 2.45 R-sq = 96.3% R-sq(adj) = 95.3%…arrow_forwardA researcher interested in explaining the level of foreign reserves for the country of Barbados estimated the following multiple regression model using yearly data spanning the period 2001 to 2016: ??=?+????+????+???I Where FR = yearly foreign reserves ($000’s), OIL = annual oil prices, EXP = yearly total exports ($000’s) and FDI = annual foreign direct investment ($000’s). The sample of data was processed using MINITAB and the following is an extract of the output obtained: Predictor Coef StDev t-ratio p-value Constant 5491.38 2508.81 2.1888 0.0491 Oil 85.39 18.46 4.626 0.0006 EXP -377.08 112.19 * 0.0057 FDI -396.99 160.66 -2.471 ** S = 2.45 R – sq = 96.3% R – sq (adj) = 95.3% Analysis of Variance Source DF SS MS F P Regression 3 1991.31 663.77 ? ?? Error 12 77.4 6.45 Total 15 e) Perform the F Test making sure to state…arrow_forwardExplain the process of Computing the Regression Line?arrow_forward
- College AlgebraAlgebraISBN:9781305115545Author:James Stewart, Lothar Redlin, Saleem WatsonPublisher:Cengage LearningBig Ideas Math A Bridge To Success Algebra 1: Stu...AlgebraISBN:9781680331141Author:HOUGHTON MIFFLIN HARCOURTPublisher:Houghton Mifflin HarcourtLinear Algebra: A Modern IntroductionAlgebraISBN:9781285463247Author:David PoolePublisher:Cengage Learning
- Glencoe Algebra 1, Student Edition, 9780079039897...AlgebraISBN:9780079039897Author:CarterPublisher:McGraw HillElementary Linear Algebra (MindTap Course List)AlgebraISBN:9781305658004Author:Ron LarsonPublisher:Cengage Learning
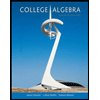
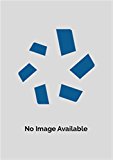
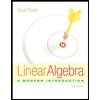
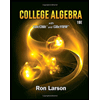
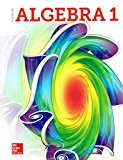
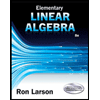